In the simple linear regression model, we used a continuous outcome (days to align) and a single continuous predictor (irregularity index). The flexibility of the multiple regression model allows us to use a continuous outcome (days to align) with 2 or more predictors that can be continuous or categorical. We can also use a single categorical predictor of 2 or more levels to predict a continuous outcome such as days to align. If the categorical predictor has only 2 levels such as sex (male, female), then the simple regression analysis is equivalent to an independent t test. If the single categorical variable has more than 2 levels, then the simple linear regression is equivalent to 1-way analysis of variance (ANOVA). If we have 2 categorical predictor variables with 2 or more levels, linear regression is equivalent to 2-way or a higher level of ANOVA. This flexibility of the regression models allows us to perform most analyses using a unified approach. Using linear regression instead of a t test or ANOVA allows us to directly obtain estimates (differences between treatment groups) along with their confidence intervals instead of only P values.
Additionally, interaction terms can be included, and the interaction can be evaluated. However, the interpretation of the results from linear regression compared with ANOVA with an interaction might be slightly different because of the coding of the variables.
From t test to linear regression
In a previous example, we compared the ages of female and male patients in a sample using a t test, and the results are shown in Table I . In our sample, the mean age is 4.95 years lower in female than in male patients, with a range of 7.66 to 2.23 years lower; this is a statistically significant finding. Exactly the same analysis can be conducted by using linear regression as shown in Table II .
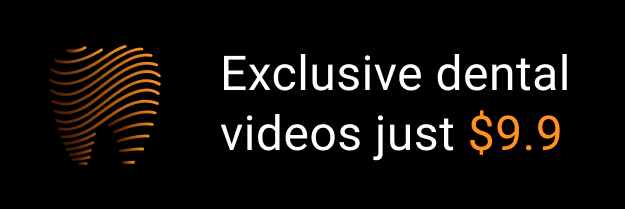