Abstract
Objectives
Conventional 3-dimensional color spaces such as L * a * b * or L * C * h have a limitation in that colors of materials can only be separated on the same hyperplane. Therefore, it would be useful to find appropriate axes for dental color analysis by analyzing spectral data itself, rather than conventional 3-dimensional color spaces.
Methods
Hyperspectral data are detailed color spectra with narrow spectral bands over a continuous spectral range. We acquired hyperspectral data of the shade guides without specular reflection, and standardized them as reflectance data. Then, reflectance data were weighed by luminous efficiency function, and used in principal component analysis (PCA). Principal components (PCs) and their contribution, and values of respective shades to respective PCs were calculated as PC scores.
Results
Cumulate contribution rate of 1st to 3rd PCs were approximately 100%, which meant shade colors were very similar to each other. Respective PCs showed specific figures, and values of shades showed sequences unique to each PC, which were independent of each other; values to the 1st PC showed gradual changes with change in shade numbering, values to the 2nd PC showed relatively high scores on opaque shades, values to the 4th PC showed lower scores on B and C group shades, and values to the 6th PC showed differences between manufacturers.
Significance
Using PCA, we could find axes independent of the conventional 3-dimensional color spaces. These axes reflected certain changes which are not detected on conventional color spaces. Our methods are taking into account color matching under any illumination by focusing on the spectra themselves, and we can discuss about components of the teeth from spectra of resulting principal components. By applying our method to conventional systems, it would help diagnose color differences of dental materials.
1
Introduction
Accurate color matching of the dental shade is one of the most challenging aspects of dental restoration from the esthetic standpoint, because all objects have a specific spectral reflectance. Even if two objects appear the same under a certain illumination, they appear different under other illuminations due to metameric matching .
Up to now, many 3-dimensional color spaces based on retinal sensitivity have been proposed for color analysis, e.g., CIE 1931 RGB , CIE 1931 XYZ , CIE 1976 L * a * b *, CIE 1976 L * u * v *, and CIE 1976 L * C * h . Above all, L * a * b * or L * C * h is commonly employed for tooth color analysis because L * a * b * is a uniform color space which is suitable for measuring color difference, and L * C * h well reflects human senses. However, these three axes, e.g., L *, a *, and b *, are not always suitable for dental color analysis. Because a * and b * were determined as green–magenta axis and blue–yellow axis, and variation of dental color does not follow these axes. Furthermore, inseparable colors in a certain color space cannot be distinguished in any other color space, as all of these color spaces are derived from the same color space. In particular, XYZ is a linear transformation of RGB color space. L * a * b * and L * u * v * are uniform color spaces derived from XYZ by a different nonlinear transformation, and L * C * h is a cylindrical representation of L * u * v *. In other words, 3-dimensional color space is a hyperplane in the high-dimensional world, and a linear/nonlinear transformation is a shifting or distortion of each axis on the same hyperplane. If the 3-dimensional hyperplane was inappropriate, no axis on the hyperplane would be suitable for discrimination of the samples.
Previously, various types of color acquisition systems and imaging systems have been employed for tooth and shade color analysis, e.g., digital cameras, colorimeters, spectrophotometers, and spectroradiometers . A digital camera is a simple and easy-to-use system for 3-color image acquisition . Each camera has a specific sensitivity and can be converted to device-independent L * a * b * color space by calibration. However, it cannot simulate various illuminations. Some studies adopted a multispectral/hyperspectral system including a spectroradiometer or spectrophotometer . Multispectral/hyperspectral data are consisting of more than three bands. In general, multispectral data are of several bands, and hyperspectral data are of much more bands which can be shown as spectra. The system allows precise color acquisition, but size of the dataset is too large to process (e.g., the spectroradiometer we used acquires 401-dimensional data). To solve this problem, the data were compressed into 3-dimensional color space . This method is better than the original 3-dimensional acquisition by a 3-dimensional imaging system, because it allows higher bit depth and emulates various illuminations . However, 3-dimensional color matching requires simulation of any illumination for complete color matching. The problems due to the inappropriate hyperplane still remain. For detecting lesions such as caries, hyperspectral imaging is effective . They focused on spectral differences among lesions, and subtraction between wavelengths was proposed for regional analysis. We have employed similar method for regional tongue analysis . These methods are suitable for discriminate apparent difference between regions. However, they are inappropriate for detailed analysis of spectra, because it requires only a few wavelengths for analysis and discarding information of other wavelengths.
We have already proposed utilization of principal component analysis (PCA) to inspect correlations between tongue color spectra and medical diagnosis . PCA is a mathematical procedure that uses an orthogonal transformation to convert a set of observations of possibly correlated variables into a set of values of uncorrelated variables called principal components, and often used to compress data dimensions. Using PCA and vector rotation method, we were able to separate spectral color differences between individuals, which could not be achieved on conventional 3-dimensional color spaces, that is, the method effectively found the appropriate axes for distinguishing and separating hyperspectral data. Additionally, as this system is focusing on spectral properties themselves, it is adaptable for color matching under any illumination. Resulting spectra is useful to presume physical and chemical components of the teeth which are effecting on their color.
