8
Brain Mechanisms of Adaptation of Oral Sensorimotor Functions
8.1 Brain Plasticity and Adaptation
8.1.1 Introduction
Plastic may be one of the most important inventions in the modern world because it is so malleable that it can be moulded into almost any form for any function. Likewise, ‘neuroplasticity’ may be one of the most important concepts in brain science. While plastic materials can be moulded into any wanted shape and size, the human brain can be shaped (and ‘re‐shaped’) at the functional and structural levels so that individuals can adapt to the changing environment. The reason why neuroplasticity has drawn much attention in neuroscience is that it provides a biological basis for behavioural adaptation, i.e. the ability that people can modify their behaviour to meet environmental changes. In terms of brain plasticity, behavioural changes are associated with changes in brain structure and function. In this chapter, we discuss the mechanisms of neuroplasticity and briefly summarize the advantage of neuroimaging in studying brain plasticity.
8.1.2 Neuroplasticity: Clarification of Misconception
The term ‘neuroplasticity’ has been widely used for many years in the neuroscientific literature. It generally refers to the flexibility of the brain ‘to modify, change and adapt both structure and function throughout life and in response to experience’ (Voss et al. 2017). Some terms relevant to plasticity, such as ‘rewiring of the brain’, ‘cortical reorganization’ and ‘compensation’, have frequently emerged in scientific publications and mass media. In the following sections, we first clarify the definitions and misconceptions of the terms.
8.1.2.1 Behavioural and Brain Plasticity
At the level of human behaviour, plasticity is associated with ‘the susceptibility of human behaviour to modification’ (Pascual‐Leone et al. 2005). At the level of the central nervous system (CNS), brain plasticity refers to the capacity for the nervous system to be reorganized functionally and structurally (Gazzaniga et al. 2019), and notably, such a reorganization can be moulded by environmental changes and experiences (Pascual‐Leone et al. 2005; Voss et al. 2017) (Figure 8.1a). Human behaviour is far complicated than a fixed set of responses triggered by environmental stimuli. A common belief about the neural system is that stimuli from the environment are translated by the brain into a corresponding behaviour. Such a stimulus–response association is pre‐programmed and fixed (Figure 8.1a). However, our behaviour can be modified by long‐term experiences, such as the case of chronic pain (see Chapter 6). In patients with chronic pain, their behaviour towards stimuli may vary depending on their past experience of pain. In other words, our behaviour is modifiable rather than pre‐programmed, and the neural system is flexible to be tuned according to environmental changes rather than hard wired. For example, long‐term experience of chronic pain may sculpt the brain at the structural and functional levels, leading to different behavioural responses to environmental stimuli (Figure 8.1a).

Figure 8.1 The concept of neuroplasticity and functional adaptation. (a) With a ‘pre‐programmed’ nervous system, our behaviours responding to environmental stimuli are determined by a fixed set of stimulus–response links. For example, a great danger will elicit a stronger emotional response compared to a mild danger (the upper panel). However, our nervous system is modifiable and can be tuned according to environmental changes. Long‐term experience may sculpt the brain at the structural and functional levels, leading to different behaviours responding to environmental stimuli. For example, past experience may predispose the brain to be more sensitive to danger and make a stronger emotional response (the lower panel). (b) Functional adaptation is associated with the improvement of one’s functional performance under environmental challenges. For example, individuals learn to run faster (i.e. increased performance) when they are threatened by a greater danger (the upper panel). Compensation, in contrast, highlights the restoration of performance from a worse status back to a normal status. For example, individuals with a disability can run as quickly as normal individuals when facing danger by compensating their mobility with the help from tools and rehabilitative therapy (the lower panel).
