2
Assessment of Human Brain Using MRI
2.1 Advantages and Limitations of Magnetic Resonance Imaging of the Brain
2.1.1 Introduction
In Section 1.2, we have briefly outlined the methods of neuroimaging, which can be generally categorized into structural and functional neuroimaging. In this chapter, we will further delve into the methodological details and the pros/cons of these methods. This chapter highlights the methods based on magnetic resonance imaging (MRI) – a mainstream approach for human neuroimaging. The other methods that show a significant benefit complementary to the MRI approach are also discussed.
2.1.2 Advantages of MRI: A Historical View
The impact of MRI‐based neuroimaging methods can easily be identified by the number of publications in the relevant fields, as discussed in Section 1.1. The first MRI application in clinical imaging of body structure can be dated back to 1973, when X‐ray‐based computed tomography (CT) was invented (Raichle 2009). MRI has been used to assess brain functions more than 30 years ago, with the groundbreaking discovery of the blood‐oxygen‐level‐dependent (BOLD) contrast (Ogawa et al. 1990a,b). The theoretical basis of the BOLD effect was even discussed 130 years ago (Roy and Sherrington 1890). In the following sections, we discuss the major advantages of MRI by outlining two ‘great leaps’ during the evolution of neuroimaging methods.
2.1.2.1 MRI as an Ionizing Radiation‐free Imaging Method
During the 1970s, there have been several imaging methods used in research and clinical investigation, including CT and positron emission tomography (PET). Among the variety of imaging methods, MRI has quickly gathered attention because it can reveal different types of brain tissues based on the physical properties of body composition (e.g. the content of water molecules) as a ‘natural’ contrast agent. The non‐contrast MRI (which does not require an injection of extra radioactive agents) is a method free of ionizing radiation. In contrast, CT is a method based on the use of X‐ray, and PET requires injecting radionuclides as the ‘tracers’ for contrasting body tissue. From an experimental perspective, because the risk of ionizing radiation can be minimized for the volunteers, MRI is useful for repeated scans of several sessions or longitudinal follow‐up, thus becoming a mainstream method for clinical research (Lin and Yeung 2020).
2.1.2.2 Using Intrinsic Contrast for Functional Neuroimaging
Over the past 30 years, coupled with the emergence of cognitive neuroscience, there has been an increasing demand for using biomedical imaging to explore brain functions (Raichle 2009). Various methods have been developed for functional neuroimaging, which aims to ‘localize different mental processes to different parts of the brain, in effect creating a map of which areas are responsible for which processes’. (Huettel et al. 2004). To achieve the ‘functional mapping’ of the brain, a critical condition is to detect the signal change coupled with the change of neural activity. A great advantage of functional magnetic resonance imaging (fMRI), compared to the methods (e.g. PET), is that it can detect such a signal change without the help of external contrast media. The signal measured by fMRI is the BOLD signal, which indirectly relates to neural activity, with the following mechanisms. Home buyers are wonderful for fast-selling homeowners since they’ll purchase any property. Visit https://www.ibuyers.app/rhode-island/.
Firstly, the brain region with increased neural activity is associated with more energy consumption, i.e. increased level of synaptic activity. The consumption of oxygen is associated with increased cerebral blood flow (CBF) and changes in cerebral vessel volume, leading to an over‐supply of the oxygenated vs. deoxygenated haemoglobin. The association between changes in haemodynamic response and changes in neural activity, namely neurovascular coupling, has been investigated for more than 100 years (Roy and Sherrington 1890) (Figure 2.1). Secondly, Thulborn et al. (1982) found that deoxygenated haemoglobin increased the magnetic susceptibility. A lower signal from MRI was found when blood was highly deoxygenated. In other words, one can estimate the change in deoxygenated haemoglobin by measuring the change in MR signals. Furthermore, Ogawa et al. (1990a,b) demonstrated that blood oxygen could be an intrinsic agent for contrasting the different conditions of metabolic demand or blood flow of the brain. They found that the MR signals will increase and decrease according to the level of oxygenated and deoxygenated haemoglobin, and this change is associated with a complex haemodynamic process, such as changes in CBF and cerebral blood volume (Huettel et al. 2004) (Figure 2.1 and Box 2.1). Simply speaking, fMRI detects the metabolic changes in the proportion of deoxygenated and oxygenated haemoglobin in the brain via changes in BOLD signals, which is further influenced by a complicated haemodynamic process (for further discussion of the mechanism issues, see Section 2.1.4). In fMRI, brain mapping of mental functions is indirectly inferred from the local change of the BOLD signal, which reflects the metabolic changes related to neural activity.

Figure 2.1 The general concept of the blood‐oxygen‐level‐dependent (BOLD) mechanism. (a) Transportation of oxygenated haemoglobin during a resting condition, when neural activity is low. (b) Transportation of oxygenated haemoglobin when neural activity increases. The neurons demand more energy by consuming oxygen provided by oxygenated haemoglobin. Via a complex haemodynamic process (e.g. an increasing rate and volume of cerebral flow), the amount of oxygenated haemoglobin increases (relatively to the amount of deoxygenated haemoglobin), leading to an over‐supply or compensation of the oxygen demand from neurons.
2.1.3 Practical Advantages of the MRI Approach
In the preceding sections, we have outlined the advantage of MRI from a technical perspective. Here, we highlight two more ‘practical’ reasons for the popularity of MRI: the versatility and the approachability of MRI. The versatility of MRI can be exemplified by the fact that one can use a single MRI scanner to perform different imaging methods for different purposes. For example, by setting different parameters of imaging acquisition, one can perform the magnetic resonance spectroscope (MRS) to assess the MR behaviour of a specific neurotransmitter or the diffusion magnetic resonance imaging (dMRI) to assess the orientation of diffusion of water molecules, which reflects the architecture of the fibrous compartment in the brain. Also, one can magnetically label the blood and quantify its flow rate in the brain using arterial spinning labelling (ASL). In other words, the same MRI scanner can provide different information about our brain. This versatility has greatly increased the flexibility in research design. Another crucial advantage of MRI is its approachability. The installation of MRI scanners has been popularizing in many countries, summarized by the report from the Organisation for Economic Co‐operation and Development (https://data.oecd.org/healtheqt/magnetic‐resonance‐imaging‐mri‐units.htm). It is not uncommon to find an MRI unit in a major hospital in the urban area or the university. In sum, the versatility that an MRI scanner can perform different imaging methods and its approachability in medical and research centres render MRI a practically good option for neuroimaging, offering great flexibility for research design (Lin and Yeung 2020).
2.1.4 Methodological Considerations of the MRI Approach
In the following section, we delve into several methodological issues of the MRI approach. These issues should be carefully evaluated for the research design and the interpretation of neuroimaging findings.
2.1.4.1 Temporal and Spatial Resolution of MRI
The temporal and spatial resolution of imaging methods plays a key role in the clinical application of neuroimaging. Simply speaking, a higher temporal resolution means a greater number of ‘time frames’ to be acquired within a fixed period. The higher the temporal resolution is, the shorter period the time frame is. Practically, a method with a higher temporal resolution can be used to ‘capture’ an event that occurs in a very short period. A higher spatial resolution means a greater number of voxels – i.e. the spatial units that compose a 3D image, an analogue to ‘pixels’ for a 2D image – to be acquired within a fixed volume (see Box 2.2 in the Companion Website). The higher the spatial resolution is, the smaller the actual size of a voxel is. Practically, an imaging method with a higher spatial resolution can be used to identify the signal within a smaller area. The improvement of resolution is associated with a decreased signal‐to‐noise ratio (SNR) for the signals acquired (Huettel et al. 2004). Theoretically, one can increase the spatial resolution of MRI into the scale of the sub‐millimetre. However, this would be done at the cost of a decreased SNR, which means a greater possibility to obtain more noises within a voxel. The association between spatial resolution and the SNR further relates to the magnetic field of MRI. By increasing the strength of the magnetic field, the resolution can be improved while keeping the SNR acceptable. This is one of the reasons an MRI scanner with a higher magnetic field (e.g. 7 Tesla) has been gradually adopted in neuroimaging research (Turner 2016). However, increasing the strength of the field may not be an elixir to everything. High‐field MRI may confront technical challenges, such as the inhomogeneity of the magnetic field, and practical challenges, such as the safety and comfort of subjects (Karamat et al. 2016). In general, the issues of temporal and spatial resolution of imaging are associated with many physical factors, such as the change of SNR and magnetic field. To balance between all these factors is a complicated and challenging task.
