Chapter 13. Issues of Bias and Confounding in Clinical Studies
Elliot Abt, D.D.S., M.S., M.Sc.; Jaana Gold, D.D.S., M.P.H., Ph.D., C.P.H.; and Julie Frantsve-Hawley, Ph.D., C.A.E.
In This Chapter:
Introduction
Bias and confounding are two phenomena that can distort the results of a study, thus lowering validity (internal validity) and applicability (external validity). Bias is a systematic error in the design, conduction, or data analysis that leads to an incorrect assessment of the true effect of an exposure (or intervention) on an outcome.1 Confounding, on the other hand, is the presence of a third factor that can alter the association between an exposure and an outcome.
Investigators may make wrong conclusions about the beneficial or harmful effects of a tested treatment, and it is important for clinicians to understand how bias can impact study results.2 Bias can be intentional—which is considered unethical and one should expect that this not be done—or unintentional, as a result of poor methodology.
It is important to note that one cannot assess the absolute impact that bias has on a study. However, one can and should assess the potential or the risk that bias could have impacted results and conclusions. Bias can also cause associations to be either larger (overestimation) or smaller (underestimation) than the true associations.3 Oftentimes, little can be done when bias has occurred as there are no statistical tests that can control for bias. However, it can be minimized when a study is carefully designed and conducted.4 Potential sources of bias can differ among study designs. Conversely, confounding can be minimized in the design and/or analysis phase of a study.
Specific concerns about confounding and the different types of biases found in clinical trials, prognostic studies, and diagnostic test studies will be discussed in more detail in this chapter.
Confounding
Confounding occurs when a variable is associated with both an exposure (or intervention) and the outcome of interest.1 A true confounder must be associated with the exposure and be an independent risk factor for the outcome of interest without being in the causal pathway between exposure and outcome. Additionally, a confounder must be distributed unequally among study groups. Issues of confounding are problematic because they can distort true associations between exposure and outcome by either exaggeration or minimization. That is, confounding can bias the association away from the null (positive confounding) or toward the null (negative confounding), and the magnitude of such confounding can be small, moderate, or large.
Age and smoking are common confounders. An association between diabetes and periodontal disease may be confounded by age if not controlled for in either the study design or statistical analysis. One might find an association between coffee drinking (exposure) and cardiovascular disease (outcome). This association should raise suspicion for confounding, as there is little evidence linking exposure and outcome. Smoking may be confounding this relationship if coffee drinkers are more likely to smoke than nonsmokers, because smoking is a known risk factor for cardiovascular disease. This would be an example of positive confounding if further analysis found that coffee drinking has no effect on cardiovascular disease.
Diseases that share several risk factors can be confounded in poorly designed or analyzed studies. Studies assessing the relationship between periodontal disease and coronary heart disease can be confounded with common risk factors such as age, smoking, stress, socioeconomic status, and obesity.5
Control of Confounding
Confounding can be controlled for in the design phase, the analysis phase, or a combination of both. In the study design phase, randomization, restriction, and stratification can be used in randomized trials, whereas matching is often done in observational studies. In the analysis stage, statistical methods such as stratification of analysis (adjusting) and multiple (or logistic) regression analyses are commonly employed.
The single best method to control for confounding is randomization, a powerful tool that controls for all known and unknown confounders (see Chapter 3). However, randomization may not ensure that potential confounders are distributed equally among study groups. For example, appropriate randomization could result in unequal numbers of smokers in control and treatment groups. This is when stratification or restriction can be employed. Stratification of randomization calls for randomizing smokers separately from nonsmokers to ensure equal distribution among groups. Restriction would involve preventing smokers from meeting the inclusion criteria for participation, thus eliminating smoking as a potential confounder. The disadvantage of restriction is that the effect of an intervention among smokers would be unknown.
Observational studies are highly affected by confounding as true randomization is not employed (see Chapter 4). In a case-control study, a patient with a particular condition or disease (case) is matched with another person without the condition (control) who is similar in age, gender, ethnicity, and/or socioeconomic status. The goal is to mimic randomization by having groups as similar as possible to each other to minimize potential confounding factors.