8.1.2.2 Misconceptions About Brain Plasticity
Brain plasticity is often linked to the concept of functional adaptation, which is associated with the improvement of one’s functional performance under environmental challenges (Frisancho 1993) (Figure 8.1b). There are several misconceptions regarding plasticity. Firstly, brain plasticity is often linked to the recovery of functions in patients with brain disorders (e.g. stroke or trauma). Therefore, brain plasticity is sometimes conceived as an improvement responding to the malfunction caused by pathological conditions. This is not entirely correct because plasticity is ‘an intrinsic property of the nervous system retained throughout a lifespan’ (Pascual‐Leone et al. 2005). The brain is continuously being moulded as long as it interacts with the environment, as a normal ongoing state (Pascual‐Leone et al. 2005). Therefore, it is not a phenomenon specific to a disease. Secondly, plasticity is associated with behavioural modification, which can be acquired by learning (e.g. to develop a skill of using a high‐speed dental handpiece). Therefore, plasticity is often considered for the gain of new abilities. However, the behavioural change may not necessarily be an increase in capability. It may be loss of an already gained function, the release of a previously suppressed function or expressing a similar function by different neural substrates (Pascual‐Leone et al. 2005). Moreover, even if the behavioural change is a positive one (e.g. acquiring expertise in dancing), such a change does not necessarily correspond to a ‘positive’ change of the brain (e.g. an increased amount of a brain feature). For example, a previous study of structural brain features found that ballet dancers, compared to nondancers, showed a lower (but not higher) grey matter volume (GMV) in multiple regions of sensorimotor control and learning (Hänggi et al. 2010). One should be careful when making inferences between the modification at behavioural and brain aspects, which can be far complicated at the cellular level (Zatorre et al. 2012).
8.1.2.3 Recovery, Reorganization and Compensation
From the neurological perspective, the term ‘recovery’ mostly applies to conditions when patients suffer brain damage. For example, post‐traumatic patients may initially lose some functions corresponding to the regions of brain damage. Recovery is identified when patients regain their performance to the pre‐traumatic level. Such a recovery is more commonly seen for the functions of cognitive domains (Mogensen 2011). Functional recovery is associated with neural reorganization, which is usually promoted by training programmes for rehabilitative purposes. Notably, even without explicit training, patients are continuously challenged by daily life activities as an ‘informal’ training and reorganization may still undergo (Mogensen 2011). Therefore, from the behavioural perspective, the term ‘reorganization’ is often associated with brain plasticity, especially in the literature on the acquisition of new skills and expertise (Chang 2014). The term ‘compensation’ may refer to a process associated with the damage of the CNS and deficits in functions. The compensating brain should ‘assist in maintaining a normal level of behaviour’ (Gregory et al. 2017). Therefore, a prerequisite condition for functional compensation is that one’s performance returns to a normal level. From the perspective of ageing neuroscience, the term also refers to the situation ‘in which age‐related increases in brain activity are directly correlated with better performance in older adults’ (Cabeza et al. 2018). Both the perspectives from neurology and ageing neuroscience consistently highlight that compensation relates to the change of performance from a sub‐normal level (due to diseases, trauma or ageing) to a normal level (Figure 8.1b).
8.1.3 Neural Mechanisms of Plasticity
The concept of neuroplasticity has evoked great interest in neuroscience. However, the mechanisms underlying neuroplasticity are complicated because of the complexity of the nervous system. The nervous system is organized with a hierarchical architecture, from the cellular level (e.g. a neuron) to the brain level (e.g. the whole‐brain connectome). Therefore, researchers have used different approaches to investigate neuroplasticity, from the changes of synaptic activity to the changes of functional/structural brain connectome. Many neuroimaging research focuses on neuroplasticity at the whole‐brain scale. The major aim of these studies is to identify a structural or functional change corresponding to the effect of long‐term experience, which can be the acquisition of a new skill or the suffering from chronic pain. Because the nervous system is organized in a hierarchical architecture, the alterations at the whole‐brain scale reflect the changes in the synapse, which is the major ‘site’ where plasticity occurs. Synaptic plasticity can be altered in several ways, including changing synaptic structures, such as the dendritic spines and filopodia, adding new synapses and removing pre‐existed synapses (Fu and Zuo 2011). For example, during motor training, elimination of the pre‐existed spines and generation of new spines were identified in mice (Xu et al. 2009). When adult animals received lesions and sensory manipulations, the dynamics of axonal arborization were modified (Fu and Zuo 2011). At the synaptic level, both de novo growth and removal of dendritic spines are associated with neuroplasticity (Fu and Zuo 2011).