The objective of our study is to find the appropriate axes for dental color analysis, other than conventional 3-dimensional color spaces, by analyzing spectral data themselves. As analyzing spectral data themselves is useful for dental color analysis, we acquired hyperspectral data without specular reflection, and performed PCA to set appropriate axes for data separation.
2
Materials and methods
2.1
Materials
Two series of shade guides, BEAUTIFIL II (SHOFU INC., Kyoto, Japan) and CLEARFIL MAJESTY (KURARAY MEDICAL INC., Tokyo, Japan), were used in the analysis. In total 28 shades were used; BEAUTIFIL II is of 12 shades (A1, A2, A3, A3.5, A4, B2, B3, C3, Inc, BW, A2O, A3O) and CLEARFIL MAJESTY is of 16 shades (A1, A2, A3, A3.5, A4, B2, B3, C3, XL, HO, OA2, OA3, OA4, E, OC, T). In the series, the shades are numbered sequentially in order of color density, but the color change was not sequential and differed between manufacturers in multispectral view. A spectroradiometer (CS-2000, KONICA MINOLTA HOLDINGS, INC., Tokyo, Japan) was equipped with a circular polarizer (Zéta EX C-PL Filter, Kenko Co., Ltd., Tokyo, Japan) and used for spectral data acquisition. The spectroradiometer features a spectral range of 380–780 nm containing 401 bands of 1-nm resolution. Two artificial sunlight lamps (XC-100A, SERIC LTD., Tokyo, Japan) were equipped with linear polarizers and utilized as the light source. The artificial sunlight lamp features a color temperature of 5500 K, spectral distribution of 370–780 nm, and luminous intensity of 3000 cd.
2.2
Data acquisition setup
Scheme of the data acquisition system is shown in Fig. 1 . To measure spectral data of the shades considering its transparency, the shades were put on a black or white background. A black patch of the color chart (Muncell ColorChecker Classic, X-Rite, MI, USA) and the diffuse reflection standard (White Calibration Plate CS-A5, KONICA MINOLTA HOLDINGS, INC., Tokyo, Japan) are with the known spectral reflectance, and were used as the black and white background. Two lamps illuminated the shades from 45°, both sides. The spectroradiometer was placed orthogonal to the surface of the shades at 80 cm distance, and an area of 0.1° (1.4 mm in diameter) was measured. Two polarizers in front of the lamps were set parallel to each other, and the polarizer in front of the spectroradiometer was rotated and set where the view on the finder was the darkest in order to remove all specular reflection. Spectral data were acquired by 401 bands for two backgrounds, thus each sample has 802-dimensional data. Spectral data of the diffuse reflection standard was also measured for the subsequent standardization.
2.3
Standardization and optimization of spectral data
Acquired spectral data were standardized as spectral reflectance, which represents the specific color of the object. Values of respective wavelengths of a sample were divided by those of the diffuse reflection standard with known value. Spectral reflectance data were first converted into 3-dimensional L * a * b * color space under standard D65 illumination to show the efficiency of L * a * b * in separating color of shades . Then, spectral reflectance data were weighed to fit human senses by human visual sensitivity function. Dental restoration is especially required to fit the sensitivity of the eyes, i.e., wavelengths of high sensitivity should be weighed. Thus, each spectral reflectance datum was first multiplied by the photopic luminous efficiency function . Each datum became 782-dimensions deep, since the function was provided from 390 to 790 nm.
2.4
Principal component analysis
Principal component analysis (PCA) is a mathematical procedure that uses an orthogonal transformation to convert a set of observations of possibly correlated variables into a set of values of uncorrelated variables called principal components (PCs) . In brief, PCA is a method to set a new coordinate system. In the space where the samples are distributed, we can determine one axis with the greatest variance passing through the multidimensional mean, and this axis is called the first PC. Then, the axis orthogonal to the first PC with the second greatest variance passing through the multidimensional mean is denoted as the second PC. Next, the axis orthogonal to the first and second PC with the third largest variance passing through the multidimensional mean is denoted as the third PC, and so on. Values of the samples on each new axis are called PC scores, i.e., the weight of each sample on each principal component axis. Sample variance on each PC shows its contribution, called eigenvalue. Total eigenvalue is equal to data dimension, and fraction of each eigenvalue was calculated as the contribution rate of each PC.
In this research, every sample has 782-dimensional data, 390–780 nm (1 nm step), black and white background. On the 782-dimensional space, each sample can be written as follows,
n 1 ⋅ R → 390 b + n 2 ⋅ R → 391 b + ⋯ + n 781 ⋅ R → 779 w + n 782 ⋅ R → 780 w
where <SPAN role=presentation tabIndex=0 id=MathJax-Element-2-Frame class=MathJax style="POSITION: relative" data-mathml='R→’>R→R→
R →
is a unit vector of each wavelength and background; subscript number denotes its wavelength, and superscripts b and w denote black or white background. n x denotes x th coefficient for <SPAN role=presentation tabIndex=0 id=MathJax-Element-3-Frame class=MathJax style="POSITION: relative" data-mathml='R→’>R→R→
R →
, equal to weighed spectral reflectance value at the wavelength. All <SPAN role=presentation tabIndex=0 id=MathJax-Element-4-Frame class=MathJax style="POSITION: relative" data-mathml='R→’>R→R→
R →
are uncorrelated and orthogonal to each other by their definition.
