Chapter 11. What Is the Difference between Clinical and Statistical Significance?
Romina Brignardello-Petersen, D.D.S., M.Sc., Ph.D.; Alonso Carrasco-Labra, D.D.S., M.Sc., Ph.D.; Prakeshkumar Shah, M.Sc., M.B.B.S., M.D., D.C.H., M.R.C.P.; and Amir Azarpazhooh, D.D.S., M.Sc., Ph.D.
In This Chapter:
Issues Pertaining to Statistical Significance
• Minimal Important Difference (MID)
• Patient-Reported Outcome Measures (PROMs)
Introduction
Investigators in a study published in 2010 compared the efficacy of nimesulide with that of meloxicam (two nonsteroidal anti-inflammatory drugs) in the control of postoperative pain, swelling, and trismus after extraction of impacted mandibular third molars.1 Among their conclusions, the authors stated that “[nimesulide] was more effective than [meloxicam] in the control of swelling and trismus following the removal of impacted lower third molars.”1 This conclusion was supported by the results observed in their randomized clinical trial. The authors reported that after the third molar surgical extraction, patients experienced a reduction in mouth opening, but that this reduction was significantly larger at 72 hours after surgery when patients had received meloxicam than when patients had received nimesulide. The authors reported a P value of 0.03 for the difference in the mean reduced mouth opening of 1.39 centimeters in the nimesulide group versus 1.7 cm in the meloxicam group. This difference of 3.1 millimeters was the basis for the authors’ claim of the superiority of nimesulide. However, from a clinical perspective, this difference does not seem large. How can we know if these numbers show that the reduction in mouth opening is significantly larger when patients received meloxicam therapy, as the authors report? What do the authors mean when they use the expression “significantly larger”? Is a P value < 0.05 sufficient to claim that there is a significant difference?
In this chapter, we aim to clarify and differentiate the concepts of statistical significance and clinical significance, as well as provide guidance on how to interpret research results to determine whether an observed difference is clinically meaningful.
Statistical Significance
It is not feasible to conduct a study in which investigators study all potential patients. Thus, researchers have to base their conclusions on a sample of people and then determine the probability that a conclusion made on the basis of an analysis of data from this sample will hold true when applied to the population as a whole.2
Researchers have used statistical significance for many years as a means to assess the effects of interventions in clinical research and to show that observed differences likely are not due to chance.3 Usually, the claim of statistical significance depends on obtaining a specific P value after conducting a statistical significance test, as in the earlier example.
A P value is the probability of obtaining a mean difference that is at least as far from a specified value (null value) as the mean observed in the study, given that this specified value is the true value.4 In the example above, if we assume that the true difference in mouth-opening reduction between nimesulide and meloxicam is 0 mm, what the authors found was a 3% probability of observing the 3.1-mm difference (or larger) that they detected. Because the probability of that happening is so small, it is unlikely that the differences they observed were due to chance; thus, they could claim that there are real and statistically significant differences between the two treatments.
