Fig. 1.1
Evidence-based dentistry (EBD), and similarly EBM (evidence-based medicine) and EBN (evidence-based nursing), is composed of two fundamental and intertwined processes: evidence-based research (EBR) that seeks to obtain the best available evidence, and evidence-based practice (EBPr) that incorporates the best available evidence into clinical intervention, and related to cost-effectiveness research analysis (CER, aka CEA)
The best available evidence that is gathered through EBR is meant to complement, not replace the set of elements that the clinician utilizes in decision-making. EBD is simply intended to formulate recommendations (cf., note 1) for decision making, and not to dictate what practitioners should or should not do.“…Rather, the EBD process is based on integrating the scientific basis for clinical care, using thorough, unbiased reviews and the best available scientific evidence at any 1 time, with clinical and patient factors to make the best possible decision(s) about appropriate health care for specific clinical circumstances. EBD relies on the role of individual professional judgment in this process…” (ADA Positions and Statements).
Undoubtedly, certain interventions in dentistry need not, or cannot be subjected to the evidence-based paradigm. Take, for example, a superficial cavity in the enamel compartment of a molar: here an aggressive restoration involving a root canal, a crown, or an implant is most likely uncalled for. By contrast, carious lesions that project proximal to the pulp chamber, will, in all likelihood require aggressive restoration. In this particular case, evidence-based dental care is most probably not needed. EBD, and evidence-based medicine (EBM) and evidence-based nursing (EBN), fundamentally incorporates into clinical decisions for treatment interventions and for updating policies a plethora of well-articulated information about:
-
The patient:
-
Dental and medical history
-
Wants and needs
-
Exam results, symptoms, X-rays, laboratory tests
-
The health care provider:
-
Training, expertise
-
Clinical judgment, experience
-
Recommendations
-
Utility concerns:
-
Risk/benefit ratio
-
Cost/benefit ratio
-
Insurance coverage/private payment
-
Best available research evidence:
-
Consensus of the best available research evidence following systematic reviews (SRs) and meta- analyses (RS process)
-
rCPGs
EBD requires the synthesis of the available research in a process that involves:
-
Framing the clinical problem as patient-intervention-comparison-outcome (PICO) question, which permits timely retrieval and critical evaluation of the available research literature, and the evaluation of validity of the integrated information.
-
The data are interpreted from the perspective of Bayesian modeling in order to obtain statistical significance, infer clinical relevance and effectiveness, and extract Markov estimates (e.g., Markov model3).
A recent superbly articulated guide to evidence-based decision making for dental professionals presented a step-by-step process for making evidence-based decisions in dental practice [19]. The model consists of five distinct levels of mastery:
-
Formulating patient-centered questions – i.e., the PICO question described above
-
Searching for the appropriate evidence – i.e., the initial step of RS
-
Critically appraising the evidence – i.e., the core of RS
-
Applying the evidence to practice – i.e., EBPr and care
-
Evaluating the process – i.e., evaluating outcomes and policies
Whereas this discussion took dentistry and EBD as a model example, it is self-evident that it applies to EBM and EBN as well. In evidence-based health care in general, the presentation and evaluation of the findings of RS in a summative evaluation model is often referred to as a systematic review [33], because of the emphasis on the systematic gathering of all of the available research evidence, and the systematic analysis of the level [45] and quality of the evidence [2, 9, 11, 14, 34, 38], based on established criteria of research methodology, design, and statistical analysis [2, 3, 33] (cf., note 2). A well-conducted systematic review produces a clear, concise, and precise consensus of the best available research evidence in direct response to the PICO question. The consensus statement permits statements of rCPGs, which in turn lead to evidence-based treatment (EBT) interventions, and evidence-based policies (EBPo) [4, 5, 7, 12] (Fig. 1.2).
