Assessing Evidence
Einstein purportedly said that “his own major scientific talent was his ability to look at an enormous number of experiments and journal articles, select the very few that were both correct and important, ignore the rest, and build a theory on the right ones.”7 Most evidence-based clinicians aspire toward the same goal when evaluating clinical evidence. In this search for good evidence, a “baloney detection kit”69 can be helpful to separate salesmanship from science and suggestive hints from unequivocal evidence. This chapter introduces 12 tools that might be useful in assessing causality in clinical sciences.
Twelve Tools for Assessing Evidence
Be Skeptical
By 1990 it was concluded that “available data thus strongly support the hypothesis that dietary carotenoids reduce the risk of lung cancer.”80 Beta carotene (β-carotene) was hypothesized to interfere passively with oxidative damage to deoxyribonucleic acid (DNA) and lipoproteins,40 and these beliefs in part translated into $210 million sales of β-carotene in 1997 in the United States. Was this convincing evidence or should it be evaluated skeptically? Two large randomized controlled trials (RCTs) were initiated and both were stopped prematurely because β-carotene increased lung cancer risk, cardiovascular disease risk, and overall mortality risk.1,59 In 2005, the primary investigator of one of the trials reported that “beta-carotene should be regulated as a human carcinogen.”58
Evidence on how to cure, manage, or prevent chronic diseases is notoriously contradictory, inconsistent, and unreliable. Mark Twain reminded people to be careful when reading health books because one may die of a misprint.68 Powerful forces conspire to deliver a preponderance of misleading results:
1. Identifying a successful treatment for chronic diseases can be challenging. It has been estimated that less than 0.1% of all investigated treatments are effective. Because the odds for identifying successful interventions for chronic diseases are so small, most so-called effective treatments identified in small clinical trials turn out to be noneffective or even harmful when evaluated in rigorously conducted pivotal trials.
2. Chronic diseases can be complex and include both environmental and genetic causes. The “obvious” causes of disease, such as radiation, tobacco, and sugars, are often ignored, while more profitable etiologic hypotheses are explored ad nauseam. As a result of such biased epidemiologic research, incomplete and mistaken understandings of chronic disease etiology can lead to a cascade of wrong turns in the exploration of possible diagnosis, prognosis, and treatment.
3. Poor scientific methodology is a common problem permeating most of the evidence that surrounds us. Popular press headlines tell it all: “Lies, damned lies and medical statistics,”66 “Undermined by an error of significance: a widespread misconception among scientists casts doubt on the reliability of a huge amount of research,”51 and “Sloppy stats shame science.”40,70
These factors may all be at play in periodontics suggesting that skepticism is required in the evaluation of periodontal evidence. First, the large number of “effective” periodontal treatments may be a telltale sign of a challenging chronic disease. Before 1917, there were hundreds of pneumonia treatments, none of which worked. Before the advent of antibiotics in the 1940s, the wealth of available tuberculosis treatments was misleading in the sense that none really worked. The current “therapeutic wealth” for periodontal diseases may well mean poverty—an indication of the absence of truly effective treatments—and a suggestion we are dealing with a challenging chronic disease. Second, many no longer regard periodontal diseases as the simple, plaque-related diseases they were thought to be in the mid-twentieth century, but rather as complex diseases. Complex diseases are challenging to diagnose, treat, and investigate. Third, the scientific quality of periodontal studies has been rated as low.5,6 Major landmark trials were analyzed using wrong statistics,39 most randomized studies were not properly randomized,53 and the primary drivers of the periodontitis epidemic may have been misunderstood because of the definition of periodontal diseases as an infectious diseases without properly controlled epidemiologic studies.34,38 The chances that periodontal research somehow managed to escape the scientific challenges and hurdles that were present in research in other chronic diseases appears slim.