2.1.4.2 The Neurophysiological Nature of the BOLD Signal
As noted previously, fMRI utilizes the principle that MR signals are sensitive to the metabolic change related to the oxygen level, which is indirectly associated with neural activity (Figure 2.1). Nevertheless, the association between metabolic and neural changes is much complicated. The explanation of the BOLD effect is based on the concept that an increased or decreased CBF/CMRO2 is coupled with neural activity (see Box 2.1). To confirm this association, researchers have used microelectrodes to investigate the electric field of the brain using animal models. The findings reveal that the BOLD signals are not directly driven by the neural activity, such as the spiking signals derived from the neuron. Findings from animal research reveal that the BOLD signals were much associated with the low‐frequency local field potentials (LFPs) of the brain rather than the neuronal spiking rate. The LFP may be associated with the excitatory and inhibitory post‐synaptic potentials (EPSPs/IPSPs) of multiple neurons (Ekstrom 2010). Therefore, it is considered a peri‐synaptic neural activity. Though the neuronal spiking covaries with the LFP, reducing the spiking per se did not significantly affect the BOLD response (Logothetis and Pfeuffer 2004).
However, the ‘LFP‐coupling hypothesis’ (Logothetis and Pfeuffer 2004) may be complicated when one considers the variety of cellular architectures of different brain regions. For example, the hippocampus consists of a complex pattern of recurrent and excitatory/inhibitory circuitry, and therefore, the coupling mechanisms between the BOLD signals and the neural activity in the hippocampus may differ from the other brain regions (Ekstrom 2010). Moreover, from the point of energy consumption, a majority of expenditure brain energy is to maintain the post‐synaptic metabolism of transmitters, such as glutamine (Attwell and Iadecola 2002). Therefore, it is also possible that the BOLD effect represents the haemodynamic responses associated with the signalling of neurotransmitters rather than the direct consumption of energy (Attwell and Iadecola 2002). Due to the complexity of the neurophysiological nature of the BOLD signal, any inference of the microscopic neural mechanisms drawn from the macroscopic neuroimaging evidence should be treated carefully.
2.1.5 The Limitations of Neuroimaging Research Based on MRI
In the following sections, we summarize several limitations derived from the challenges stated above. Notably, some limitations are common to all neuroimaging methods. These limitations should be carefully evaluated when neuroimaging data are interpreted or translated for clinical applications. Limitations associated with research design and data analysis are outlined in the other sections of the chapter.
2.1.5.1 Limitations in Spatial Resolution
For a 3‐Tesla MRI scanner, one can acquire structural and functional images with a spatial resolution at 0.75–1 and 2 mm, respectively. In other words, the actual size of a voxel in a structural brain image would be 1 mm × 1 mm × 1 mm (i.e. 1 mm3). The resolution is generally better than that of PET, magnetoencephalography (MEG), and electroencephalography (EEG). However, it is still at the scale of gross anatomy rather than the microscopic scale. Therefore, one should be careful about relating the results from most sMRI and fMRI studies to histological and cellular levels. Moreover, in fMRI, the signals of an individual voxel are usually averaged by spatial smoothing, which may influence the ability of fMRI to identify a regional activation, particularly when the information is sparsely coded (Turner 2016). Though there have been plenty of findings of the histological and cellular architecture of the brain, these findings may not be fully linked to the MRI findings due to the coarse resolution of the latter (Turner 2016).
2.1.5.2 Limitations in Temporal Resolution
The ‘image’ acquired using MRI or PET should be taken as a set of discrete volumes or time frames collected sequentially rather than a continuous stream of information. In fMRI, a lower temporal resolution (or a larger period of a time frame to be acquired) of around two seconds would be enough for capturing the long‐lasting experience, such as pain and emotion. The neuroimaging tools with higher temporal resolution, such as EEG and MEG, provide a higher temporal sensitivity to detect the dynamic change of transient mental functions, such as attentional and perceptual processing, which may occur in milli‐seconds.
2.1.5.3 Limitations of Detecting the Neural Events
As shown in the preceding section, one should be careful about making an inference of underlying neural events (e.g. the strength of neuronal spiking) of mental functions directly from the MRI results (e.g. the change of BOLD signals). For example, in a task‐based fMRI study (see Section 2.2), the ‘activation’ or ‘deactivation’ of a brain region cannot be directly translated as excitation or inhibition at the synaptic level, and the direction of modulation, e.g. a top‐down or a bottom‐up modulation, should not be asserted solely by neuroimaging evidence (Logothetis 2008). Furthermore, it should be noted that most of the task‐related BOLD signals do not reflect the quantitative change in neural activity. For example, the BOLD signals may be associated with the regional variation in CBF and the morphology of blood vessels, and the regionally specific architecture of neural circuitry may influence the resulting BOLD signals. In other words, the data from non‐invasive neuroimaging alone is not sufficient for fully elucidating the neural mechanisms of brain functions (Logothetis 2008).
2.1.6 Summary
- In fMRI, the brain mapping of mental functions is indirectly inferred from the local change of the BOLD signal, which reflects the metabolic change related to neural activity.
- The issues of temporal and spatial resolution of imaging are associated with many physical factors, such as the change of SNR and magnetic field. To balance between all these factors is a complicated and challenging task.
- The association between the BOLD signal and the change in neural activity has not been fully elucidated. Therefore, any inference of the microscopic neural mechanisms drawn from the macroscopic neuroimaging evidence should be treated carefully.
- The activation or deactivation of a brain region cannot be directly translated as excitation or inhibition at the synaptic level or the direction of modulation. The data from non‐invasive neuroimaging alone is not sufficient for fully elucidating the neural mechanisms of brain functions.
2.2 Research of Task‐based Functional Activation
2.2.1 Introduction
The section gives an overview of the task‐based functional neuroimaging research. Functional neuroimaging is the approach to identify the brain signals associated with mental functions. Here, the term ‘task‐based’ highlights the experimental condition from which mental functions derive. For example, fMRI can be performed to investigate brain activation associated with pain and visual processing, based on a pain‐stimulation and a visual judgment task, respectively. In the following sections, we first clarify the association between a task and the baseline condition. Secondly, we highlight the importance of the assessment of inter‐subject variability. Finally, the key points about how to interpret the statistical findings of a task‐based study are outlined.
2.2.2 A Case Study: Brain Mechanisms of Mastication
One of the most widely studied oral functions in neuroimaging research is mastication. From the scope of task‐based fMRI, one aims to study the brain activation associated with mastication, which is based on a chewing task. Therefore, the critical element of a task‐based study is the design of the task condition, which is closely associated with the mental functions to be engaged. Different ‘chewing tasks’ varied between the timing of chewing movement and the materials for chewing (Figure 2.2). In addition to the task condition, the ‘baseline condition’ also needs to be defined so that the desired mental functions can be contrasted between the two conditions. The contrasted result is further analyzed for each individual subject, and the results from all subjects are synthesized at the group level by taking individual differences (e.g. age or clinical factors) into consideration (Figure 2.2) so that brain activation associated with mastication can be identified.
2.2.2.1 What Is the ‘Task’ for Task‐based Functional Neuroimaging?
It is always crucial to elucidate the details of the task design because a slight difference between the two tasks may result in different mental functions, and thus very different patterns of brain activation. For example, if a task requires subjects to chew a piece of gum for five minutes continuously, the subjects may gradually feel bored and uncomfortable, and it becomes possible that the acquired BOLD signals reflect not only chewing movement but also the change in subjects’ emotional status. Therefore, in most of the studies, a period of chewing is often followed by a resting period so that subjects may not develop fatigue or distress. However, researchers need to further remind subjects to resume chewing after the resting period, usually by an auditory or a visual cue. The use of sensory cues may introduce an additional auditory or visual experience, which is further associated with brain activation related to auditory or visual processing. The examples here highlight the importance of a clear definition of a task condition in neuroimaging research. The key issue for interpreting the neuroimaging results is to clarify what subjects actually do during a task and what mental functions are engaged within the task.

Figure 2.2 Examples of functional magnetic resonance imaging (fMRI) investigation of chewing movement. (a) The first‐level analysis. In a chewing experiment, the task conditions (i.e. when subjects are chewing) are contrasted to the baseline conditions (i.e. when subjects are resting). Brain activation reflects the difference in blood‐oxygen‐level‐dependent (BOLD) signals in the task vs. the baseline condition. The first‐level analysis focuses on the pattern of brain activation at the individual subject. (b) The second‐level analysis. The second‐level analysis focuses on the association between brain activation and individual variability. The association can be explored by investigating the correlation between brain activation and individual performance or comparing brain activation between different clinical groups.