In addition to the structural changes at the synaptic level, neuroplasticity can be investigated by assessing the functional changes of synaptic activity. Traditionally, the association between structural and functional changes is interpreted under the framework of Hebbian plasticity (Hebb 1949), which is generally summarized as ‘units that fire together, wire together’ (Munakata and Pfaffly 2004). In other words, structural changes (i.e. how neurons are wired) are associated with the neural activity (i.e. firing a neuronal signal) related to functional changes. The activity‐dependent alteration of synaptic plasticity includes the long‐lasting strengthening (i.e. long‐term potentiation, LTP) and the long‐lasting reduction of the synaptic strength (i.e. long‐term depression, LTD). In terms of Hebbian plasticity, the strength of synaptic plasticity is associated with the correlation between presynaptic and postsynaptic activities. Animal research has revealed that LTP occurs when presynaptic and postsynaptic activities are correlated, and the activation of the postsynaptic N‐methyl‐D‐aspartate receptor (NMDAR) plays a key role in the plasticity (Smith et al. 2009). In general, the structural and functional changes are closely associated at the synaptic level, and both are fundamental for the neural mechanisms of plasticity.
8.1.4 Neuroimaging as a Tool for Studying Brain Plasticity
The advent of neuroimaging has offered a great opportunity for researchers to investigate neuroplasticity beyond the synaptic level. Neuroimaging contributes to our understanding of brain plasticity by associating the structural and functional changes of neuroimaging findings with changes in individual experience or learning process (Zatorre et al. 2012). In the following section, we present several notable examples of neuroimaging research on brain plasticity, focusing on the structural and functional features identified via magnetic resonance imaging (MRI).
8.1.4.1 Plasticity Identified by Structural Brain Features
In a pioneering study, Maguire et al. (2000) compared GMV of licensed London taxi drivers, who were experts in spatial navigation, with control subjects (non‐taxi drivers). They found that an increased GMV in the posterior hippocampus of the taxi‐driver group vs. controls and, importantly, the posterior hippocampal volume was positively correlated with the duration they serve as taxi drivers (Maguire et al. 2000) (Figure 8.2a). The study showed that the individual variability in structural features may be associated with the effect of the plasticity of experience/learning. In another landmark study, Draganski et al. (2004) identified the structural alteration related to a training course of juggling. Using a longitudinal design, they identified changes in regional GMV before vs. after the juggling exercise. Moreover, the regional GMV change was associated with the performance of a juggling task (Draganski et al. 2004). Subsequently, Scholz et al. (2009) investigated the plasticity of white matter associated with juggling training using diffusion tensor imaging (DTI). They found an increased fractional anisotropy (FA) in the intraparietal sulcus during the post‐training scan compared to the pre‐training scan. Furthermore, the increased FA sustained for four weeks after the subjects ceased to practice juggling, suggesting a long‐lasting effect (Scholz et al. 2009) (Figure 8.2b). Together, the findings reveal that alterations of grey and white matter may reflect the plasticity related to sensorimotor training in human subjects.

Figure 8.2 Experimental design of neuroimaging research on brain plasticity. (a) A cross‐sectional study reveals a significant difference in structural brain features between subjects with and without a professional skill (e.g. driving). Individual variations in brain features further relate to the duration of skill training (the left panel). Results from the cross‐sectional design only suggest, but not confirm, the causal direction of a plastic effect. The difference in brain features (as observed by neuroimaging) may be attributed to prior experience of training (the middle panel). However, it is also possible that the individual differences in the brain features predispose their performance of a skill (the right panel). (b) To better elucidate the plastic effect of training, a longitudinal design is used to assess the performance and brain features at different stages of training. Importantly, one can assess whether the plastic effect, i.e. changes in brain features during the training session, can last for a period or vanish right after the termination of training. (c) The same longitudinal design also helps to elucidate individual differences in their performance. For example, the variation in brain features may account for the difference in learning speed.