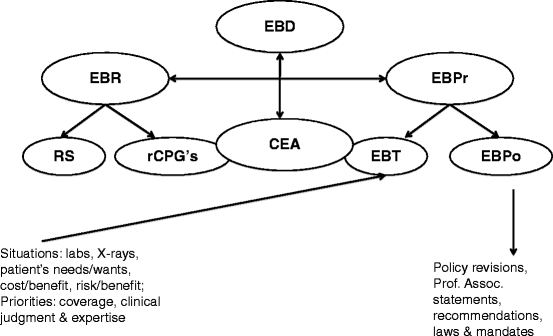
Fig. 1.2
EBR in dentistry/medicine/nursing is conducted as a process of research synthesis (RS), whose product, the systematic review generates a consensus of the best available evidence, evaluated for the level and the quality of the evidence, and analyzed by means of acceptable sampling and meta-analysis statistics. The consensus revised clinical practice guidelines (rCPGs) are incorporated into evidence-based treatment (EBT), which is duly evaluated for efficacy and effectiveness before it becomes new and improved evidence-based policy (EBPo). CEA is interdependent with rCPGs and EBT, and shown on the figure as partially overlapping
In brief, evidence-based health care rests on the consensus of the best available evidence to revise clinical practice guidelines, treatment protocols, and policies. Because the instruments and the process utilized to reach that consensus must be scrutinized, evaluated, and standardized, it is imperative that SRs be of high quality and follow a rigorous, detailed, and tested RS protocol, including that for the acceptable sampling and meta-analytical processing of the data [2, 33]. Therefore, it is important to develop and to validate standards for the evaluation of the quality and reliability of SRs and meta-analysis4.
As the EBD/M/N literature grows, multiple SRs are produced in response to any given clinical PICO question. In some instances, multiple SRs are concordant in the generated consensus statements; in other instances, discordant SRs may arise. In either instance, it is becoming increasingly important to refine RS tools to evaluate the overall evidence across multiple SRs (e.g., assessment of multiple SRs, AMSTAR; [49, 50]) for the generation of what has been termed either “complex systematic” reviews [56] or “meta-SRs” [3, 7].
1.2 Probabilistic Models for Clinical Decision Making
Clinical decisions rest on a complex admixture of facts and values. They can be made on the basis of experience in situations in which the presenting condition and patient characteristics are consistent with the findings that are associated with predictable outcomes. In this case, the dentist’s expertise helps to recognize these types of clinical situations triggered by key elements that are rapidly integrated into a mental model of diagnostic categories and an overall concept of treatment modalities. Here, treatment options derive most directly from clinical experience and judgment. They aim to meet accepted standards of care, but are rarely altered by consensus statements of the best available evidence.
By contrast, analytical decision making applies to those presenting conditions and patient characteristics that are less certain, and that require recommending treatment modalities whose benefits and harms are variable or unknown. In this context, clinical experience and judgment is insufficient in meeting accepted standards of care, and clinical decisions must be carefully pondered. Decision aids, such as the Markov tree are useful, as are quite often, recommendations that arise from the best available evidence [1].
In brief5, clinical decision-making problems often involve multiple transitions between health states. The probabilities of state transitions, or related utility values, require complex computations over time. Neither decision trees nor traditional influence diagrams offer as practical a solution as state of transition models (i.e., Markov models). Markov models represent cyclical, recursive events, whether short-term processes, and therefore are best used to model prognostic clinical cases and associated follow-up. Markov models are often used to calculate a wide variety of outcomes, including average life expectancy, expected utility, long-term costs of care, survival rate, or number of recurrences.
Discrete Markov models enumerate a finite set of mutually exclusive possible states so that, in any given time interval (called a cycle or stage), an individual member of the Markov cohort can be in only one of the states. In order to determine a value for the entire process (e.g., a net cost or life expectancy), a value (an incremental cost or utility) is assigned to each interval spent in a particular state. The assignment of value in a Markov model is called a reward, regardless of whether it refers to a cost, utility, or other attribute. A state reward refers to a value that is assigned to the members of the cohort in a particular state during a given stage. The actual values used for state rewards depend on the attribute being calculated in the model (e.g., cost, utility, or life expectancy). A simple set of initial probabilities is used to specify the distribution of model subjects among the possible state rewards at the start of the process. The resulting matrix of transition probabilities is used to specify the transitions that are possible for the members of each Markov reward state at the end of each successive stage.
Two methods are commonly used to calculate the value of a discrete Markov model: a) cohort (expected value) calculations, and b) Monte Carlo trials. In a cohort analysis, which corresponds more realistically to a clinical situation, the expected values of the process are computed by multiplying the percentage of the cohort in a reward state by the incremental value (i.e., cost or utility) assigned to that state. The outcomes are added across all state rewards and all stages. In the more theoretical Monte Carlo simulation trial, the incremental values of the series of reward states traversed by the individual are summed.