In the analysis phase, stratification of analysis refers to separately analyzing groups. Thus, for coffee drinking/cardiovascular disease, separate 2 x 2 tables (see Chapter 12) would be done for smokers and nonsmokers. This stratification provides an adjusted result, which may or may not be different from a crude (unadjusted) result. Although many criteria exist, if the results of the stratified analysis differ from the crude result by more than 10%, confounding may be present. In the above example, if the crude risk ratio is 2.5 and the adjusted risk ratio is 1.1, clearly the association between coffee drinking and cardiovascular disease has been positively confounded by smoking. That is, smoking has exaggerated the effects of the exposure on the outcome.
If multiple potential confounders are suspected, rather than numerous 2 x 2 tables being employed, multiple (or logistic) regression analysis can be performed. This is a statistical technique whereby the effect of many potential confounders, termed “input variables,” can be measured on a single “outcome variable.” Regression is an effective statistical tool to simultaneously observe whether multiple variables are or are not confounding an outcome. An example of the effects of confounding is provided in Box 13.1.
Box 13.1. Example of Confounding in Dentistry
Study: “Periodontitis Increases the Risk of a First Myocardial Infarction: A Report from the PAROKRANK Study.” Rydén et al., 2016.6
Study Design: Case-control study of 805 patients with myocardial infarction (MI) (cases) matched with 805 patients without MI (controls). All patients were given a periodontal assessment, which included a panoramic radiograph, and periodontal status was classified as healthy, mild-moderate disease, or severe disease based on percentage of alveolar bone loss.
Results: Crude odds ratio (OR) = 1.49 (95% confidence interval [CI], 1.21 to 1.83), and an adjusted OR = 1.28 (95% CI, 1.03 to 1.60). This means that patients with MI had 49% greater odds of having periodontal disease, but when adjusted for potential confounders including smoking and diabetes, this percentage dropped to 28%.
Appraisal: As both the crude and adjusted odds ratios were statistically significant, the authors claimed a relationship exists between periodontal disease and MI independent of confounding. However, the change in odds ratio from crude to adjusted was greater than 10%, which suggests that there was confounding present, pushing the association between outcome and exposure further away from the null value of 1, reducing the strength of the association. This is an example of positive confounding, where potential confounding variables exaggerated the relationship between outcome and exposure. Thus, it appears likely that potential confounders such as smoking and diabetes were distorting the relationship between periodontal disease and MI. Additionally, the reader should consider the magnitude of the effect. From case-control studies, odds ratios greater than 4 are generally needed to overcome the potential effects of bias and confounding.7 With odds ratios only slightly greater than the null, this magnitude is not generally impressive enough to support causal inference. A scientific statement from the American Heart Association does not support a causal relationship between periodontal disease and cardiovascular disease.8
Bias
Bias in Therapy Studies
Clinicians are often interested in the effectiveness of a treatment, therapy, or preventive intervention. A therapy or treatment can be defined as any intervention—which may include prescribing drugs, performing surgery, or counseling—that is intended to improve the course of or prevent the onset of disease.9 Randomized controlled trials (RCTs) (see Chapter 3) and systematic reviews (see Chapter 6) of RCTs are the best study designs to provide evidence for clinicians in their decisions on therapeutic interventions.4,10 Again, randomization controls for (but may not eliminate) all known and unknown confounders. In an RCT, study subjects are randomly allocated into two or more groups, usually treatment and control groups, and then followed for a certain time period to assess how the treatment affects the selected outcome(s). When critically appraising therapy studies, the reader needs to assess the following domains: sequence generation (selection bias), allocation concealment (selection bias), blinding of participants and personnel (performance bias), blinding of outcome assessment (detection bias), incomplete outcome data (attrition bias), selective outcome reporting (reporting bias), and other potential sources of bias (Figure 13.1).
Selection bias refers to systematic errors in how study groups were derived.11 Study subjects should be reflective of the general population from which the study sample is intended to be generalized, and a potential for bias can occur when efforts are not made to ensure that this is in fact the case. When separate criteria are used to recruit and enroll patients into different study groups, selection bias can result. In clinical trials, selection bias can occur if patients with better anticipated outcomes are allocated to a treatment group and those with worse outcomes are allocated to the control group. An example of selection bias can be identified in a coronary artery surgery study in which coronary bypass surgery was compared with medical therapy.12 The groups differed in the degree of baseline coronary artery disease. The medical therapy group had more extensive baseline coronary artery disease, which ultimately biased the results.