Don’t Trust Biologic Plausibility
If an irregular heartbeat increases mortality risk and if encainide can turn an irregular heartbeat into a normal heartbeat, then encainide should improve survival.14 If high serum lipid levels increase myocardial infarction risk and if clofibrate can successfully decrease lipid levels, clofibrate should improve survival.64 If Streptococcus mutans causes dental decay, and if chlorhexidine can eradicate S. mutans, then chlorhexidine can wipe out dental decay. Such “causal chain thinking” (A causes B, B causes C, therefore A causes C) is common and dangerous. These examples of treatment rationales, although seemingly reasonable and biologically plausible, turned out not to help or to harm patients. Causal chain thinking is sometimes referred to as “deductive inference,” “deductive reasoning,” or a “logical system.”
In mathematics, “once the Greeks had developed the deductive method, they were correct in what they did, correct for all time.”64 In medicine or dentistry, decisions based on deductive reasoning have not been “correct for all time” and are certainly not universal. Because of an incomplete understanding of biology, the use of deductive reasoning for clinical decisions may be dangerous. Deductive reasoning largely failed for thousands of years to lead to medical breakthroughs. In evidence-based medicine, evidence that is based on deductive inference is classified as level 5, which is the lowest level of evidence available.
Unfortunately, much of our knowledge on how to prevent, manage, and treat chronic periodontitis depends largely on deductive reasoning. Small, short-term changes in pocket depth or attachment levels have been assumed to translate into tangible, long-term patient benefits, but minimal evidence to support this deductive inference leap is available. In one small study without statistical hypothesis testing, dental plaque was related to the transition from an unnatural inflammation-free condition referred to as “Aarhus superhealthy gingiva” to experimental gingivitis47 (which is different from clinical gingivitis). Such studies do not offer proof that dental plaque bacteria cause destructive periodontal disease. It is even unclear if experimental gingivitis and plaque are correlated at a site-specific level above and beyond what would be expected by chance alone. One subsequent study at the same university, using a similar population, and using a similar experimental design, failed to identify an association between plaque and gingivitis.20 Evidence that personal plaque control affects the most common forms of periodontal diseases is still weak35 and largely based on “biologic plausibility” arguments. A move toward a higher level of evidence (higher than biologic plausibility) is needed to put periodontics on a firmer scientific footing.
What Level of Controlled Evidence Is Available?
1. RCT. Individuals or clusters of individuals are randomly assigned to different exposures and monitored longitudinally for the endpoint of interest. An association between the exposure and the endpoint is present when frequency of the endpoint occurrence differs between the exposure groups. The RCT is the “gold standard” design in clinical research. In evidence-based medicine, RCTs, when properly executed, are referred to as level 1 evidence and the highest (best) level of evidence available.
2. Cohort study. Exposed individuals are compared to nonexposed individuals and monitored longitudinally for the occurrence of the primary endpoint of interest. An association between the exposure and endpoint is present when the frequency of endpoint occurrences differs between exposed and nonexposed individuals. A cohort study is often considered the optimal study design in nonexperimental clinical research (i.e., for those study designs where randomization may not be feasible). In evidence-based medicine, cohort studies, when properly executed, are referred to as level 2 evidence.
3. Case-control study. Cases (individuals with the endpoint of interest) are compared with controls (individuals without the endpoint of interest) with respect to the prevalence of the exposure. If the prevalence of exposure differs between cases and controls, an association between the exposure and the endpoint is present. In a case-control study, it is challenging to select cases and controls in an unbiased manner and to obtain reliable information on possible causes of disease that occurred in the past. The case-control study is the most challenging study design to use for obtaining reliable evidence. As a result, in evidence-based medicine, case-control studies, when properly executed, are the lowest level of evidence.
< ?xml:namespace prefix = "mml" />
Did the Cause Precede the Effect?