As stated earlier, the P value is obtained when conducting statistical hypothesis testing. To perform this test, we start by assuming that the result of interest (the mean or proportion of the outcome of the study) is equal to some specific value. This claim is called the null hypothesis. In the example, the null hypothesis was that there is no difference in mouth-opening reduction between the two drug groups. The investigators then construct an alternative hypothesis such that it contradicts the null hypothesis. In this case, the alternative hypothesis was that differences existed between the drugs with regard to mouth-opening reduction.5 The next step is to compare the data obtained in the study with the value specified in the null hypothesis—using the probability theory—to attain a P value. The P value is related to how much the data contradict the null hypothesis. If a large P value is obtained, the data are consistent with the null hypothesis. Conversely, if a small P value is obtained, the data contradict the null hypothesis, and the results are unlikely to have occurred if the null hypothesis actually were true. However, the investigators must decide whether the P value is sufficiently small to reject the null hypothesis. Although it is arbitrary, a P value of 0.05 has been the conventionally accepted value for level of significance.6
Type I Error
The level of significance reflects the probability of committing a type I error—that is, rejecting the null hypothesis when it actually is true.7 In other words, it is the probability of falsely claiming that there is a difference in mouth-opening reduction when there is not. According to the earlier description, the P value is not the probability that the null hypothesis is true. This is a common misconception. A large P value does not mean that the null hypothesis is true; at best, it implies that the study results are inconclusive. Likewise, a small P value does not mean that the alternative hypothesis is true; at best, it implies that the data are incompatible with the null hypothesis being true.5
Type II Error
On the other hand, a probability exists of not rejecting the null hypothesis when it is false, which is known as a type II error. A type II error occurs when researchers fail to observe a difference between interventions even though a true difference does exist.8 For example, imagine a study in which the researcher wants to determine whether the incidence of cleft lip and palate is larger in one of two towns. Let us assume that a difference between the towns truly exists, and that the true incidence in town A is five in 1,000 newborns, whereas in town B, it is one in 1,000 newborns. If the researcher observes only 50 newborns in each town, it is likely that he or she will not find an infant with cleft lip and palate in either town. At the end of the study, the data will suggest that the incidence of this malformation is 0 for both towns. Therefore the researcher will claim falsely that no difference exists between the two towns with regard to the incidence of cleft lip and palate, because he or she failed to find any infant with the malformation. This is an issue of “power” of the study.
Study Power
The power of a study is its capacity to detect differences that truly exist, and it is defined as the probability of rejecting the null hypothesis when it is false.5 Power is the opposite of a type II error; higher power implies a smaller probability of committing a type II error, and vice versa. Thus, our hypothetical study of the incidence of cleft lip and palate in two towns was underpowered. Also, as this example illustrates, the power of a study depends, in part, on the sample size (the number of newborns observed) and the effect size (the difference in the incidence of the malformation between the groups).
Issues Pertaining to Statistical Significance
Understanding statistical significance requires thinking in terms of probability. At the completion of a statistical significance test, there are two possible outcomes: reject the null hypothesis or fail to reject the null hypothesis. This qualitative result often is used as a substitute for quantitative scientific evidence.7 As illustrated in Tables 11.1 and 11.2, one issue of concern is that the results of statistical testing are influenced highly by the sample size and variability within the sample. Table 11.1 shows that increasing the sample size, while keeping everything else constant, results in a smaller P value’s having been obtained in hypothesis testing. This leads to statistically significant results when the sample size is larger and to nonstatistically significant results when the sample size is smaller. Table 11.2 shows that, while keeping everything else constant, a smaller variation in the response to an intervention among participants in one group results in a smaller P value’s having been obtained in statistical testing. Consequently, statistically significant results are obtained when the variability is smaller, and nonstatistically significant results are obtained when the variability is larger.
Therefore, studies in which the sample size is large, in which there is little variability within the sample, or both, are more likely to lead to statistically significant results compared with identical studies in which the sample sizes are smaller and the variability is greater. This is true even when the effect size (the difference between the groups) is the same, as shown in Tables 11.1 and 11.2.
Table 11.1. Relationship Between Sample Size and P Values (Assuming Constant Means and SDs*)†
* SDs = standard deviations.
† This table illustrates the influence that sample size has on P values. When the mean of the outcome and its SD are constant, an increase in sample size leads to smaller P values.
‡ Two-sided unpaired t test; significance level ≤ 0.05. P values were calculated by using statistical software (The R Project for Statistical Computing, The R Foundation for Statistical Computing, Vienna).
Table 11.2. Relationship Between Variability Estimates and P Values (Assuming Constant Means and Sample Size)*
* This table illustrates the influence that sample variability has on P values. When the mean outcome value and sample size are constant, an increase in sample variability leads to higher P values.
† SD = standard deviation.
