In the previous article, I discussed the null (H 0 ) and the alternative (H a ) hypotheses, and the z and t statistics. The next step is to calculate the probability of obtaining data at least as extreme as those we have observed if the null hypothesis were true. This probability is called the P value. To obtain this probability, we calculate first the test statistic, and then we compare this with the distribution implied by the null hypothesis to obtain a probability (use tables or statistical software). In general, the more extreme the test statistic, the more unlikely the null hypothesis, and the smaller the calculated probability will be.
The general rules for interpreting P values are given in Table I .
Large P value | Small P value |
---|---|
Do not reject H 0 | Reject H 0 |
Sampling variation is a plausible explanation | Sampling variation is an unlikely explanation |
μ 1 is not significantly different from μ 0 | μ 1 is significantly different from μ 0 |
To put into practice what I discussed, we can conduct hypothesis testing in our sample of adolescent patients visiting the office and see whether the hypothesis that the mean of our office sample is equal to the hypothesized true population mean age of 15.3 years.
Please note that in the rare occasion when we know the population standard deviation, we calculate the z value using formula 1:
(Formula 1)
Two-sided z test
H 0 : μ = 15.3 vs H 1 : μ ≠ 15.3 and known standard deviation (σ of population known)
Otherwise, we should estimate the standard error as shown in Table II (SD/√n=5.78/√74=0.67)
( SD / n = 5.78 / 74 = 0.67 )
from our sample and then calculate the t statistic using formula 2:
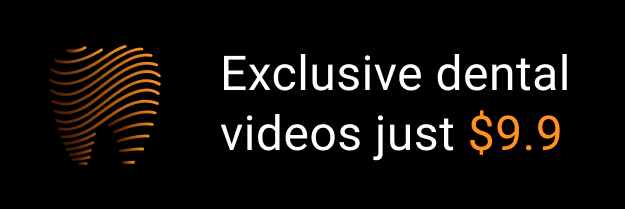