In 2001, a study published in the British Medical Journal suggested that retroactive prayer shortened hospital stay in patients with bloodstream infection.45 The only problem was that patients were already dismissed from the hospital when the nonspecified prayer to the nonspecified deity was made. To most scientists, findings in which the effect (shorter hospital stay) precedes the cause (the prayer) are impossible, and this provides an unequivocal example of a violation of correct temporality; the effect preceded the hypothesized cause. In chronic disease research, it is often challenging to disentangle temporality, and fundamental questions regarding temporality often remain disputed. For example, in Alzheimer research the amyloid in the senile plaques in the brain is often considered to be the cause of Alzheimer disease, but some researchers suggested that amyloid may be the result rather than the cause of Alzheimer disease and that the amyloid may be protective.44 Vigorous investigation of temporality is a key aspect in scientific investigation.
Temporality is the only criterion that needs to be satisfied for claiming causality; the cause needs to precede the effect. In periodontal research, many studies relating plaque or specific infections to periodontal diseases suffer from unclear temporality. Are observed microbial profiles the result or the cause of periodontitis? There are no cohort studies in adults that have established that an infectious cause precedes the onset of chronic periodontitis.38 Unequivocal establishment of temporality is an essential element of causality and can be difficult to establish for chronic diseases, including the epidemiology of periodontal diseases.
No Betting on the Horse After the Race Is Over
An acquired immunodeficiency syndrome (AIDS) researcher at an international AIDS conference was jeered when she claimed that AIDS therapy provided a significant benefit for a subgroup of trial participants.57 A study published in the New England Journal of Medicine48 was taken as a textbook example of poor science24 when it claimed that coffee drinking was responsible for more than 50% of the pancreatic cancers in the United States. Results of a large collaborative study demonstrating that aspirin use after myocardial infarction increased mortality risk in patients born under Gemini or Libra provided a comical example of an important scientific principle: data-generated ideas are unreliable.
An essential characteristic of science is that hypotheses or ideas predict observations, not that hypotheses or ideas can be fitted to observations. This essential characteristic of scientific enterprise—prediction—is often lost in medical and dental research when poorly defined prestudy hypotheses result in convoluted data-generated ideas or hypotheses that fit the observed data. It has been reported that even for well-organized studies with carefully written protocols, investigators often do not remember which hypotheses were defined in advance, which hypotheses were data derived, which hypotheses were “a priori” considered plausible, and which were unlikely.82 A wealth of data-generated ideas can be created by exploring patient subgroups, exposures, and endpoints, as shown by the following:
1. Modifying study sample definition. A commonly observed posttrial modification of a hypothesis is to evaluate improper or proper subgroups of the original study sample. Improper subgroups are based on patient characteristics that may have been influenced by the exposure. For example, one may evaluate tumor size only in those patients who survived or pocket depths only in those teeth that were not lost during the maintenance. Results of improper subgroup analyses are almost always meaningless when establishing causality. Proper subgroups are based on patient characteristics that cannot be influenced by the exposure, such as gender, race, or patient’s age. A review of trials in the area of cardiovascular disease suggested that even the results of proper-subgroup analyses turn out to be misleading in a majority of cases.82 In the human immunodeficiency virus (HIV) area, one proper subgroup analysis (based on racial characteristics) drew an investor lawsuit on the basis that company officials “deceived” investors with a “fraudulent scheme.”17
2. Modifying exposure definition. After or during the conduct of a study, the exposure definition can be changed, or the number of exposures under study can be modified. In a controversial trial on the use of antibiotics for middle ear infections, the placebo treatment was replaced with a boutique antibiotic, causing a potentially misleading perception about the antibiotics’ effectiveness.18,22,49 In another example of “betting on the horse after the race was over,” a negative finding for cigarette smoking (the primary exposure) as a cause for pancreatic cancer led reportedly to the data-generated hypothesis that coffee drinking increased pancreatic cancer risk.18 When this study was repeated in the same hospital, using the same protocol, but now with the pretrial hypothesis to evaluate coffee drinking, the results of the prior study could not be duplicated.
3. Modifying endpoint definition. Almost all pivotal trials specify one primary endpoint in the pretrial hypothesis. In periodontal research the absence of a specific pretrial defined endpoint is common and permits effortless changing of the endpoint definition. The typical periodontal trial has six endpoints and does not specify which endpoint is primary, and it is not always clear what is a good or a bad outcome.36 Similarly, the definition of adverse pregnancy outcomes is flexible and susceptible to post-hoc manipulations to squeeze out statistical significance. Statistical trickery to reach desired conclusions under such circumstances may be child’s play.