8.1.4.2 Plasticity Identified by Functional Brain Features
In addition to the structural features, functional magnetic resonance imaging (fMRI) has been widely used to assess the functional features associated with learning. For example, Steele and Penhune (2010) investigated the brain activation associated with motor sequence learning in younger adults. They identified brain activation at the regions related to motor learning (including the premotor cortex [PMC], the supplementary motor area [SMA], the basal ganglia and the cerebellum) during the early stage of learning. Notably, as the performance improved from Day 2 to Day 5, the activation of the motor‐related regions (including the PMC and the cerebellum) decreased. Across individual subjects, a greater performance was associated with lower activation of these regions (Steele and Penhune 2010). Lewis et al. (2009) investigated the changes in the intrinsic functional network before and after subjects received training on a shape discrimination task. They found an activation at the visual cortex associated with the modulation of visual stimuli. In addition, they identified more negative connectivity between the dorsal attention network and the modulated area of the visual cortex after training (vs. before training). In contrast, less negative connectivity between the default mode network (DMN) and the unmodulated area of the visual was identified after training (vs. before training) (Lewis et al. 2009). The findings suggest that brain plasticity may be associated with changes in the functional connectivity between brain regions, in addition to the activation of a single region. In another longitudinal study with a longer training period, Herholz et al. (2016) investigated the changes in brain activation after six‐week piano training and the association between the activation and the learning rate of subjects (Figure 8.2c). They found that the activation of the PMC and the cerebellum was associated with the ‘learning effect’ (i.e. after > before piano training). Moreover, the brain activity before training (including the primary auditory cortex, the hippocampus and the caudate nucleus) was predictive to the learning rate that subjects demonstrated during training (Herholz et al. 2016). In general, the findings suggest that functional features, including task‐related activation and the pattern of intrinsic functional features, can be sculpted by motor or perceptual learning.
8.1.5 Limitations of Neuroimaging Research on Neuroplasticity
The above‐mentioned studies have demonstrated neuroimaging as a powerful tool to investigate the brain plasticity associated with learning. However, there remain several critical questions unanswered. In the following sections, we focus on the association between neuroimaging features and synaptic mechanisms and the association between plasticity induced by training and experience.
8.1.5.1 Association Between Neuroimaging Features and Synaptic Mechanisms
Synaptic plasticity plays a key role in neuroplasticity. However, the association between the plasticity at the synaptic level and the plasticity at the brain level has not yet been clarified. For example, even though fMRI revealed changes in regional activation associated with the performance of learning (e.g. Steele and Penhune 2010), the association between the functional features of neuroimaging and the underlying synaptic changes has remained unclear (Poldrack 2000). Using DTI, researchers have identified the alterations of white matter in rats trained with a spatial navigation task for five days. Furthermore, they found that at the brain level, the alterations observed via DTI were associated with an increase in glial fibrillary acidic protein (GFAP) and synaptophysin, suggesting an effect of synaptic plasticity at the cellular level (Blumenfeld‐Katzir et al. 2011). Another study has identified that in human subjects, learning‐induced plasticity on the white matter can be identified just after two hours of training (Sagi et al. 2012). In this study, DTI was performed before and after subjects played a computer game for two hours. They identified a decrease in mean diffusivity in the hippocampus and the parahippocampus, the regions associated with spatial navigation. Moreover, they had the animal subjects perform a water maze task and found the same plasticity effect on the hippocampus, with an increased GFAP and brain‐derived neurotrophic factor (BDNF), a factor for neuronal growth. In general, the findings from combined animal and neuroimaging research suggest that brain plasticity identified using neuroimaging methods may be associated with the cellular mechanisms of synaptic plasticity.