Selection bias can also occur when people who volunteer and agree to participate in a study are different than “regular” control subjects or from those who do not participate. This bias, sometimes called response bias, may favor the treatment group if volunteers are more motivated and concerned about their health. For example, in an oral hygiene study, people with better oral hygiene practices may be more likely to respond and agree to participate in the study.
Clinicians can identify studies where the impact of selection bias was minimized or reduced by evaluating how randomization was implemented, which consists of sequence generation and allocation concealment.11 Sequence generation refers to how the randomization schedule is achieved; this is best accomplished by drawing lots or using a computer to generate numbers. However, even a well-prepared (typically, computer-generated) randomization schedule does not ensure random allocation. Examples of inappropriate sequence generation would be randomization via alternation (that is, one patient goes to the intervention arm, the next one is allocated to the control arm, the third one back to the intervention arm, and so on), date of birth, or last-name alphabetization. Allocation concealment, in which the investigators do not know the treatment allocation prior to the start of a study, lowers the risk of selection bias. Lack of proper allocation concealment can lead to spurious study results. Schulz et al.13 reported that trials in which allocation concealment was either inadequate or unclear can exaggerate treatment effects as much as 40%.
Figure 13.1. Bias Elements for Therapy Studies
|
Clinicians can identify studies where the impact of selection bias was minimized or reduced by evaluating how randomization was implemented, which consists of sequence generation and allocation concealment.11 |
Performance bias refers to systematic errors in how researchers implement the interventions under investigation.4,11 For RCTs, both the control group and the treatment group should receive similar care except for the intervention or exposure being studied. Any additional differences in treatments between groups could alter the study results. For example, groups or individuals are treated unequally if some receive more or different oral health education or more treatment visits than others. Additionally, knowledge of the interventions or therapy by the participants, study personnel, or investigators can be reduced by blinding, which can reduce performance bias. However, blinding is not always possible, because of the nature of the therapy or treatment. For example, in clinical trials comparing composite to amalgam restorations, blinding of both investigators and participants would not be possible.14
Detection bias refers to systematic differences in how the outcomes are measured and evaluated.4,11 In clinical trials, the concern is that detection bias can influence the study results by over- or underestimating the treatment effect. That is, investigators may be aware of the specific therapy provided and may assign a more favorable outcome to the participants from one group compared with those from the other group. Blinding of outcome assessors (that is, those assessing or adjudicating an outcome) is one way to overcome this issue. This is especially important when assessing subjective outcomes, such as postoperative pain.11 In clinical studies in which the outcome is very different between groups and is clinically visible or recognizable, it may be impossible to blind those assessing clinical outcomes. For example, trials of silver diamine fluoride would reveal black-stained arrested dentinal lesions.15 However, even in these circumstances, nonclinical personnel analyzing data can often be blinded.
Attrition bias can occur when loss to follow-up is significant within an entire study and/or when fundamental differences in the reason for or percentage of dropouts exist between study arms. This brings into question whether something about the study conduct, or the intervention/exposure, is influencing subjects’ continuation in the study. For example, if patients with adverse effects are more likely to drop out of a study, the resultant data from the retained patients is likely to be distorted. Patients may become unavailable for examinations during the study period because they no longer want to participate (noncompliance) or cannot be contacted. In a clinical trial, patients could withdraw from the treatment group if the treatment is causing side effects, is not tolerated well, or causes other symptoms. Loss of participants can affect the validity/results of a study. It has been suggested that even less than a 5% loss can lead to a small bias; however, greater loss can be a threat to the validity of the study.16
|
Attrition bias can occur when loss to follow-up is significant within an entire study and/or when fundamental differences in the reason for or percentage of dropouts exist between study arms. |
To preserve the prognostic balance afforded by randomization, all randomized patients should be included in the final analysis and in their original groups. The analysis should be performed according to the intention-to-treat (ITT) principle in which the primary outcome is recorded for all randomized patients throughout the follow-up period. An ITT analyzes data “as randomized,”17 and it ignores noncompliance, protocol deviations, withdrawal, and anything that happens after randomization. A numerical example of the importance of ITT would be if 20 patients were originally in a study arm and five patients experienced success; the success rate would be 5/20, or 25%. However, if 10 patients dropped out of the study and their data was not analyzed, success would then be 5/10, or 50%. Thus, failure to employ an ITT principle would double the success rate. An ITT approach was used in a study by Weintraub et al.18 on fluoride varnish efficacy in preventing caries in young children, which showed a beneficial effect of fluoride varnish in caries incidence. The primary analysis included data from all children regardless of their adherence to the study protocol.