Deviating from the pretrial hypothesis is often compared to data torturing.52 Detecting the presence of data torturing in a published article is often challenging; just as the talented torturer leaves no scars on the victim’s body, the talented data torturer leaves no marks on the published study. Opportunistic data torturing refers to exploring data without the goal of “proving” a particular point of view. Opportunistic data torturing is an essential aspect of scientific activity and hypothesis generation. Procrustean data torturing refers to exploring data with the goal of proving a particular point of view. Just as the Greek mortal Procrustes fitted guests perfectly to his guest bed either through bodily stretching or through chopping of the legs to ensure correspondence between body height and bed length, so can data be fitted to the pretrial hypothesis by Procrustean means.
What Is a Clinically Relevant Pretrial Hypothesis?
When alendronate was shown to lower fracture rates (a tangible benefit),19 it became the leading worldwide treatment for postmenopausal osteoporosis, and its use is expected to continue to grow.8 When a randomized trial showed that simvastatin saved lives of patients with prior heart disease (a tangible benefit),19 sales increased by 80% in the first 9 months after the study’s publication. A pivotal trial on hormone replacement therapy turned thriving drug sales into a major decline.27 A pivotal trial found a routine eye surgery to be harmful, prompting the National Institutes of Health to send a clinical trial alert to 25,000 ophthalmologists and neurologists.3
Clinically Relevant Endpoint.
An endpoint is a measurement related to a disease process or a condition and used to assess the exposure effect. Two different types of endpoints are recognized. True endpoints are tangible outcomes that directly measure how a patient feels, functions, or survives46; examples include tooth loss, death, and pain. Surrogate endpoints are intangible outcomes used as a substitute for