‡ Two-sided unpaired t test; significance level ≤ 0.05. P values were calculated by using statistical software (The R Project for Statistical Computing, The R Foundation for Statistical Computing, Vienna).
In the study in which De Menezes and Cury1 compared the efficacy of nimesulide versus that of meloxicam for the control of postoperative pain, swelling, and trismus after extraction of impacted mandibular third molars,1 let us imagine that the authors had measured the outcome pain as dichotomous (that is, the presence or absence of pain). In addition, let us suppose that after completing the trial, these authors observed that 85% of participants who received nimesulide therapy reported experiencing no postoperative pain, whereas 90% of participants allocated to the meloxicam group reported experiencing no postoperative pain. If we compare these two proportions in a trial in which researchers enrolled a total of 80 patients, the P value for the hypothesis testing would be.25. On the other hand, had researchers in the trial enrolled 800 participants, the P value for the same comparison would be.016. Although the difference in the proportion of patients with no postoperative pain in both trials was 5%, the conclusion drawn in the trial with the smaller sample size is that there was no statistically significant difference between the two drugs, whereas in the trial with the larger sample size, the authors could have made the opposite claim.
In light of the above, the main problem is that hypothesis testing can omit any differences that were observed in the study. Statistical testing indicates only the probability of the observed differences—without regard to the size of the differences—occurring by chance.2
By using the hypothesis testing approach and claiming that there are differences in effects of interventions on the basis of a P value alone, we lose valuable information regarding the size of the effect.9 This is illustrated in Table 11.3, which shows different effect sizes leading to the same conclusion: the results are or are not statistically significant.
Table 11.3. Relationship Between Effect Size Increase and Statistical Significance (Assuming Constant Mean in Meloxicam Group and Constant Standard Deviations in Both Groups)*
* This table illustrates that when statistical hypothesis testing is used, the conclusion drawn (that is, whether differences are or are not statistically significant) does not reflect how much larger the effect of one intervention is compared with another. Even though the mean difference in outcomes across the scenarios is increasing, the conclusion derived from statistical hypothesis testing is exactly the same.
† SD = standard deviation.
‡ Two-sided unpaired t test; significance level ≤ 0.05. P values were calculated by using statistical software (The R Project for Statistical Computing, The R Foundation for Statistical Computing, Vienna).
Researchers can reach the same P value in many ways by combining different treatment effects, within-group variability, and sample sizes. Thus, the results of a statistical test cannot indicate whether a treatment effect is important enough to be useful for patients.10 Moreover, some readers may erroneously interpret small P values as large effects,11 when in fact, the P values actually represent only the probability of having observed what was observed in the study and indicate nothing about the effect size.
Clinical Significance
In 1984, Jacobson and colleagues12 proposed the term “clinical significance” as a means of evaluating the practical value of a treatment. Although the literature contains many definitions of and discussions about this term,9,13–18 most authors agree that a clinically significant result must fulfill the following criteria:
• A change in an outcome or a difference in outcome between groups occurs that is of interest to someone; patients, physicians, or other parties interested in patient care conclude that the effect of one treatment compared with another makes a difference.
• The change or difference between groups must occur in an important outcome. It can be any outcome that may alter a clinician’s decisions regarding treatment of a patient, such as a reduction in symptoms, improvement in quality of life, treatment effect duration, adverse effects, cost-effectiveness, or implementation.