8.1.5.2 Association Between Plasticity Induced by Training and Experience
While neuroimaging studies focus on the neuroplasticity of learning, such ‘learning’ is mostly induced by an explicit and well‐defined training protocol (e.g. piano training [Herholz et al. 2016] and juggling [Scholz et al. 2009]). In contrast, much of our experience was shaped implicitly by long‐term interaction with the environment. For example, the brain plasticity of chronic pain (see Chapter 6) and chronic stress has been widely reported (Radley et al. 2015). In most of these studies, changes in brain features are associated with individual differences of the cognitive–affective aspects (e.g. pain intensity, perceived stress and disability) rather than the performance quantified by a standardized task. However, one should keep in mind that the long‐term experience of pain or stress is modulated by the interaction with environmental stimuli, which are presented as a ‘background’ stimulus. For example, chronic pain usually relates to avoidance behaviour. Therefore, how individuals actively adapt to the environment plays a key role in the shaping of one’s long‐term experience. It is also noteworthy that during training individual performance is associated with their past experience. In other words, there exists a great overlap between the plasticity shaped by long‐term experience and a specific training protocol. It requires further investigation of how these factors interactively form the basis of neuroplasticity.
8.1.6 Summary
- Brain plasticity refers to the capacity for the nervous system to be reorganized functionally and structurally. Such a reorganization can be moulded by environmental changes and experiences.
- Neuroplasticity may be represented at a different scale of the neural system. At the whole‐brain level, experience and training may mould the large‐scale functional and structural networks of the brain. At the synaptic level, both de novo growth and removal of dendritic spines are associated with neuroplasticity.
- Alterations of grey and white matter, as neuroimaging features, may reflect the plasticity related to sensorimotor training in human subjects.
- Functional brain features, including task‐related activation and the pattern of intrinsic functional features, can be sculpted by motor and perceptual learning.
8.2 Adaptation of Pain and Oral Sensory Functions
8.2.1 Introduction
In the preceding chapters, we have discussed the mechanisms of the human oral motor (Chapter 4) and sensory functions (Chapters 5 and 6), with the main focus on the interaction between the brain and the stomatognathic system. In general, we interpreted the findings based on the oral–brain‐behaviour (OBB) framework (see Chapter 1), highlighting the exchange of sensory and motor information between the brain and the oral apparatus. However, the brain–stomatognathic association is not a static one. According to the brain–stomatognathic axis (BSA) framework, both the brain and the stomatognathic system respond to various environmental and bodily changes, which include the challenge of different foods, deficits of oral structure (e.g. tooth loss) and ageing. In addition to the essential sensorimotor functions, the ability to maintain feeding behaviour by adapting to the changing environmental and bodily conditions should be considered as one of the primary functions of the stomatognathic system. In this chapter, we look into how individuals adapt to oral sensory stimuli. The role of the brain in such an adaptive process is highlighted.
8.2.2 The Manifolds of Adaptation
Before the following discussion, first, we may need to define the concepts of adaptation, which plays a key role in the understanding of human behaviour. In a very broad sense, adaptation can be defined as ‘a process whereby the organism has attained a beneficial adjustment to the environment’ (Frisancho 1993). To highlight the brain–stomatognathic association in adaptation, we clarify the following concepts: sensory adaptation (for oral sensory stimuli and pain), behavioural adaptation (for maintaining feeding behaviour) and the difference between functional and structural adaptation.
8.2.2.1 Sensory Adaptation
Sensory adaptation generally refers to ‘a gradual loss of sensation during prolonged stimulation’ (Theunissen et al. 2000). Experimentally, it can be observed as ‘the diminution of perceived sensation with constant stimulus intensity’ (Price 1999). This definition, though not clearly defining the temporal and spatial features of the stimuli, points out a key element of adaption: the nervous system becomes gradually less responsive to environmental changes. One of the benefits of sensory adaptation is to encode environmental stimuli with higher efficiency. Because the information‐processing capacity of the nervous system is limited, it would be difficult for the nervous system to encode all information from the environment, which has a great variability (Wark et al. 2007). Instead, our nervous system would ‘adapt’ (i.e. becoming less responsive) to environmental stimuli that are more common to receive. In the meantime, the mechanism enables the system to be more sensitive to a rare stimulus (e.g. a suddenly increased brightness of light) (Wark et al. 2007). In other words, sensory adaptation does not mean a ‘sluggish’ status of our perception but a status for individuals to get ready for the changing environmental conditions.