Reporting bias refers to systematic errors in reporting study results. This may occur when only those results that support the intervention under testing and/or statistically significant results are reported. When researchers, sponsors, and editors prefer to publish only positive or significant results and leave studies with nonsignificant findings unpublished, this is referred to as publication bias.3,10,19–22 Publication bias has been a problem in health research for many years. Studies with statistically significant results are more likely to be published, published multiple times, or published in higher-impact journals (see Chapter 14). Efforts have been made to address this bias by requiring that clinical trial methods be preregistered with organizations such as ClinicalTrials.gov to ensure that analyses are determined a priori and reported as planned. With clinical trials, withholding negative results from publication could have a major impact on the quality and safety of our health care system.20,22 Publication bias can exaggerate results of systematic reviews and meta-analyses, which can impact scientific validity.19,20 One of the most commonly used tests to detect publication bias in systematic reviews is the funnel plot.20 Unfortunately, a trend for a significant increase in publication bias over the years has been reported22 and evidence on publication bias in the dental literature is limited.19
Systematic reviews of randomized trials should have strict inclusion/exclusion criteria as well as an assessment of the quality of included studies. Reviews that include studies at high risk of bias can overestimate treatment effects by 40%.23 In a Cochrane review by Rasines Alcaraz et al.,14 all seven included trials were at high risk of bias because of significant issues with the reporting, and the final analysis included only two studies. The authors concluded that there was low-quality evidence on the risk of recurrent caries with resin composites as compared with amalgam restorations. Many Cochrane reviews have similar conclusions because of the high risk of bias in individual RCTs. For example, a Cochrane review by Riley et al.24 included 10 RCTs assessing the effects of xylitol on dental caries with seven studies assessed at high risk of bias. However, readers need to remember that insufficient evidence from systematic reviews does not mean a particular therapy is not working or that treatment is not effective. An example of assessing bias in a therapy study from the dental literature is provided in Box 13.2.
Box 13.2. Example of Assessing Bias in a Dental Therapy Study
Study: “Treatment of Deep Caries Lesions in Adults: Randomized Clinical Trials Comparing Stepwise vs. Direct Complete Excavation, and Direct Pulp Capping vs. Partial Pulpotomy,” Bjørndal et al., 201025
Study Design: Multicenter randomized controlled trial of 314 patients with deep carious lesions that received partial caries removal, followed by complete excavation at a later date (treatment group) or complete caries excavation. The two primary outcomes measured were pulpal exposure and maintenance of pulp vitality.
Results: Partial or stepwise caries removal resulted in a lower risk of pulp exposure (risk difference = 11.4%; 95% confidence interval [CI], 1.2 to 21.3) and an increased odds of success, defined as maintaining vitality at one year, of 74% (odds ratio=1.74; 95% CI, 1.03 to 2.94).
Appraisal: Selection bias was avoided by having sequence generation performed by computer-generated numbers, and allocation concealment was achieved by central telephone randomization. Adequate randomization controls for but does not always eliminate confounding, as baseline differences between groups may persist. To lower this risk, the investigators stratified the randomization scheme by creating different strata (in this case, for pain, age, and location) whereby randomization is performed within each stratum.1 That is, patients with and without pain, greater or less than 50 years of age, and being treated at one of six centers were done in blocks of six to more equally distribute these prognostic variables between control and treatment groups. Performance bias was minimized by blinding patients and treating both groups equally, apart from the intervention. Although investigators cannot be blinded, those evaluating radiographs and statistical analyses were unaware of group assignment, thus lowering the risk of detection bias. Attrition bias also appeared to be minimal, as only 7% of patients were lost to follow-up and the numbers were similar for both groups. Although it was unclear if an intention-to-treat analysis was performed, there was no evidence of reporting bias.
From a methodological standpoint, this randomized controlled trial was well done as close attention was paid to the multiple factors that can lead to bias, which can negatively impact the validity of an investigation. Understanding the domains of quality in randomized trials can help readers of the dental literature develop good critical appraisal skills.