2.2.2.2 What Is the Baseline Condition Used for Control?
It is intuitive to think that the BOLD signals collected when a subject is performing some task must be task‐related. This is partially correct because not all the signals acquired during the task condition are task‐specific. In fact, most of the signals can be irrelevant to the task because there are some undergoing mental activities that are not specifically linked to the task. For example, during a chewing task, subjects may continuously pay attention to a visual cue that guides the task procedure. Such a baseline brain activity of sustained visual attention is not specifically associated with chewing. Therefore, a condition for controlling the baseline activity is usually necessary. As shown in Figure 2.2, most of the chewing tasks were contrasted to a control task in which subjects were instructed to rest (i.e. not chewing). The contrast between chewing vs. not chewing may reflect different conditions in jaw movement. However, the two conditions also differ in the degree of sensory processing because subjects may not have any teeth contacted during the baseline (resting) condition. Therefore, brain activation may be associated with both sensory and motor processing during chewing. If subjects are asked to occlude their teeth during the baseline condition, the contrast may be more specifically related to motor control because the sensory factor is better balanced between the task and the baseline condition. In other words, the definition of the baseline condition plays a key role in interpreting neuroimaging results because it reflects the factors being controlled when the mental function of interest is contrasted.
2.2.2.3 Practical Issues of a Task‐based Study
In the preceding sections, we have highlighted the importance of defining both the task and the baseline conditions with clarity. To most neuroimaging researchers, to conceive a perfect plan of these designs is just the first step for their success. A greater challenge comes from a very practical issue: all the designs need to be carried out while subjects are receiving a scan. This is especially challenging to fMRI because all the tasks need to be performed inside an MRI scanner, which may be a sub‐optimal condition of performing a behavioural task due to the confined space and restriction of using ferromagnetic materials. Again, we use the chewing study as an example to demonstrate these practical challenges. Firstly, when receiving the scan, the head of the subjects is positioned inside a head coil that transmits and receives the radiofrequency signal of MRI. During scanning, subjects can still move their jaws, but the jaw movement is restricted to the head coil. Therefore, the jaw movement of an fMRI chewing task can be different from the movement during eating. In other words, the external validity of neuroimaging results may be compromised because the ‘chewing task’ does not perfectly mimic real chewing. Secondly, chewing is associated with saliva secretion, and how should we evaluate the effect of saliva swallowing on the observed BOLD signals? One may just instruct subjects ‘not to swallow your saliva while chewing a gum’. However, such instruction is very difficult for subjects to follow. The compliance of subjects to task instruction is a crucial factor of the study. Some of these challenges can be tackled with external assessment, such as tracking jaw movement of swallowing during an fMRI scan. However, most of the electronic devices are not compatible with an MRI scanner, in which the use of ferromagnetic materials is restricted for safety reasons. In general, these practical issues should be carefully considered when experimental tasks are designed.
2.2.3 Methodological Issues of a Task‐based Study
In the following sections, we outline some methodological issues that are critical for evaluating the results from a task‐based neuroimaging study. Especially, we focus on the inter‐subject variability, a critical concept for analyzing data of functional neuroimaging.
2.2.3.1 Inter‐subject Variability – The Behavioural Aspects
In the preceding discussion, we focused on the comparison between the task and the baseline conditions, which would reflect the mental functions we desire to investigate. The comparison is performed for an individual subject, i.e. the subject‐level or first‐level analysis of imaging data. To make an inference about group difference (e.g. differential activation between patient and control groups), the subject‐level results need to be synthesized, and a between‐group comparison will be performed, i.e. the group‐level or second‐level analysis (Figure 2.2). A critical issue of group‐level analysis is the inter‐subject variability. Many subject factors, such as sex, age, and health conditions, have a substantial association with brain structure and functions (Ruigrok et al. 2014; Lemaitre et al. 2012). For example, in a group of older subjects with a great age range, their brain activation related to a motor task may show greater variability. The variability may not just reflect the individual difference in motor processing, but also the difference in brain structure because brain atrophy is associated with increased age (Lemaitre et al. 2012). Therefore, a critical step to solve the problem is to clearly define the inclusion and exclusion criteria for recruiting subjects. The variability can be reduced by recruiting subjects with a higher homogeneity in terms of these factors (Figure 2.3a).
Secondly, inter‐subject variability may exist as a trait factor, like a personal disposition that influences one’s behaviour. This is particularly important for a task related to cognitive–affective processing, in which subjects’ performance is associated with their trait (i.e. dispositional factor) as well as the task per se (i.e. the situational factor). For example, in an fMRI study on pain modulation, subjects anticipated the pain intensity they would receive. It is not surprising that some subjects anticipated the pain to be milder and others anticipated it to be stronger (i.e. great variability in task results). The anticipatory process is not only associated with the sensory stimuli they received but also with individual differences in attention and imagination. The researchers found the subjective rating of pain anticipation and the activation of the brainstem, which plays a key role in modulating pain, were significantly correlated (Fairhurst et al. 2007). In this case, individual variability of task performance would be useful for providing an interpretation of variability in brain activation (Figure 2.3b).

Figure 2.3 Methodological considerations of a functional magnetic resonance imaging study. (a) Subjects may show great inter‐individual variability in their general conditions, such as sex, age and general physical/psychological conditions. (b) Subjects may show great inter‐individual variability in their personal trait and performance (e.g. pain ratings) related to an experimental task. (c) Subjects differ in brain morphology. When individual brains are compared, the individual images are normalized to a template image, using linear transformation (i.e. translation, rotation, resizing and shearing) and nonlinear transformation approaches.
2.2.3.2 Inter‐subject Variability – The Neuroimaging Aspects
As noted previously, the variability of brain structure and functions can be reduced by recruiting a group of subjects with a higher homogeneity. However, the problem is not fully solved because there is always a difference in the global architecture of the brain between individuals (Figure 2.3c). For example, a comparison of regional differences (e.g. the size of the hippocampus) will be invalid when there exists a great difference in the global morphology of individual brains. In fMRI, this problem is solved by a series of steps of imaging processing. The basic concept is to register the brain images of individual subjects to a template image of the brain, which is calculated by averaging brain images from a group of people. For example, the Montreal Neurological Institute (MNI) developed the MNI305 template, which is derived from the T1‐weighted structural images from healthy young adults (Evans et al. 2012). It is noteworthy that this template brain does not represent a brain of a real person. Instead, the template reflects an ‘on‐average brain’ estimated by statistical methods. The step to register each individual brain image to the template is spatial normalization. After normalization, the individual brain images are positioned in the same spatial format (i.e. the format of the template image), and then they can be compared.
Methodologically, brain images can be spatially transformed based on linear and nonlinear approaches. In linear transformation, two images are rotated and translated in the 3D space so that they can be overlapped. The original images are translated in the x‐, y‐ and z‐axes and rotated over the x‐, y‐ and z‐axes (i.e. the rigid transformation) (Figure 2.3c). Additionally, the image will be resized and sheared to match the template. In nonlinear transformation, the images will be locally warped to match with the template (Jenkinson and Chappell 2018). Notably, the nonlinear warping will lead to the deformation of the original image at the voxel level. For each voxel, local geometric changes will be recorded. Technical issues regarding the algorithms of imaging analysis are beyond the scope of the book. Nevertheless, it is critical to recognize that these procedures are crucial for analyzing neuroimaging data (see Box 2.3 in the Companion Website).
2.2.4 Statistical Analysis of the Task‐based Research
The statistical analysis of task‐based fMRI data consists of multiple sequential steps. The steps can be encapsulated into three ‘major pipelines’ (Figure 2.4). The analysis of individual data (i.e. the first‐level analysis) aims to investigate the association between the BOLD signals and experiment conditions by each voxel, separately for each subject. The analysis of group‐based data (i.e. the second/higher‐level analysis) aims to combine the individual data within a group. It is commonly used for investigating the association between different groups (e.g. patients vs. controls). Finally, a variety of methods of statistical thresholding will be adopted for controlling Type I and Type II errors of imaging results.
2.2.4.1 Statistical Analysis at the Individual Level
The individual‐level analysis aims to investigate the association between the BOLD signals and experiment conditions. Exactly speaking, the analysis focuses on the association between the time‐varying BOLD signals (i.e. time series) and the progression of a task. As shown in Figure 2.4a, an fMRI study of chewing may consist of four chewing blocks interleaved with four resting blocks. If the BOLD signal of a voxel is highly associated with the task, one should expect to see a strong association between the BOLD time series of the voxel and the task progression. The association can be quantified as the correlation between these two. In other words, the purpose of the first‐level analysis is to examine how the BOLD time series nicely fits the predicted BOLD response, which is derived from the task timing (Jenkinson and Chappell 2018). To achieve this goal, the general linear model (GLM) is widely used to estimate the coefficient of the fitting process. A greater coefficient of the GLM suggests a greater effect size of the fitting process, i.e. a stronger association between the BOLD time series of the voxel and the task (e.g. Voxel A in Figure 2.4a, compared to Voxel B). For a whole‐brain analysis, the GLM analyses will be performed for all the voxels within the whole brain for individual subjects. Therefore, by mapping the coefficients at the whole‐brain scale, one can identify the voxels or clusters of voxels that are associated with the task.