The Markov model is most often represented in a graphical form known as a cycle tree. Since it is based on a node and branch framework, it is easily integrated into standard decision tree structures and can be appended to paths in a Markov decision tree. The root node of the Markov cycle tree is called a Markov node. Each of the possible health states is listed on the branches emanating from the Markov node, with one branch for each state. Possible state transitions are graphically displayed on branches to the right. A state from which transitions are not possible, such as the Dead state, is called an absorbing state. No state rewards are given for being in the Dead state, and zero values are assigned to the state rewards of all absorbing states. In this fashion, the Markov process integrates a termination condition, or stopping rule, specified at the Markov node to determine whether a cohort analysis is complete. This rule is the termination condition at the beginning of each stage. When the termination condition is verified, the Markov process ends and the net reward(s) are reported. The termination condition can include multiple conditions, which may be cumulative or alternative.
The Markov model generates an expected value analysis that is performed at, or to the left of each Markov node in cohort analysis. The expected value analysis can generate additional information about the Markov cohort calculations. For example, in a model designed to measure the time spent in the diseased state diagnosed as dementia of the Alzheimer’s type, the expected value will generate the average life expectancy for a patient in the cohort. Additional calculated values will include the amount of time spent, on average, in each of the specified states of Alzheimer’s dementia. The percentage of the cohort in each state will be computed at the end of the process. When the termination condition has been set to continue the process until most of the cohort is absorbed into the Dead state, the final probability of patients in the Dead state will approach 1.0. In brief, one of the strongest assets of the Markov model is its capacity to yield both an extensive numerical description of the process under study, as well as a detailed graphical representation and associated costs.
From this viewpoint, cost-effectiveness analysis (CEA)6 can be performed either on the basis of expected value calculations or using Monte Carlo simulation. This is particularly important in the case of complex state transition models, because in order to evaluate individual outcomes – as distinguished from cohort analysis – Markov models must be calculated using Monte Carlo simulation.
CEA is a collection of methods for the evaluation of decisions based on two criteria using different outcome scales. It is of particular interest in situations where resource limitations require balancing the desire to maximize effectiveness and the need to contain costs.
CEA can simultaneously compare the expected costs and the expected effectiveness values of the options at a decision node. CEA generates a cost-effectiveness graph, which is interpreted as the best available evidence in support of fundamental CEA tools, calculations, results, and findings, including incremental values and the existence of dominance7 or extended dominance8.
The remainder of this discussion pertains to the circumstance of analytical decision making in health care.
Analytical decisions, generally speaking, refer to the behavioral and the cognitive processes of making rational human choices, from the logical and rational evaluation of alternatives, the probability of consequences, and the assessment and comparison of the accuracy and efficiency of each of these sets of consequences. Decision-making principles are designed to guide the decision-maker in choosing among alternatives in light of their possible consequences [1].
Decision theory has emerged principally from two schools of thought: first, the probability theory recognizes that decisions may involve conditions of certainty, risk, and uncertainty. In this model, the probability of occurrence for each consequence (i.e., utility) is quantifiable, and alternatives of occurrence are associated with a probability distribution. The correctness of decisions can be measured as the adequacy of achieving the desired objective, and by the efficiency with which the result was obtained [3, 15, 51].
The process of making a decision driven by a probabilistic estimation of either the “prospects” or the “utility” of its outcome is in effect the informed choice among the possible, probable, and predicted occurrence. Whereas decisions are most often made without advance knowledge of their consequences, three fundamental rules guide probabilistic decision making:
The prospect theory [30] rests on empirical evidence, and seeks to describe how individuals evaluate potential losses and gains, and make choices in situations where they have to decide between alternatives that involve a known or anticipated risk.
The prospect-based decision-making process invo lves two stages:
-
In the initial editing stage, possible outcomes of the decision are estimated, ranked, and evaluated heuristically.
-
In the final evaluation phase, decisions are estimated if their outcome could be quantified and computed, based on potential outcomes, gains and losses, and their respective probabilities, eval uation.
The aim of prospect-based decisions is to yield a choosing and deciding heuristic that establishes the more likely outcome in terms of having the more profitable utility. The utility perspective on decision making follows from the prospect-theoretical framework, and finds its roots in Antiquity [3] to our contemporary Peter Singer (6 July 1946), presently holding an academic appointment both at Princeton University and at University of Melbourne.