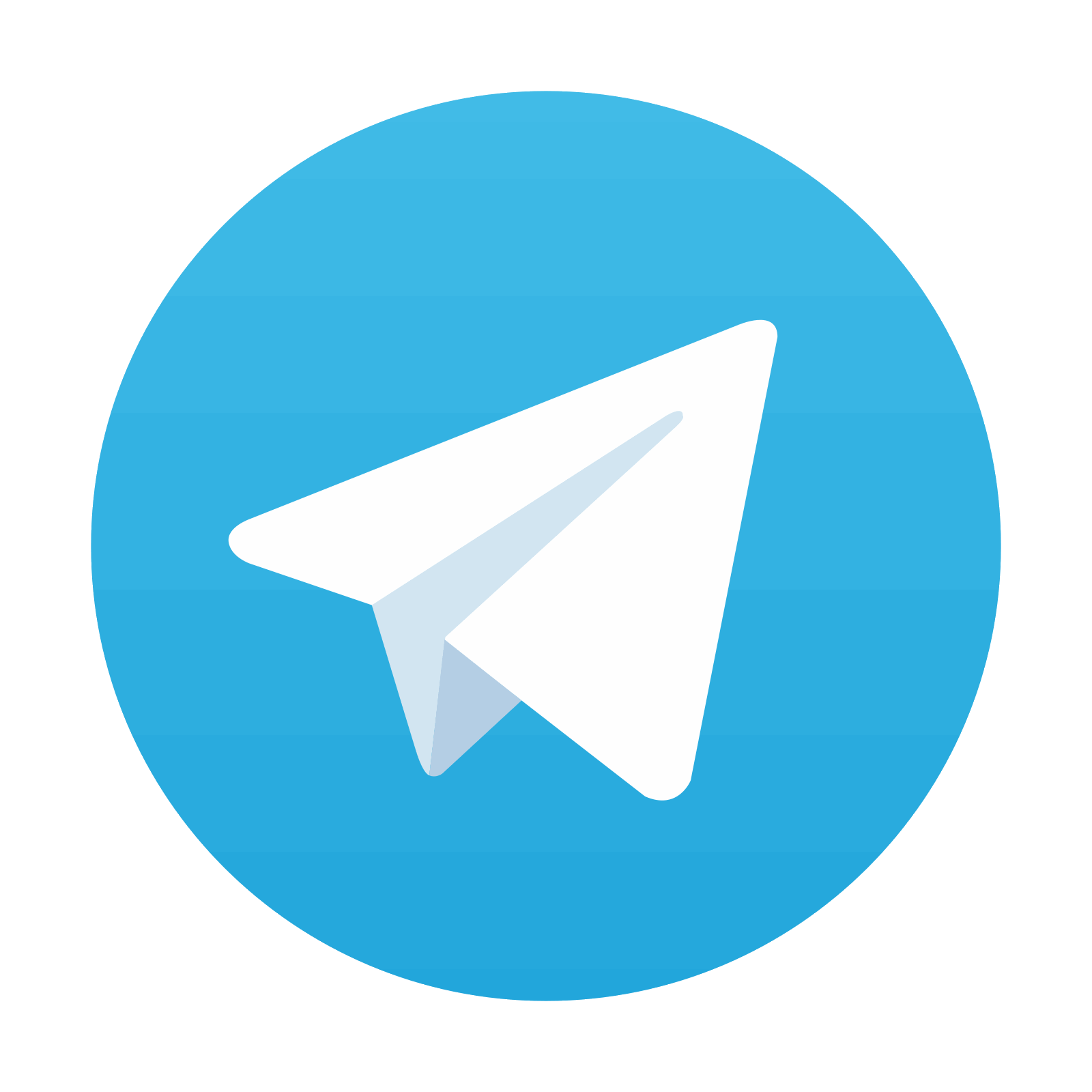
Stay updated, free dental videos. Join our Telegram channel

VIDEdental - Online dental courses
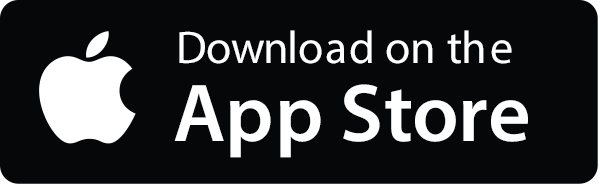