8.2.2.2 Behavioural Adaptation
The term ‘behavioural adaptation’ has been widely used in research on animal behaviours, which focus on the association between the choices and consequences of behaviours (e.g. foraging, mating or parental behaviour) and ever‐changing environmental conditions (Clark 1991). In the following section, we limit the use of behavioural adaptation to the change of individual behaviours to cope with environmental and bodily changes. Unlike sensory adaptation, which is unintentional neural processing of stimuli, behavioural adaptation is associated with one’s intention to improve functions by explicitly choosing among different strategies.
8.2.2.3 Structural vs. Functional Adaptation
The key element for functional adaptation is that the changes aim at improving one’s functional performance under environmental challenges (Frisancho 1993). Notably, functional adaptation can be associated with structural adaptation or even the adaptive process at a more fundamental level, e.g. genetic adaptation. Therefore, functional adaptation is associated with the adaptation from structural to behavioural aspects (Frisancho 1993). In contrast to functional adaptation, the term structural adaptation is discussed by a stricter definition. In the following sections, we tend to refer to the structural adaptation to the condition when both changes in structural features and corresponding changes in functional performance are identified.
8.2.3 Adaptation of Pain
Sensory adaptation is commonly seen for visual and auditory stimuli. However, sensory adaptation to noxious stimuli, e.g. heat stimuli, may not be a pronounced phenomenon. A key difference between pain and other sensory modalities is that pain experience cannot be interpreted solely by the intensity of nociceptive input (see Chapter 6), and it may vary between individuals and conditions due to a variety of cognitive–affective factors (e.g. the perception of threat). Therefore, when it comes to the adaptation of pain, the effect of cognitive–affective processing should not be ignored.
8.2.3.1 Sensory Adaptation of Pain
Experimentally, the adaptation of pain can be identified if subjects perceive diminution of sensation when receiving constant stimulus intensity (Price 1999). Coghill et al. (1993) found that when subjects received prolonged heat stimuli to evoke pain for 20 minutes, they reported a decrease in intensity and unpleasantness (that indicated adaptation), but only within the first two minutes of stimulation. The perceived intensity and unpleasantness remained stable from 2 to 20 minutes (Coghill et al. 1993). The findings suggest that pain intensity did not decrease as a linear function of time. Using a different paradigm, Bingel et al. (2007) delivered painful stimuli for 20 minutes to healthy subjects consecutively for eight days. They also found a gradual decline in pain ratings in an exponential but not a linear pattern (Bingel et al. 2007). The same pattern of an exponential decline of pain was observed by following research (Riedl et al. 2011). Notably, because pain is often perceived as a threat related to tissue damage (IASP 2020), the observed increase or decrease in perceived pain cannot be fully attributed to sensory adaptation per se. Other factors, such as changes in cognitive and emotional experiences of pain, should not be ignored when one interprets the results (Price 1999).
8.2.3.2 Pain Adaptation at the Cognitive–Affective Level
Pain can be modulated by a variety of cognitive–affective factors, including the individual ability to cognitively appraise (or reappraise) the threat related to pain. In psychology, the term ‘appraisal’ refers to ‘the evaluation of the meaning of emotional stimuli’ (Kalisch et al. 2006) and is considered as a major factor contributing to the generation of emotional responses. Therefore, it can be conceived as a process that an individual actively engages with an emotional stimulus by interpreting and evaluating its meaning. For example, when patients notice that a dentist brings forwards a ‘forceps‐like’ instrument, they may feel anxious about the sharp edge of the forceps, which just look threatening. However, upon realizing that it is a common dental instrument, patients may feel more relaxed. The example shows that the way how we think about a stimulus affects our emotional experience. Individual differences in emotional responses to the same stimuli may be attributed to their different ways of evaluating the stimuli, i.e. individual variability in the ability of cognitive appraisal.