In the context of decision research, the term utility refers to a measure of perceived or real benefit, relative satisfaction from, or desirability of – such as, for example, “increase in quality of life” – a direct consequence of the utilization of goods or services, and health care intervention. It follows that certain interventions, or modifications thereof may contribute to increasing or decreasing such benefits, and therefore the utility of the said goods or services. For this specific reason, utility-based decision making rests largely on a rationale centered upon utility-maximizing behavior, rather than strictly economic constraints. That is to say, good dentistry should be driven by the intent of benefitting the patient, and of providing the best possible care at the lowest possible cost (cf., CEA), and are expressed as cost-to-benefit ratios.
The fundamental assumptions of the utility theory of decision making state that:
-
Utilities and probabilities of one alternative (or set of alternatives) should not influence another alternative.
-
Alternatives are “transitive,” and are subject to ordering based on preferences.
-
By the very nature of the process, creativity and any form of cognitive input is excluded, as it strictly rests on probabilistic rules.
As we discussed elsewhere [3], utility theory proposes to generate two types of measurable outcomes:
-
Cardinal utility, the magnitude of utility differences, as an ethically or behaviorally relevant and quantifiable measure.
-
Ordinal utility, that is utility rankings, which do not quantify the strength of preferences or benefits.
As such, utility is often described by an indifference curve, which plots the combination of commodities that an individual or a society would accept to maintain a given level of satisfaction. In that respect, individual utility (or societal utility) is expressed as the dependent variable of functions of, for instance, production or commodity9 [3].
Probability-based decision making can be driven by, and carried out for the purpose of altering utility outcomes. Nevertheless, the latter point can be particularly problematic since cognitive dissonance does arise between the outcome of the purely probabilistic process, and the clinician’s knowledge, information processing, beliefs, preferences, expertise, whether or not in the context of newly rCPGs resulting from SRs.
Cognitive and social psychologists correctly argue, however, that individuals in a social group – such as dentists, doctors, physician assistants, nurses and nurse practitioners, and patients – often have different value systems upon which to establish the utility of a given intervention. Consequently, it is unclear how cardinal and ordinal utility can be reconciled across these different perspectives. Neither does the utility theory of decision making nor its alternative that is concerned with the prospect of risk and benefits (hence, prospect theory of decision making) permit adequate normative and summative evaluation of outcome and success. Alternate decision-making theories may actually be prone to be more useful than utility or prospect theory in the context of evidence-based health care.
1.3 Logic Evidence-Based Decisions in Clinical Practice
Rather than relying on probabilistic conditions, the decision-making process may rest on cognitions and reasoning, such as either rationality or logic. In that respect, the cognition-based approach to decision making would become akin to the so-called intelligence cycle, which originated as the processing of information in the context of a civilian or military intelligence agency or in law enforcement as a closed path consisting of repeating nodes, and the closely logically related target-centric approach [3, 10]. This process of decision making is critical to the analysis of gathered intelligence, and may be summarized in certain fundamental steps, or phases, which exemplify the process of evidence-based cognitive decision making, whether it be a rational model or a logic model (vide infra):
1.
In the directive phase, the specific “intelligence question” is posed – in the preceding chapter, we indicated that the directive phase of the evidence-based process is the statement of the PICO question.
2.
In the collection phase, the data, information, processed intelligence, and corporate “wisdom” resides – in the context of evidence-based decision making, we stressed the need to integrate expertise and experience with the entire body of available evidence.
3.
In the analysis phase, the collected information is collated, analyzed, and evaluated. This is identical to the step we described above where the best evidence is obtained from the entire body of available evidence, based on the level and the quality of the evidence, followed by acceptable sampling and meta-analysis.
4.
In the dissemination phase, the processed information, data and intelligence is presented in a form that is useful, relevant, in context, and most importantly, timely. This step corresponds, for all intents and purposes, to the systematic review format of reporting the evidence-based process.
5.
In the reflection phase, the newly discovered information is incorporated into corporate wisdom, from which flows new and improved questions and tasks. The evidence-based paradigm here speaks of the dissemination of the consensus of revised guidelines.
The traditional intelligence cycle, and the rational and the logic cognition-based models of decision making, distinguish “collectors,” “processors,” and “analysts.” In a remarkable parallel, so does the evidence-based paradigm separate those who perform RS and EBR to produce the consensus for rCPGs, from those who integrate consensus statements into evidence-based treatment intervention (EBT) and practice (EBPr), and from the policy makers, who integrate normative and summative evaluations into new and improved EBPo for the benefit of the stakeholders (cf. Fig. 1.2). The remainder of this writing compares and contrasts the rational vs. the logic model of clinical decision making, and argues in support of the latter in the context of evidence-based health care.