• The change or difference must be statistically significant. The difference must be greater than what may be explained by a chance occurrence.9,13–18
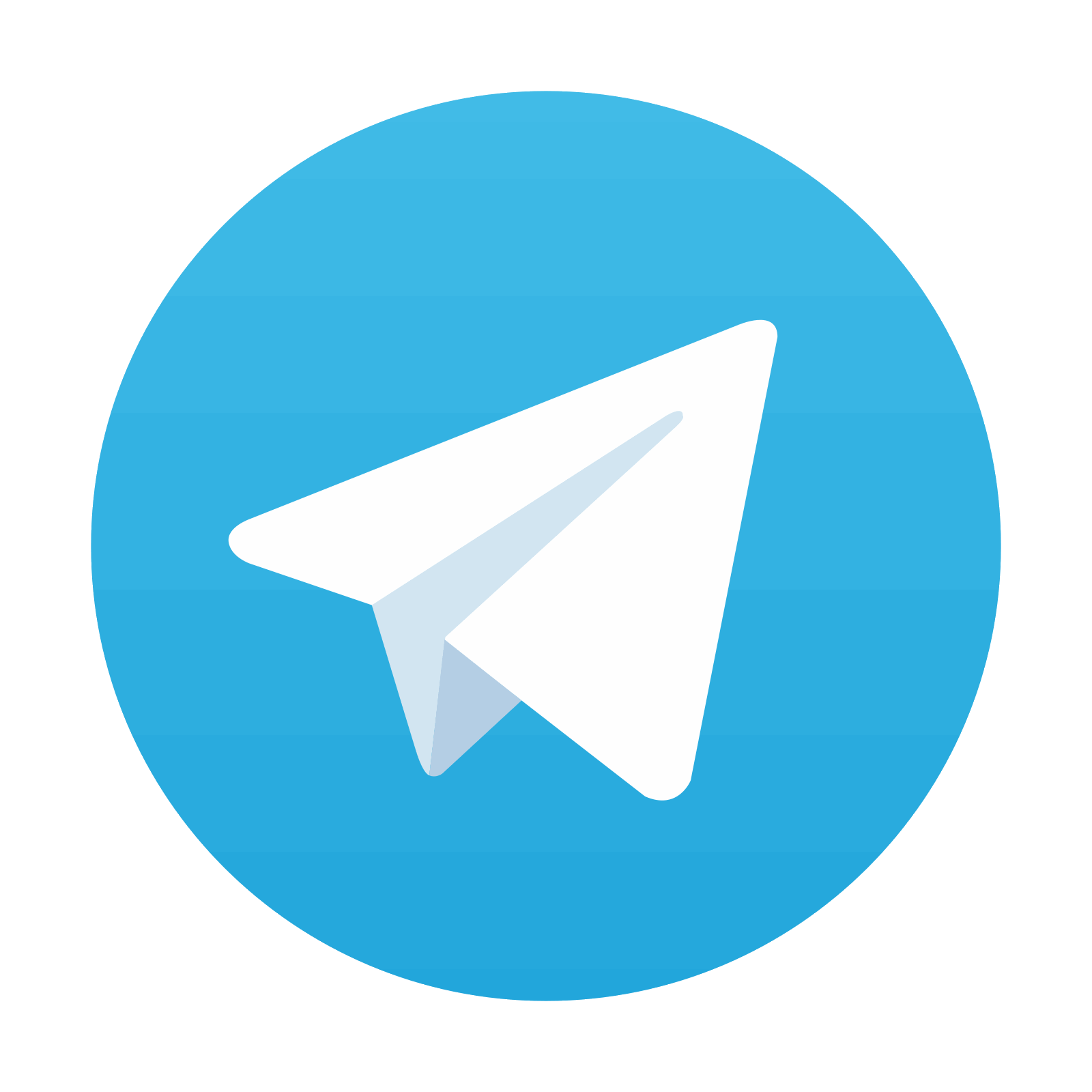
Stay updated, free dental videos. Join our Telegram channel

VIDEdental - Online dental courses
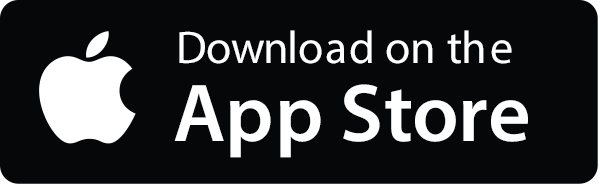