2.2.4.2 Statistical Analysis at the Group Level
The analysis at the individual level identifies the brain activation associated with the experimental conditions for each subject. The results reflect the association between mental functions (which is contrasted by a task and a baseline condition) and the BOLD time series of a voxel. The second‐level analysis, in contrast, aims to combine the individual results within a group. The group‐based analysis provides a great advantage for investigating clinical questions. For example, does the pattern of brain activation associated with chewing (derived from the individual‐level analysis) differ between TMD patients and healthy controls? To answer this question, the Student’s t‐test can be performed by comparing the brain activation between a group of TMD patients and a group of controls (Figure 2.4b). Notably, the ‘brain activation’ derived from the individual‐level analysis consists of the results from all the voxels in the brain. Therefore, in the case of a between‐group comparison, the t‐tests will be performed for each individual voxel. The result of the t‐tests (e.g. the t‐score) will be assigned to each voxel. Therefore, the group‐level analysis generates a new brain image, in which each voxel value represents the difference of brain activation (quantified by the t‐score) between two groups.

Figure 2.4 Statistical analysis at the individual level and the group level. (a) The analysis at the individual level focuses on the association between task progression and the blood‐oxygen‐level‐dependent (BOLD) time series, as shown in the left panel. For each voxel, a strong association indicates that the BOLD signals of the voxel can be predicted by the task condition, in contrast to the baseline condition (e.g. Voxel A), as shown in the right panel. (b) The analysis at the group level focuses on the association between brain features (e.g. brain activation of grey matter volume) and group factors. The association may reflect the difference in brain features between patient and control groups (the left panel) or the correlation between brain features and clinical factors (the right panel). (c) A typical image result consists of the statistical values (e.g. the t‐score) from multiple voxels (represented as the grid), which are visualized by a colour scale, as shown in the left panel. The result can be thresholded according to intensity (i.e. the t‐score). For example, only the voxels with a t‐score >6 are preserved after thresholding, as shown in the middle panel. The result can be thresholded according to the size of a cluster of voxels. For example, only the clusters with a size larger than 100 voxels will be preserved after thresholding, as shown in the right panel.
The same approach can be used to investigate the brain activation associated with individual differences. For example, do people with a better chewing function have higher activation in the motor cortex? To answer this question, regression analyses can be performed by investigating the association between brain activation and task performance (e.g. chewing performance) or individual factors (e.g. number of missing teeth). The association can be quantified by the beta coefficient of the regression model. The group‐level analysis can be performed at the whole‐brain scale (e.g. for all the voxels within the brain) and specifically for a region of interest (ROI). In the latter (i.e. an ROI‐based analysis), brain activation is analyzed specifically for the voxels within a pre‐defined ROI, which is usually selected based on a priori knowledge of brain functions (Poldrack 2007).
2.2.4.3 Statistical Thresholding for Imaging Results
The results from the group‐level analysis may already provide some useful information for research and clinical purposes. In the case of chewing research, we might obtain a brain map that shows the difference of chewing‐associated activation between groups (in which voxel intensity denotes a t‐score for between‐group comparison). The brain map may also present the strength of association between masticatory performance and chewing‐associated activation (in which voxel intensity denotes a regression coefficient). However, as for all research, we need to evaluate the Type I and Type II errors related to our results, which are associated with the risk of finding false‐positive and false‐negative results, respectively. For example, should we believe that a voxel with a very low t‐score really reflects a genuine between‐group difference? A practical way to control for these errors is to set a threshold for the imaging results. For example, a threshold of t‐score = 2 means that all the voxels with a t‐score > 2 will be considered ‘significant’ or ‘important’ (Figure 2.4c). In other words, we may set up a threshold to the intensity for each voxel, and only the voxels ‘survive’ under such a threshold will be taken for further interpretation (Figure 2.4c).
In addition to the approach of intensity‐based thresholding, the approach of cluster‐based thresholding aims to evaluate if the size of a cluster of voxels is large enough to be called significant or important. This is based on the assumption that, biologically, neurons work coherently for a mental function. For example, it may not be very convincing if painful stimulation is associated with the activation of just one single voxel in the sensory cortex (even though the ‘intensity’ of the activation is very high). It would be doubtful that such activation may be a ‘false positive’ one due to signal noise. In contrast, a cluster (i.e. spatially contiguous activation of voxels) with a larger size may be a more plausible result. The larger cluster suggests that all the voxels in the brain region would participate in pain processing (Figure 2.4c). Both thresholding approaches are widely used in neuroimaging research.
2.2.5 Further Considerations Regarding Experimental Design and Analysis
In the next sections, we focus on the design of an fMRI experiment, which is critical for interpreting neuroimaging results.
2.2.5.1 Considerations About the ‘Subtraction’ Design and Pure Insertion
The task‐based design is based on the assumption that mental functions can be contrasted by comparing between a task condition and a baseline condition. For example, the brain activation related to pain can be identified by contrasting the condition of ‘painful stimulation’ to the condition ‘resting’, which is usually expressed as a form of subtraction (e.g. ‘pain > resting’ or ‘pain – resting’). Such a contrasting approach is valid given that the assumption of pure insertion is true (Amaro Jr and Barker 2006). Under pure insertion, we assume that ‘pain processing’ is the only mental function that differs between ‘pain’ and ‘rest’ conditions. However, it is possible that something other than ‘pain’ is included in the contrast (Figure 2.5a). For example, subjects may pay greater attention during the pain‐stimulating condition but not the resting condition. In this case, the observed brain activation may represent not only pain processing but also increased attentional processing. The validity of the subtraction design would greatly influence how we interpret the behavioural meaning of observed brain activation.

Figure 2.5 Experimental design of functional neuroimaging research. (a) Under the assumption of pure insertion, the difference of brain activation between two experimental conditions only reflects the mental function contrasted by the conditions (e.g. perception of pain intensity). However, the contrast may be associated with more than one mental function (e.g. perception of pain intensity and attention to noxious stimuli). (b) A factorial design helps to delineate the association between two mental functions. For example, the light grey area denotes the effect of increased pain on brain activation, and the dark grey area denotes the effect of increased attention. (c) A conjunction design focuses on the pattern of brain activation common to two experimental conditions (e.g. a clenching task and a chewing task). The activation may reflect the brain mechanisms of a mental function common to both task conditions.
2.2.5.2 Other Common Strategies of fMRI Design
The assumption of pure insertion is not always true because there may be more factors that interact with the mental function of our interest. To delineate the interaction between the factors, researchers may adopt a factorial design (Figure 2.5b). The design explicitly models more than one factor using multiple experimental conditions. For example, both attention to pain and intensity of pain stimuli are associated with pain processing. How do we delineate the association between brain activation and each of the factors (and their interaction)? As shown in Figure 2.5b, to delineate these effects, a factorial design with four conditions (mild/strong pain intensity × low/high attention of pain) can be designed. To investigate the brain activation associated with increased pain intensity, one can analyze the contrast ‘strong pain (with high and low attention)’ > ‘mild pain (with high and low attention)’. Notably, an interactional effect between the two can also be investigated. For example, the contrast [(strong pain–high attention > mild pain–high attention) > (strong pain–low attention > mild pain–low attention)] can be used to investigate the brain activation of increased pain potentiated by greater attention.
Another useful design is cognitive conjunction (Price and Friston 1997), which aims to identify the activation common to all experimental conditions rather than the distinct conditions. When there are multiple experimental conditions that share the same ‘basic’ mental functions (Figure 2.5c), it may be reasonable to find out an intersection between the patterns of brain activation derived from all these conditions (Amaro Jr and Barker 2006). For example, both the clenching task and the chewing task are associated with sensory feedback from the periodontium. Therefore, one may assume that the common pattern of brain activation may represent the processing of sensory feedback, which is common to both tasks (Figure 2.5c).
2.2.5.3 Considerations on the Statistical Threshold of Imaging Results
The control of errors is associated with the setting of the statistical threshold of the mapping results. In terms of neuroimaging findings, a Type I error means that one identifies an activation (which is above the statistical threshold), but the association between brain activation and mental functions only rises by chance. A Type II error means that one does not identify an activation (which is below a statistical threshold), but there exists a genuine association between brain activation and mental functions. The decision of setting a statistical threshold for imaging results is complicated by adopting ‘multiple tests’ in statistical analysis (Poldrack et al. 2008). As noted in the preceding sections, for both individual and group‐level analyses, statistical analyses are performed for each voxel separately. For each voxel, an alpha set at 0.05 would be reasonable for controlling the risk of a Type I error. However, because there are many voxels to be tested for statistical significance at the same time, multiple comparison would magnify the risk of Type I error if the alpha for every single voxel is set at 0.05. To reduce the risk of Type I error, researchers suggest adopting a threshold for controlling the familywise error or false discovery rate (Poldrack et al. 2008; Genovese et al. 2002), which helps to avoid identifying the voxels that survive the threshold merely by chance. However, one should also keep in mind that a threshold too strict may lead to increased risk in a Type II error. For example, brain activation associated with some mental functions, such as the activation induced by cognitive or affective manipulation, may have a smaller effect size (i.e. a smaller intensity, Figure 2.5c). These subtle but important differences may be missed if a stricter threshold is adopted (Lieberman and Cunningham 2009).