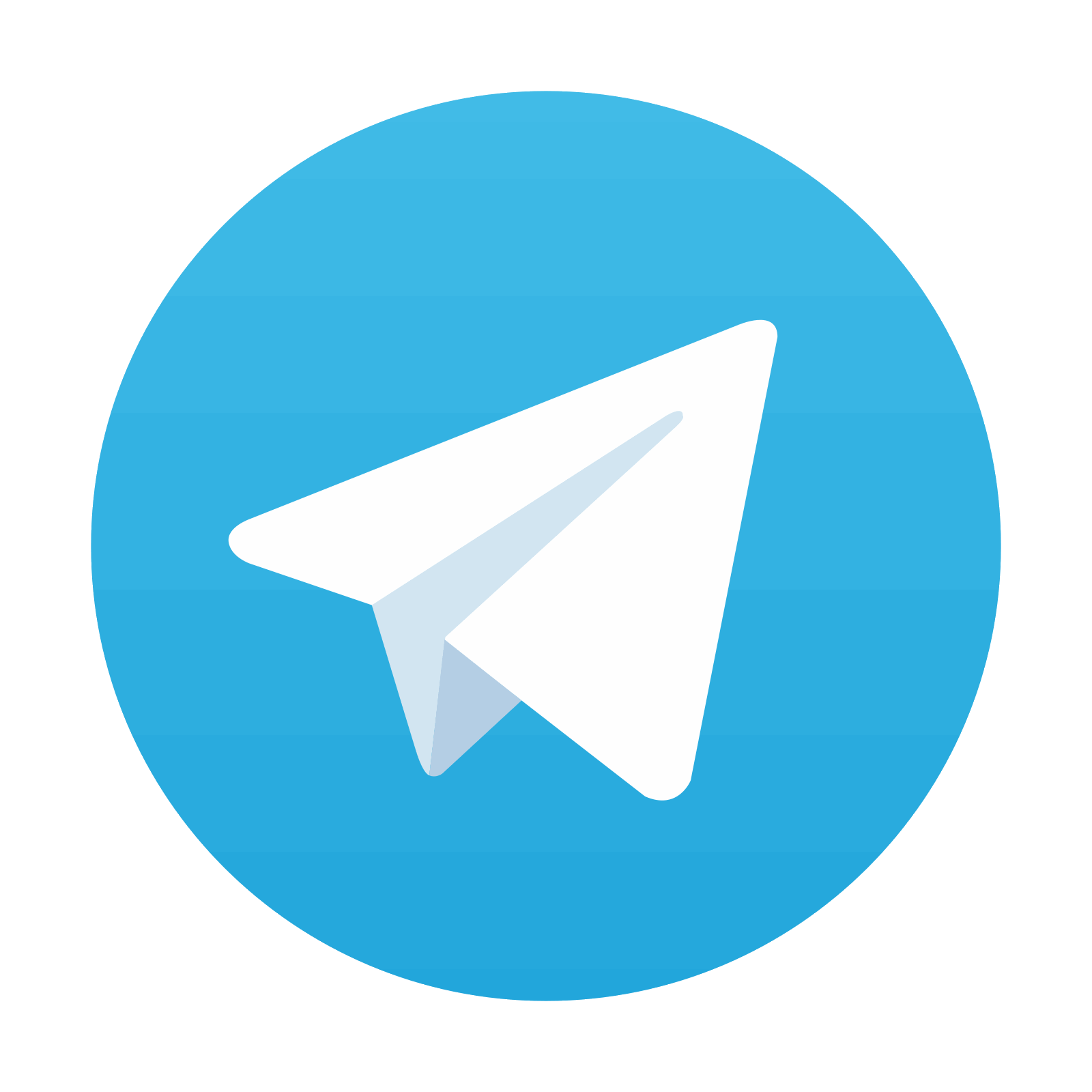
Stay updated, free dental videos. Join our Telegram channel

VIDEdental - Online dental courses
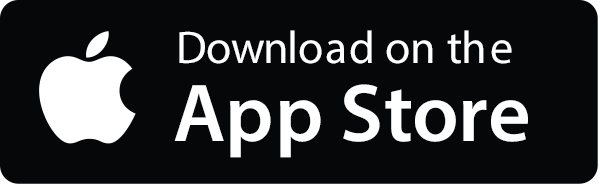