Then, PCs were calculated as the samples on the 782-dimensional space , and Eq. (1) is expressed as follows,
m 1 + P → 1 + m 2 ⋅ P → 2 + ⋯ + m 781 ⋅ P → 781 + m 782 ⋅ P → 782
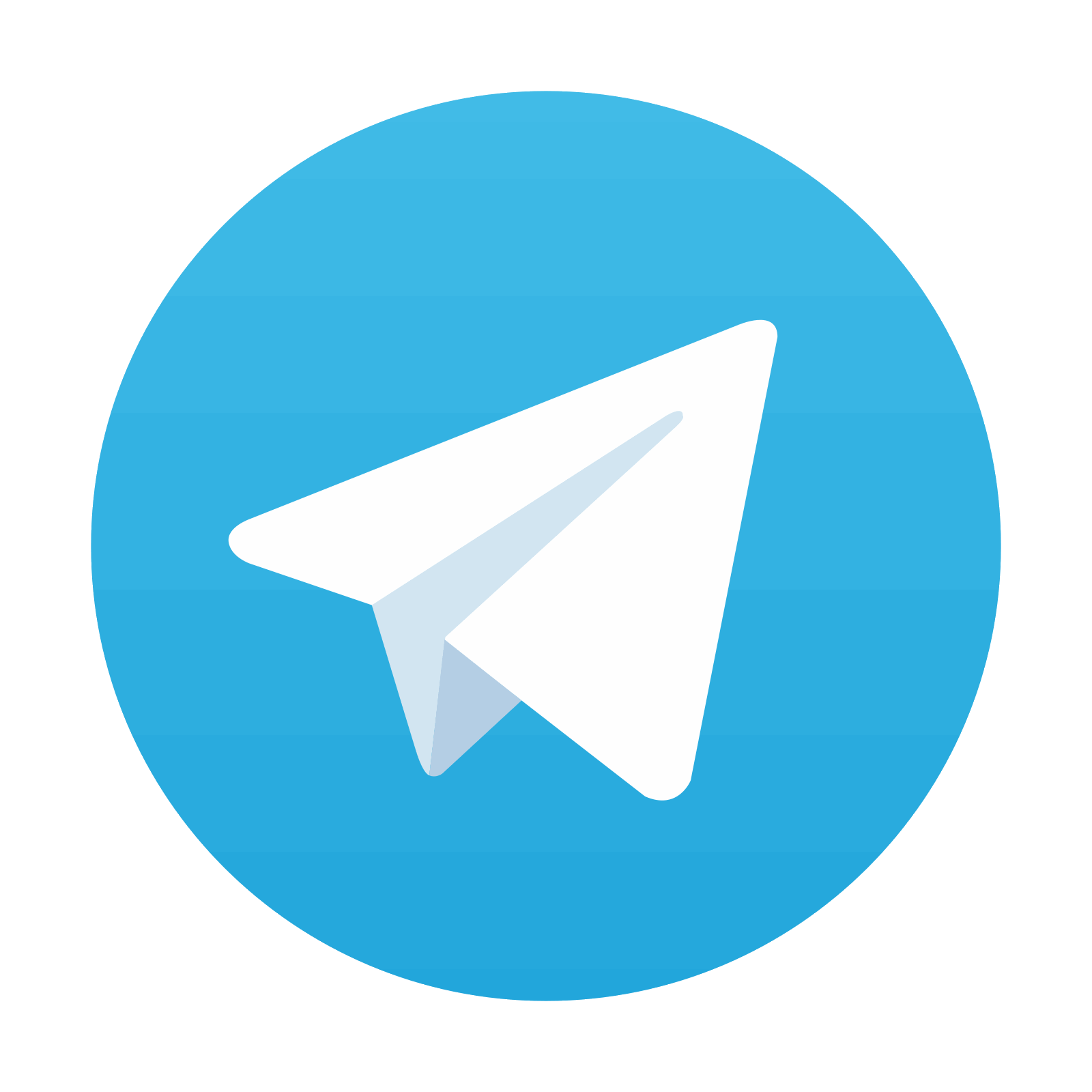
Stay updated, free dental videos. Join our Telegram channel

VIDEdental - Online dental courses
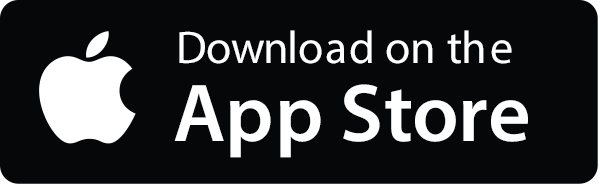