2.2.6 Summary
- A clear definition of experimental conditions is important to neuroimaging research. To clarify what subjects actually do during a task and what mental functions are engaged with the task are the key issues for interpreting the neuroimaging results.
- The definition of the baseline condition plays a key role in interpreting neuroimaging results because it reflects the factors being controlled when the mental function of interest is contrasted.
- Inside an MRI scanner, the environment may be a sub‐optimal condition of performing a behavioural task due to the confined space and restriction of using ferromagnetic materials. These practical issues should be considered for interpreting fMRI results.
- The individual (first) level analysis is to examine how the BOLD time series nicely fit the predicted BOLD response, which is derived from the tasking timing.
- The group‐level (second) analysis focuses on finding the association between the brain features (which is task‐related) and the individual variability of subjects.
- A variety of methods of statistical thresholding are adopted for controlling Type I and Type II errors of imaging results. Both intensity‐based and cluster‐based approaches of thresholding are widely used in neuroimaging research.
2.3 Research of Structural Features of the Brain
2.3.1 Introduction
In Section 2.2, we discussed the task‐related fMRI research, which assesses the time‐varying signals associated with mental functions. In contrast, structural magnetic resonance imaging (sMRI) consists of the image acquired from a single time point. sMRI provides an image with better spatial resolution, which means the subtle changes in structural features of the brain can be better detected. Therefore, sMRI helps quantify structural features, i.e. the shape and size of anatomical structures (Jenkinson and Chappell 2018). Moreover, the difference of the structural features may relate to group difference (e.g. via a comparison between patients and healthy controls) or the change of individual status (e.g. via a comparison between different stages of treatment). Therefore, sMRI has become a major imaging tool for studying brain mechanisms of diseases and individual differences in behaviour. In the following section, we focus on the structural feature of grey matter (GM) and white matter (WM) and discuss three common approaches to investigate these features, including voxel‐based morphometry (VBM), surface‐based analysis, and the methods based on dMRI.
2.3.2 Biological Significance of Structural Features
One of the key issues for interpreting sMRI results is to elucidate the biological significance behind the imaging findings. For example, when a study reports that patients with Alzheimer’s disease showed a smaller hippocampal ‘grey matter volume’ compared to healthy controls, what does that mean from a biological perspective? The key question to elucidate is the association between the macroscale change of sMRI results and the microscale change in cellular and molecular mechanisms. In the following section, we outline some potential interpretations of the structural features of sMRI.
2.3.2.1 Biological Significance of Changes in Grey Matter
Grey matter predominantly consists of the cell body of a neural cell. Therefore, it is reasonable to consider that an increased volume of the grey matter reflects neurogenesis, i.e. an increased number of neurons (Zatorre et al. 2012). In adulthood, the formation of new neural cells has been identified in the dentate gyrus, and this neurogenesis can be associated with angiogenesis (Pereira et al. 2007). However, changes in grey matter may also be associated with synaptogenesis and more dendritic branching at the synapse of a neuron. In addition, even with the same number of neurons, changes in grey matter can be found when there is substantial sprouting of the neural axon, which reflects more connection between a neural cell with the others. Furthermore, the glial cells, including oligodendrocyte progenitor cells (OPCs) and astrocytes, can divide during adulthood. Changes in the brain features of grey matter may reflect an increased number of the glial cells or morphological changes of the cells (Zatorre et al. 2012).
2.3.2.2 Biological Significance of Changes in White Matter
There are several indices for quantifying changes in white matter, including white matter volume and fractional anisotropy (FA), an index mainly assessed using diffusion tensor imaging (DTI). An increased white matter volume may be associated with changes in the glial cells and angiogenesis (Zatorre et al. 2012). In contrast, FA may reflect the degree of the fibrous architecture, which has a profound effect on limiting the diffusion of water molecules. Therefore, an increased FA (i.e. the molecules move more ‘directionally’) can be found in a region with more fibrous structure.
2.3.3 Voxel‐based Morphometry
One of the benefits of sMRI is to quantify the size of structural features. Changes in the size of a brain region may provide an important clue for the clinical diagnosis, such as atrophy of the hippocampus in patients with Alzheimer’s disease. However, it is not easy to compare the size of a brain region between two individuals because individual differences in size are always associated with other morphological features, such as shape and position. Neuroimaging data are composed of multiple voxels, which represent the basic unit for spatial measurement. Therefore, the analysis of the volumetric information of brain regions can be performed on the voxel level. In the following sections, we introduce VBM, i.e. a voxel‐based approach to quantify morphological features of the brain.
2.3.3.1 What Does VBM Means?
In terms of neuroimaging, ‘morphometry’ can be considered an approach that measures the morphological metrics of the brain, including the size, shape and position of a part of the brain or the whole brain (Ashburner and Friston 2000). Conventionally, this can be carried out by selecting an anatomical structure of research interest (e.g. the thalamus) and having its border delineated by an expert manually. The size, shape and position of the selected structure can be assessed and compared between groups. When it comes to VBM, the measurement focuses on the voxel level rather than the anatomical structure as a whole (Ashburner and Friston 2000; Ashburner and Friston 2001). The brain images from two individuals are ‘matched’ at the voxel level, so the difference can be revealed. Such a process of ‘matching’ is achieved by registering the individual brain images to a template image. It should be noted that when two brains are matched at the voxel level, some morphological differences, such as the shape of individual brains, cannot be revealed because the shapes of individual brains have already been registered to the standard image, via multiple steps of transformation (Figure 2.3c). The VBM focuses on the intensity of each voxel, which may disclose important clues about the types of brain tissues, while discounting the individual difference in the global shape of the brain (Ashburner and Friston 2000, Ashburner and Friston 2001). For simplicity, VBM can be recognized as ‘a voxel‐based comparison of the local concentration of grey matter between two groups of subjects’ (Ashburner and Friston 2000). In other words, it is a method sensitive to the local composition of brain tissues, ‘while discounting positional and other large‐scale volumetric differences in gross anatomy’ (Ashburner and Friston 2001).
2.3.3.2 Steps of VBM Analysis
Practically, VBM is frequently performed on the T1‐weighted MRI data, which shows a good contrast between WM (with higher/brighter intensity), cerebrospinal fluid (CSF), with lower/darker intensity), and GM (with moderate intensity). Conventionally, the VBM approach consists of the following steps: (i) tissue classification, (ii) spatial normalization and (iii) intensity modulation.
The first key step is tissue classification. The original T1‐weighted image will be matched to tissue probability images. These images contain the information regarding the probabilistic information that a given tissue type (WM, GM or CSF) is found in the voxel (Ashburner and Friston 2005). The original T1‐weighted image will be segmented according to the probabilistic images. The segmentation generates three separate images that contain the GM, WM and CSF area, respectively (i.e. GM/WM/CSF‐segmentation images). Notably, in these segmented images, the area of a tissue type is not defined by a binary value, which represents the clearly‐cut border of the segmented part of the tissue. Instead, the voxel intensity of the segmented images ranges from 0 to 1, which represents the proportion of a tissue type within a voxel. Therefore, a voxel may have an intensity of 0.8 in the GM‐segmentation image and 0.2 in the WM‐segmentation image, suggesting it more likely to be classified as GM compared to WM. The second key step is spatial normalization. Back to the original concept of morphometry, if one needs to compare at the voxel level, an essential step is to match the individual brain images with each other. In VBM, this is achieved by spatial normalization, which registers the individual brain to a template image. Conventionally, VBM analysis uses a customized template, i.e. an ‘averaged brain’ estimated by the brain images from all the subjects included in the analysis. Individual images are registered to the template via linear and nonlinear transformation (see Section 2.2). Finally, the intensity of images is usually ‘modulated’ for estimating the volumetric information. During normalization, the local geometric features have been ‘morphed’ so that some areas may be contracted and some areas can be expanded. Notably, after the local deformation, the intensity value of each voxel will need further adjustment to compensate for the change of volumetric expansion/contraction. This adjustment is performed by ‘modulating’ the voxel intensity, which integrates the voxel intensity (based on tissue segmentation) and the information of local deformation (Jenkinson and Chappell 2018).