8.2.3.3 Maladaptive Behaviour of Pain
Via cognitive appraisal, individuals may form a positive experience of pain and adapt to it. In contrast, some factors may have a negative effect on modulating pain. Cumulating evidence has suggested that an increased fear/anxiety of pain and pain catastrophizing may relate to maladaptive behaviour, such as avoidance behaviour (Vlaeyen et al. 2016). An increased fear/anxiety of pain is associated with the stronger intensity of pain that one perceives (Lin et al. 2013; Ploghaus et al. 2001) and biases our perceptual decision whether the stimulus is painful or not (Wiech et al. 2010). For example, increased uncertainty about receiving painful stimuli and an increased threat value of stimuli increased fear and anxiety of pain (Lin et al. 2013; Ploghaus et al. 2001; Wiech et al. 2010). Another critical factor is pain catastrophizing. There are three major constructs of pain catastrophizing: rumination, magnification and helplessness (Sullivan et al. 1995). An increased degree of rumination and magnification is associated with the tendency to attend to pain and exacerbate it. An increased degree of helplessness is associated with the inability to effectively cope with pain (Lin 2013). It should be noted that a stronger tendency of pain catastrophizing does not just mean greater fear or anxiety. Stronger catastrophizing is associated with an increased tendency to form catastrophic thoughts. The thoughts (e.g. ‘pain may get worse and worse’) contribute to the development of maladaptive behaviour, such as self‐limiting daily activities to avoid pain (Vlaeyen et al. 2016).
8.2.4 Neuroimaging Research on Pain Adaptation
While there have been plenty of studies on brain activation when individuals feel pain (see Chapter 6), fewer studies focused on the brain mechanisms of pain adaptation. In the following sections, we focus on the brain activation of the pain‐adaptation paradigm, which demonstrates the decline of pain ratings after days of pain stimulation. The brain mechanisms of adaptation at the cognitive–affective level, mainly from cognitive appraisal, are also discussed.
8.2.4.1 Sensory Adaptation of Pain
In an earlier study, Bingel et al. (2007) investigated the change of brain activation towards heat pain stimuli delivered on eight consecutive days in healthy adults. Behaviourally, they found a nonlinear decline of pain intensity during this period, similar to the findings from Coghill et al. (1993). fMRI was performed on Day 1, Day 8 and two weeks after the end of pain stimulation (i.e. Day 22). They found a decline of brain activation of the pain‐related regions, including the thalamus, the anterior insula, the secondary somatosensory cortex (S2) and the putamen, corresponding to the decline of perceived pain. Notably, comparing the brain activation of Day 8 with Day 1, they found an increase in brain activation in the sub‐genual anterior cingulate cortex (ACC), which may suggest a role of anti‐nociceptive control of pain (Bingel et al. 2007). Subsequently, Riedl et al. (2011) investigated the intrinsic connectivity network (ICN) of 13 healthy adults who received heat pain stimulation for 11 consecutive days. In order to explore the ICN, the subjects received resting‐state fMRI before and after pain stimulation on Day 1 and Day 11. Pain adaptation was identified by a nonlinear decline of pain ratings (Riedl et al. 2011). They found that in Day 11, compared to Day 1 (i.e. after adaptation of pain), there was higher functional connectivity in the sensorimotor network and the ventromedial prefrontal cortex (vmPFC). After adaptation (i.e. Day 11), the connectivity of the vmPFC became correlated with both pain ratings and the connectivity of the sensorimotor network (Riedl et al. 2011). The findings support the preliminary conclusion that cognitive–affective processing of pain, which is associated with the ACC and the vmPFC, may play a key role in pain adaptation.