true endpoints74; examples include blood pressure and probing depths of periodontal pockets. Treatment effects on surrogates do not necessarily translate into real clinical benefit (Table 87-1). Use of surrogate endpoints has led to widespread use of deadly medications, and it has been suggested that such disasters should prompt policy changes in drug approval.63 Most major causes of human disease (e.g., cigarette smoking) were identified through studies using true endpoints. A first requirement for a clinically relevant study is the pretrial specification of a true endpoint.
TABLE 87-1
Examples of Potentially Misleading Surrogates*
Disease/Conditions | Experimental Treatment | Control Treatment | Effect on Surrogate Endpoint | Effect on True Endpoint | Misleading Conclusion | Reference |
AIDS | Immediate zidovudine | Delayed zidovudine | Significant increase of 30-35 CD4 cells/mm3. | No change in incidence of AIDS, AIDS-related complex, or survival. | False-positive | 80 |
Osteoporosis | Fluoride | Placebo | Significant increase of 16% in bone mineral density of lumbar spine. | Nonvertebral fracture rates increased by 85%. | False-positive | |
Lung cancer | ZD1839 (Iressa) | Placebo | Dramatic tumor shrinkage in 10% of patients. | No effect. | False-positive | 82 |
Aphthous ulcers | Thalidomide | Placebo | Although thalidomide expected to decrease TNF-α production, significant increase of 4.4 pg/mL in TNF-α production occurred, suggesting harm. | Pain diminished and ability to eat improved. | False-negative | 32 |
Edentulism dentures | Implant-supported | Conventional dentures | No impact on chewing cycles. | Improved oral health–related quality of life | False-negative | 5 |
Prostate cancer | Radical prostatectomy | Watchful waiting | Substantial elimination of tumor mass. | No effect on overall mortality risk. |
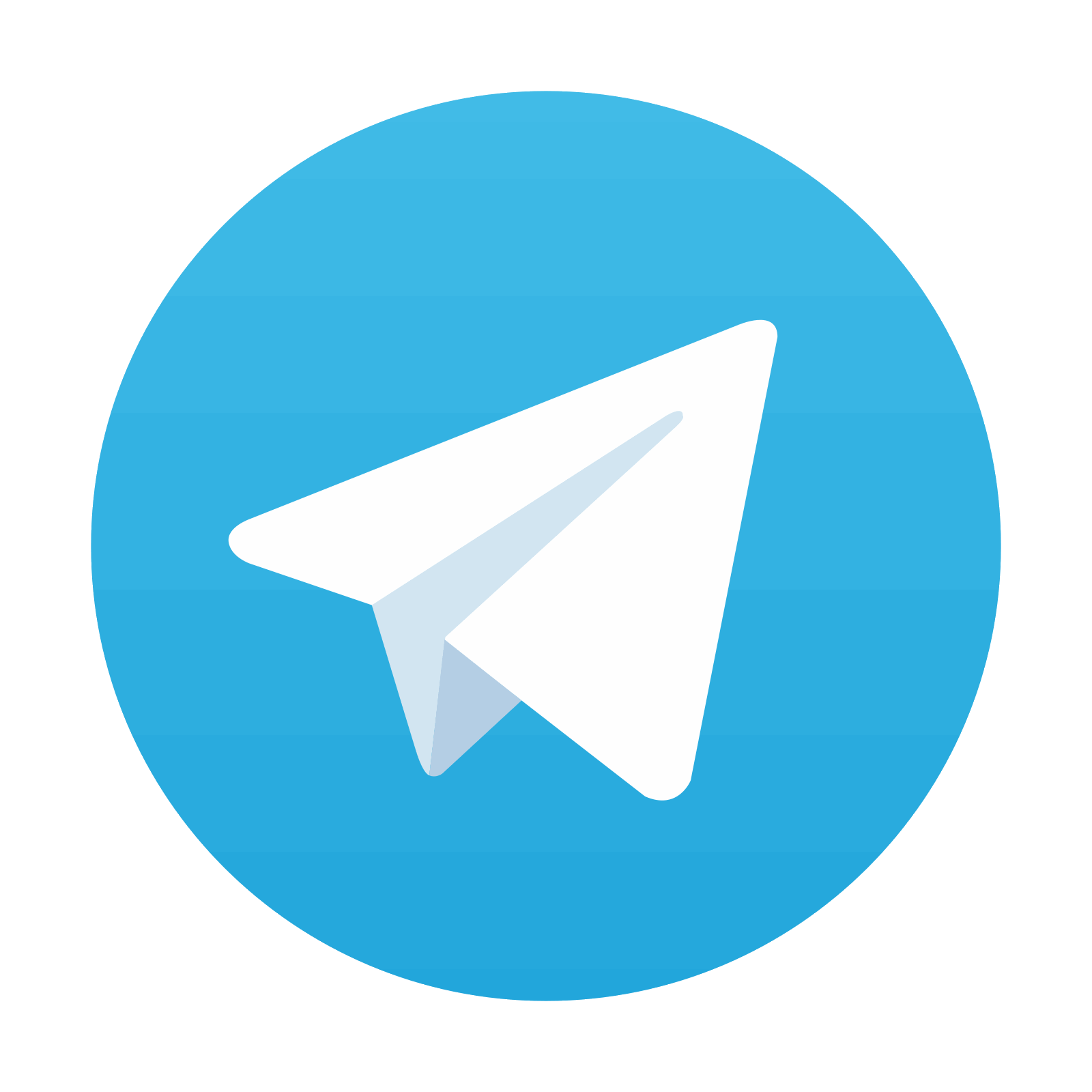
Stay updated, free dental videos. Join our Telegram channel

VIDEdental - Online dental courses
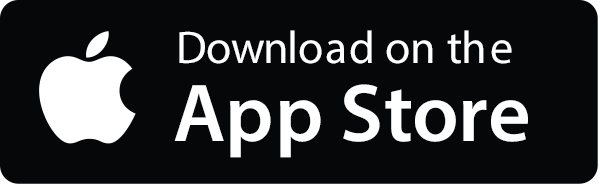