2.3.3.3 Interpretation of the VBM Results
As noted in the preceding section, in the GM image derived from segmentation, the voxel value should be realized in a probability sense, i.e. how possible this voxel can be classified as part of grey matter. Therefore, this value does not quantify the ‘actual size’ of grey matter tissue. The VBM results consider both the results of tissue classification (e.g. the amount of GM) and the volume change (i.e. spatial normalization). Therefore, the results can be considered ‘the proportion of voxel that is grey matter, having adjusted for the confounding effects of warping the brains’, or from the perspective of volumetric change, as ‘the proportion of volume change attributable to grey matter’ (Ashburner and Friston 2001). Some interpretations of VBM results can be over‐simplified and misleading. For example, when a VBM analysis revealed a smaller grey matter volume of the hippocampus in patients with dementia, compared to healthy controls, the results should not be interpreted as a smaller hippocampal size because it ignores the fact that the volume change is adjusted from specific tissue type (here, for the grey matter).
2.3.4 Surface‐based Approach
The VBM approach analyzes the amount of a specific tissue type based on the concept that the brain can be dissected into multiple voxels, and all the analyses are performed at the level of brain voxels. The surface‐based approach, instead, is based on the concept that the brain is composed of layers of tissues. The importance of the layer structure can easily be recognized from the gross anatomy of the brain since the brain cortex is folded into multiple gyri and sulci. In the following sections, we introduce the basic concepts of the surface‐based approach for assessing brain morphometry.
2.3.4.1 Assessment of Cortical Thickness
The cortex is mainly composed of grey matter, which forms continuous layers folded into multiple gyral and sulcal regions. One of the primary morphological features of the layer structure is its thickness. In the surface‐based approach, such as the method based on FreeSurfer (Fischl 2012), the thickness of the cortical layer (i.e. cortical thickness) is defined as the distance between the grey/white and pial surfaces. The surface‐based methods have been proved to be a useful way of studying cortical thickness. For example, Fischl and Dale (2000) have investigated the thickness of the cerebral cortex from 30 younger adults, showing an average ± standard deviation thickness of 2.7 ± 0.3 mm in the gyral area and 2.2 ± 0.3 mm in the sulcal area. In general, the cortical thickness is within the range of 1– 4.5 mm, consistent with the findings from post‐mortem research (Fischl and Dale 2000). The thickness‐based analysis has provided useful information for our understanding of the brain. Firstly, the analysis considers the realistic shape of the brain cortex, which is full of folding areas. Secondly, the analysis is also used for assessing other surface features, including sulcal depth (as an index for the boundary between brain lobes) and curvature (i.e. how curved or flat the part of the surface is), in addition to cortical thickness. Thirdly, the analysis may also help to match images between individual subjects. For example, across subjects, the structural images can be normalized to a template by matching the position of sulci, which may provide more accurate registration of brain images between different individuals.
2.3.4.2 Segmentation of Brain Regions
As noted earlier, segmenting different types of brain tissues is a critical step for analyzing the structural features of the brain. This tissue segmentation can be performed decently by VBM. However, segmenting different brain structures, i.e. to label a voxel as part of the hippocampus or the amygdala, can be a real challenge because this classification cannot be carried out merely by assessing the intensity of a voxel. To achieve this, researchers have adopted positional information from the brain, using a similar concept as surface‐based analysis. In addition to assessing the information of voxel intensity, the relative spatial location of brain structures is considered in the algorithm of segmentation (Fischl 2012). For example, a voxel will show a greater probability of being classified as the amygdala than the hippocampus if its spatial location is in front of a voxel classified as part of the hippocampus (based on the a priori knowledge that the amygdala is anatomically anterior to the hippocampus). The surface‐based approach, such as the method based on FreeSurfer, has been widely used for the automatic segmentation of cortical and subcortical regions and the estimation of their volumes.
2.3.4.3 Steps of Surface‐based Approach
The general step of the surface‐based analysis is similar to VBM analysis, in which segmentation and spatial normalization play a key role. An obvious difference between VBM and the surface‐based approach is the method for image registration. In VBM, the individual brain images are registered to a template image, which is positioned in a 3D space composed of orthogonal axes in three directions. In the surface‐based approach, the registration is performed in a ‘surface space’, which can be visualized as a inflated spheric area that represents the whole surface area of the cortex. The ‘surface space’ is different from the ‘voxel space’ of VBM analyses. In VBM, brain voxels are organized in a fixed grid with three dimensions. In contrast, the surface space is organized as a mesh composed of multiple small triangles (i.e. faces) with intersected points (i.e. vertices). Notably, while the number of voxels is fixed in VBM (based on its spatial resolution), the number of vertices of the mesh is not fixed and needs to be estimated to fit the individual brain (Jenkinson and Chappell 2018). The different ways of organization of brain images have a profound influence on how data are analyzed.
Before registration, the original brain image needs to be transformed into this surface area by mapping the x‐, y‐ and z‐coordinates from the ‘brain space’ to the surface space. This is performed by an algorithm that deforms the folded brain (with a lot of sulci) into an inflated sphere, much like blowing air to a crumpled plastic bag to inflate it like a balloon. By this deformation, the locational information of the sulci and gyrus can be transformed into a smoother surface for analysis (Jenkinson and Chappell 2018). The template to be registered is also represented in the surface space, and therefore, within the same surface space, individual brain images are normalized to the template. Notably, because the 3D gyral and sulcal regions are ‘flattened’ into a surface form, many morphological features, including the surface area of cortical regions, can be analyzed via this approach.
2.3.5 Diffusion MRI
dMRI is able to ‘map the fibre architecture of tissue’ and, to the present, the only in vivo method for assessing the features of neural fibres (Jones et al. 2013). dMRI assesses the physical properties of the diffusion of water molecules in brain tissues. During MRI, increased signals are obtained with all the molecules which show a similar tendency in spinning (i.e. phasing). However, the signals from phasing may be attenuated with the molecules spin in different ways (i.e. dephasing). The dephasing effect is more prominent in a compartment where the molecules can freely diffuse in all directions, compared to a compartment that is restricted in diffusion. Therefore, dMRI assesses the degree of attenuation of signals, which reflects the diffusion of water molecules, and diffusion is associated with the presence of axon bundles in a compartment. In this compartment, water molecules can preferentially diffuse along the direction where axons are oriented (Jenkinson and Chappell 2018; Jones et al. 2013). Therefore, dMRI has been widely used for assessing the local microstructural properties of molecular diffusion and the estimation of anatomical connectivity (Jenkinson and Chappell 2018).
2.3.5.1 Assessment of Microstructure of White Matter
dMRI assesses the features of molecular diffusion from multiple directions and the attenuation of magnetic resonance signal associated with the molecules spinning. Therefore, when signal attenuation is more pronounced in one direction than directions, it signifies that in this particular direction, molecules can diffuse more freely compared to the other directions. In DTI, axial diffusivity (AD) is the diffusivity assessed along the long axis. Diffusivity is also assessed along the direction perpendicular to the axial direction, i.e. the radial diffusivity (RD). The mean diffusivity (MD) is the average of diffusion over all directions. The FA represents the degree that the estimated pattern of diffusion deviates from an isotropic form. The higher the FA value is, the more likely that diffusion is restricted in a single direction (i.e. anisotropic) rather than spread evenly in all directions. It is noteworthy that the local properties only reflect the molecular behaviour of diffusion, they cannot be overdrawn as an index of white matter integrity (which should be preserved in a pathological condition), and the changes in the local properties cannot be directly translated to the changes in structural connectivity (Jones et al. 2013).
2.3.5.2 Tractography: Estimation of Anatomical Connectivity
In addition, dMRI is also a powerful tool for researchers to explore the global architecture of the brain by answering questions such as ‘which gray matter regions are inter‐connected by white matter fibers?’ (Jones et al. 2013). To investigate the structural connectivity between brain regions, one would adopt the approach tractography, an analysis ‘to determine anatomical connectivity by tracing the paths of axonal fiber bundles through the white matter’ (Jenkinson and Chappell 2018). It should be noted that tractography is the method primarily for qualitatively visualizing the connection between grey matter regions based on the local properties of molecular diffusion. One needs to be careful when using the results of tractography for quantitative use, such as to identify the ‘number of fibres existing’ between brain regions. As shown in Section 2.4, the link between the streamlines estimated by tractography and the real ‘axonal fibres’ should not be simplified because the streamlines are estimated based on the properties of local diffusion, and the estimation per se is influenced by many factors, such as the density of fibres in a voxel and the length and shapes of fibres, which cannot be easily resolved by the dMRI data (Jones et al. 2013).