8.2.4.2 Cognitive Appraisal of Pain
When individuals are appraising a stimulus, they need to actively engage with it. Therefore, attentional processing and working memory may play a key role for them to focus on the stimuli. Such a ‘high‐level appraisal’, which taps more cognitive efforts, is different from the ‘low‐level appraisal’, which is usually automatic and pre‐attentive (Kalisch et al. 2006). In one study, Kalisch et al. (2006
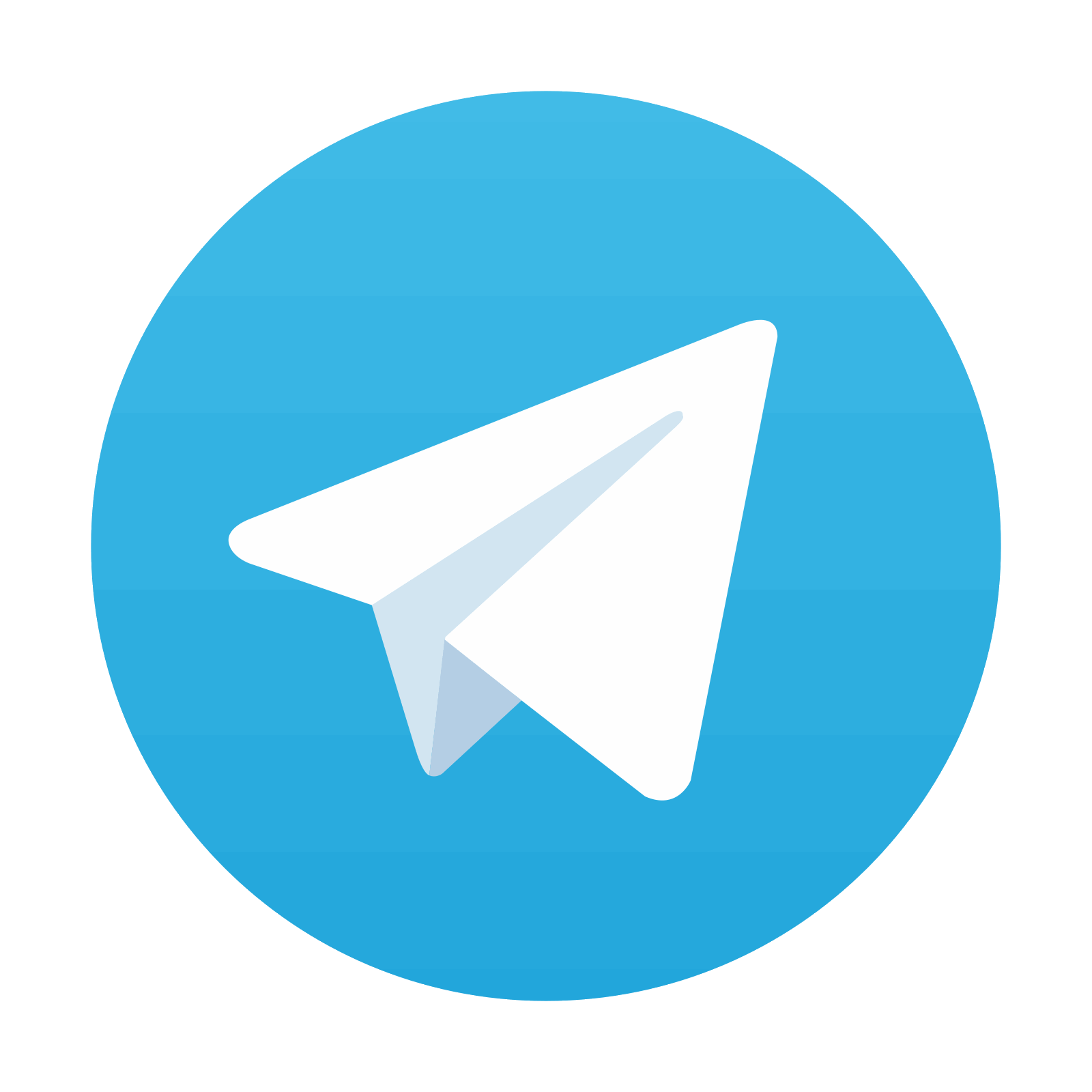
Stay updated, free dental videos. Join our Telegram channel

VIDEdental - Online dental courses
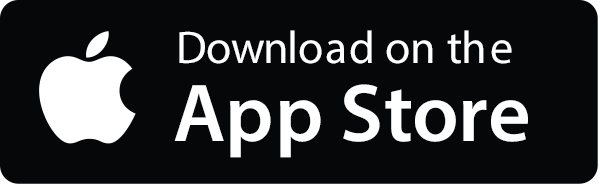