2.3.6 Further Considerations of Structural MRI
It is noteworthy that, again, spatial resolution plays a dominant role in interpreting all the structural results. For example, the VBM approach focuses on tissue‐specific features on the voxel level. Even when the spatial resolution is very high, one cannot guarantee that only one type of brain tissue exists in a voxel. The partial volume (PV) effect, i.e. the signals of a voxel is a ‘weighted average’ of the signal from different types of brain tissues, should be considered. Such a weight reflects the fraction of the volume associated with each tissue (Jenkinson and Chappell 2018). Likewise, dMRI does not provide a direct assessment of the size and density of axons or the degree of myelination in a voxel (Jenkinson and Chappell 2018). The PV effect should also be considered for dMRI because a voxel size is relatively large, and multiple fibres of different directions may be included in a voxel. To clarify the interaction between multiple fibres within a voxel is still a major challenge in dMRI (Jenkinson and Chappell 2018).
VBM is a method based on the intensity of the T1‐weighted image. The spatial distribution of the intensity values is affected not only by the tissue types but also by other imaging factors such as relaxation time (which is further associated with tissue myelination) and field inhomogeneity (Zatorre et al. 2012). The same consideration applies to dMRI. The dMRI signal is associated with the microscale architecture that hinders diffusion. However, diffusion per se can be influenced by other factors, such as the mobility of molecules, which further depends on the viscosity and temperature factor (Jones et al. 2013).
Finally, a general rule of the analysis of sMRI data is that the results from the current analysis are based on those from earlier analyses. That means the effect of any methodological variations (or even an error) from an earlier stage of analyses will contribute to later analyses. For example, the methods of tissue segmentation at the beginning of VBM and surface‐based analyses will affect the final results of the between‐group comparison. Therefore, the methodological details of imaging processing need to be documented and explicitly stated (Ridgway et al. 2008).
2.3.7 Summary
- VBM is the approach that assesses morphological features of the brain at the voxel level. The approach consists of three steps: tissue segmentation, spatial normalization and intensity modulation. The approach helps to identify the spatial distribution of grey matter and white matter and quantify their amount in a voxel by adjusting the size.
- The surface‐based approach is based on the concept that the brain is composed of layers of tissues. It has been widely used for assessing morphological features of the cortical layer, including cortical thickness, surface area and morphological features of gyri and sulci. The methods are also useful for segmenting brain regions and registration between images.
- dMRI assesses the degree of attenuation of signals at multiple directions, which reflects the degree that water molecules can freely diffuse in all directions. DTI has been widely used to investigate the local properties of diffusion (e.g. FA) that relate to the microstructure of white matter, and the global pattern of structural connectivity, via tractography.
Figure 2.6 Conceptual differences between functional specialization, functional segregation and functional integration. (a) Functional specialization highlights the association between a brain region and a specific mental function. For example, activation at the occipital lobe is considered mainly for the processing of visual perception. (b) Functional segregation highlights that a mental function is associated with multiple brain regions that are functionally connected within a module. For example, visual cognition is associated with the module consisting of the yellow regions, and motor control is associated with the module consisting of the blue regions. (c) Functional integration highlights the pattern of global communication between multiple brain regions. For example, individual variability in mental functions may be associated with the efficiency of how information is distributed in a network.
2.4 Research of Brain Connectivity
2.4.1 Introduction
It has been a long tradition for neuroscientists to pursue the functional ‘regions’ that are associated with specific mental functions. In terms of task‐based neuroimaging, this means to find a ‘blob’ in an image, i.e. a cluster of brain activation above a statistical threshold, in a specific task condition. The activation is associated with the mental functions induced by the task, as evidence of functional specialization/segregation of an anatomical region (Smith 2012; Genon et al. 2018) (Figure 2.6). More recently, our understanding of brain functions has focused on the complexity of the nervous system. The brain is considered a complex system in which mental states are associated with ‘the interaction between multiple physical and functional levels’ (Bassett and Gazzaniga 2011), rather than an assembly of independent anatomical regions, with each region specializing only for a single mental function (Figure 2.6a). The shift of research focuses is associated with a shift of methodological focus from the ‘blobology’ (i.e. focusing on the clusters or ‘blobs’ of brain activation related to a task) to ‘connectology’ (Smith 2012). In the following sections, we provide a brief introduction to the methods of investigating brain connectivity.
2.4.2 Segregation and Integration of Brain Functions
As a network where mental experience emerges, the brain is governed by some basic principles, including functional segregation and functional integration (Tononi et al. 1994; Friston 2011; Sporns 2013). The concept of functional segregation refers to ‘neuronal processing carried out among functionally related regions arranged within modules’ (Sporns 2013). This concept highlights that some brain units may work closely as a community or a function‐specific module for achieving specific mental functions (Figure 2.6b). In contrast, the concept of functional integration highlights how different parts of the brain interact with each other for achieving mental functions. In other words, in functional integration, one is mainly interested in ‘the efficiency of global communication’ and ‘the ability of the network to integrate distributed information’ (Sporns 2013) (Figure 2.6c). Notably, the two concepts are not exclusive to each other. The brain is balanced between the status of highly segregated (i.e. each part of the brain works completely independently) and the status of highly integrated (i.e. each part of the brain works completely dependently). The coexistence of segregation and integration is crucial to the complexity of the brain network (Tononi et al. 1994). At the core of our understanding of functional segregation and integration is the concept of ‘brain connectivity’, which can be generally considered as the pattern of links between brain regions. It may refer to the structural connectivity (i.e. ‘the existence of white matter tracts physically interconnecting brain regions’) (Uddin 2013) or the functional connectivity (i.e. ‘the temporal correlations between spatially remote neurophysiological events’) (Friston 1994). The methods for quantifying structural and functional connectivity are introduced in the following sections.
2.4.3 Functional Connectivity
Functional connectivity can be broadly defined as ‘the temporal correlations between spatially remote neurophysiological events’ (Friston 1994). Based on the definition, two aspects are critical for the assessment of functional connectivity. Firstly, the temporal correlations derive from the association between functional signals, such as the BOLD time series acquired via fMRI. Therefore, to calculate the correlation, one needs to first define the duration of the functional signals. For example, in resting‐state fMRI research, the correlation is calculated based on the BOLD time series during the whole resting period. Secondly, the functional signals derived from two spatially very close regions (e.g. two neighbouring voxels) will have a strong correlation because they are likely to be the same anatomical structure. Therefore, researchers are interested in the correlation of signals from geometrically remote brain regions. The analysis of functional connectivity helps to elucidate the association between the remote brain regions of a known neural circuitry.
2.4.3.1 Intrinsic Brain Functions
As noted earlier, functional connectivity can be used to quantify the functional signals collected during a resting state, in which subjects are not engaged in a task. Why is it important to explore the connectivity during a resting rather than a task condition? To explain this, one needs to consider the intrinsic brain functions, which are less related to the sensory and motor functions induced by a specific task. In other words, some brain functions are associated with the ‘baseline status’ (i.e. the non‐task‐specific conditions) of our mind. These functions include information processing for ‘interpreting, responding to and predicting environmental demands’ (Raichle 2010). Experimentally, this baseline status is set by asking subjects to relax and rest during fMRI scanning. The importance of the baseline status can be demonstrated by the fact that the brain, with only 2% of our body weight, consumes around 20% of the total energy during resting (Raichle 2010). Notably, even when the brain is actively engaged with a mental operation (e.g. performing a language task), the additional energy consumption is often less than 5% (Raichle 2010). The findings of the high baseline consumption of energy suggest that even when we are not engaged with a mental function overtly, the brain always maintains intrinsic and ongoing activities.
2.4.3.2 Spontaneous BOLD Activity and the Resting‐state fMRI
In terms of the connectivity of intrinsic brain functions, researchers focus on the temporal correlation of the spontaneous BOLD activity, a low‐frequency signal (0.01–0.1 Hz) collected during a resting state. The spontaneous BOLD activity can be defined as ‘the activity that is not attributable to specific inputs or outputs’ (Fox and Raichle 2007). Therefore, the activity is usually assessed in a resting state when subjects are not actively engaged with a specific sensory or motor task. The fluctuation of this BOLD activity may represent the stimulus‐independent mental activity, such as self‐referential processing or memory, which are considered part of intrinsic brain function (Fox and Raichle 2007; Raichle 2010). It may also represent a preparatory status for coming tasks by coupling the brain regions that usually work together for a specific function (Fox and Raichle 2007, Raichle 2010).

Figure 2.7 Analysis of resting‐state functional connectivity. (a) The spontaneous blood‐oxygen‐level‐dependent (BOLD) activity is acquired using resting‐state functional magnetic resonance imaging. Subjects fix their eyesight on a crosshair without additional external stimuli. (b) Brain images are segmented into multiple regions according to a brain atlas. (c) To each brain region, the mean BOLD time series is extracted by averaging the time series from all the voxels within a region. (d) Association between the regional time series is quantified with correlation coefficients. (e) The correlation coefficient represents the strength of the connection between each pair of brain regions. (f) In the seed‐based approach, a brain region of interest (i.e. the ‘seed’ region) is pre‐selected. Functional connectivity is calculated between the seed region and all the other voxels to explore the brain regions that have a strong connection with the seed region.
2.4.3.3 Research Approaches of Functional Connectivity
Cumulating evidence has revealed that even during the resting state when individuals are not actively engaged with external stimuli, the human brain still works actively, as demonstrated by the connectivity of the spontaneous BOLD activity. The study design of intrinsic functional connectivity is illustrated in Figure 2.7. Firstly, the spontaneous BOLD activity is acquired during a resting state, which relates to the intrinsic brain functions of a non‐task‐specific ‘baseline’ status (Figure 2.7a). The brain is segmented into multiple brain regions according to a brain atlas (Figure 2.7b) (see Box 2.4 in the Companion Website). For an individual brain region, BOLD time series is extracted from each of the voxels within the brain region, and a mean time series of a region is calculated by averaging the BOLD time series of all the voxels (Figure 2.7c). Several additional steps are included here, such as extracting the low‐frequency component of the time series. Next, based on the definition of functional connectivity, the strength of association between the brain regions is quantified by correlation coefficients (Figure 2.7d). The correlation coefficient represents the strength of the connection between each pair of brain regions (Figure 2.7e). For example, a stronger correlation is identified between region 1 and region 2, compared to the correlation between region 2 and region 4. The approach is helpful to elucidate a hypothesis based on a priori knowledge of neural circuitry. For example, one can compare the strength of functional connectivity between two plausible pathways (e.g. between region 1 and region 2 or between region 2 and region 4). One can also use the same approach for investigating individual differences in behaviour, such as quantifying the association between perceived pain and the connectivity of pain‐related regions.
Another widely used approach is the seed‐based approach. The approach explores the brain regions that are highly correlated with a ‘seed’ region at the voxel level. For the seed‐based approach, the BOLD time series from a pre‐defined brain region (i.e. the ‘seed region’) is collected, and correlation is calculated between the time series of the seed region and the time series from all the voxels outside the seed region (Figure 2.7f). This step will generate a functional connectivity map, which reveals the area with stronger connectivity with the seed region. The seed‐based approach is especially useful to explore the global pattern of brain regions that form strong functional connectivity with the region of our interest (i.e. the seed).
2.4.4 Structural Connectivity
Taking telecommunication as an analogue, functional connectivity of the human brain can be exemplified as the ‘communication’ between brain regions. More communication is represented by a higher coherence of BOLD time series. In contrast, the study of structural connectivity focuses on how lines are wired. In the human brain, these ‘lines’ reside in white matter, which is predominantly composed of neural fibres. Therefore, the primary approach to study structural connectivity is to investigate the spatial distribution of white matter of the brain, which can be assessed by dMRI, as discussed in Section 2.3. Another less straightforward approach is to estimate the covariance of structural features to provide a picture of the strength of association of structural features between different brain regions.
2.4.4.1 Structural Connectivity Based on Tractography
dMRI provides information regarding the local properties of molecular diffusion at the voxel level (see Section 2.3). Based on the dMRI data, tractography can be used to reveal the streamlines connecting brain regions. Structural connectivity is quantified according to the ‘streamlines’ estimated using the algorithm of deterministic tractography or probabilistic tractography. In deterministic tractography, each voxel is assigned with a single direction (Figure 2.8a), and a continuous curve (i.e., a streamline) is formed by tracking the direction of voxels (Jenkinson and Chappell 2018). In probabilistic tractography, there exists an uncertainty of the direction within a voxel. Therefore, the direction of a voxel may vary, forming a probability distribution. As shown by a simplified case in Figure 2.8b, the distribution presents a high probability for the leftward direction. However, it does not exclude the possibility of an upper direction, though the probability is smaller. Due to the probabilistic nature of voxel direction, it may not be adequate to track for a single streamline. Instead, tracking procedures are repeated many times, and different streamlines with random directions can be identified (Figure 2.8b) (Jenkinson and Chappell 2018). As shown in Figure 2.8b, probabilistic tractography reveals the tract consisting of the most likely direction from individual voxels. The intensity of a voxel represents the frequency that a streamline passes that voxel under a fixed number of sampling (of the streamlines). Therefore, a higher intensity suggests that it is more likely to find a streamline passing the voxel.

Figure 2.8 Analysis of structural connectivity. (a) In deterministic tractography, each voxel is assigned with a single direction, which reflects the principal direction of diffusivity. A continuous streamline is formed by tracking the direction of voxels. (b) Probabilistic tractography assumes that there exists an uncertainty of the direction within each voxel. In the right panel, the probabilistic distribution of the directions is estimated for each voxel. A higher probability of a ‘leftward’ direction can be identified. In the right panel, the intensity of a voxel represents the frequency that a streamline passes that voxel. For example, more streamlines pass the yellow voxel (here four out of seven streamlines) compared to the red voxel (here one out of seven streamlines). (c) Structural covariance quantifies the strength of association of a brain feature between different brain regions across subjects. For example, the cortical thickness of six brain regions is assessed for eight subjects. The right panel reveals the association between regions 2 and 6, as quantified by the correlation coefficient of the cortical thickness between the regions.
2.4.4.2 Structural Covariance
Structural covariance refers to the phenomenon that ‘inter‐individual differences in the structure of a brain region often covary with inter‐individual differences in other brain regions’ (Alexander‐Bloch et al. 2013). Simply speaking, in a group of subjects, two regions are structurally covaried if their structural features (such as cortical thickness) are highly correlated across the subjects. Notably, the concept of structural covariation is established on the group level. The covariation reflects the association of inter‐individual differences in structural features. Therefore, the first step of calculating structural covariation is to quantify the structural features across the subjects, such as assessing the average cortical thickness from brain regions. The degree of covariation can be quantified by the correlation coefficient between the regional cortical thickness across all the subjects (Figure 2.8c). The biological mechanisms underlying structural covariance have not been fully elucidated. The pattern of inter‐regional association revealed by structural covariance shows a substantial degree of overlap with the pattern from intrinsic functional connectivity (Kelly et al. 2012b) and the structural connectivity based on diffusion connections (Gong et al. 2012). The brain regions covary in structural features may share coordinated rates of developmental process (Alexander‐Bloch et al. 2013).
2.4.5 Analysis of the Brain Connectivity Using a Network‐based Approach
In the previous sections, we outlined several widely used methods to quantify functional and structural connectivity. In general, the approach is very useful if we want to analyze the connection between two specific regions, which have already been known as critical elements in a neural pathway. These methods help us to quantify the strength of the connectivity. However, when it comes to the global pattern of connections of brain regions, one needs to assess the property of a network, which consists of multiple connections. From the biological perspective, mental functions are associated with the myriad numbers of interactions between multiple brain regions. If we aim to study how efficiently different parts of the brain communicate with each other, the key is to understand how the individual connections assemble into a whole network. The term ‘network’ refers to a real‐world complex system, defined by a collection of nodes and links (Rubinov and Sporns 2010). Mathematically, a network can be considered as a graph consisting of ‘vertices’ (i.e. nodes) and ‘edges’ (i.e. links) (Figure 2.9a). Therefore, the structural or functional network of the brain can be visualized as a graph composed of nodes (i.e. brain regions) and linked by edges (i.e. the connectivity between regions). By analyzing the topological features of the graph, researchers can identify the structural or functional architecture of the whole brain.
2.4.5.1 The Definition of a Node
In the graph‐based network analysis, the definition of nodes is the most fundamental issue. A node can be conceived as the elemental unit to define a brain region. The links between brain regions (e.g. the inter‐regional correlation of the BOLD time series) can be quantified only when we have defined what a brain region is. For example, the node can be a brain structure with a clear anatomical border, such as the thalamus and the amygdala, and functional connectivity is quantified as the correlation between average BOLD time series from all the voxels within each region. It can even be a single voxel, and functional connectivity will be quantified between all the pair‐wise BOLD time series from all the voxels. The definition of nodes is sometimes associated with the ‘resolution’ of the brain network. A network may consist of around 100 nodes, each defined as a structurally or a functionally distinct region. In contrast, a network may consist of more than 10 000 nodes when a node is defined at the voxel level. To elucidate how nodes are defined is essential to interpret the meaning of a network properly (Butts 2009). For example, not all the ‘brain regions’ are structurally or functionally well defined as the thalamus or the amygdala. The border of some regions, such as the dorsolateral prefrontal cortex, may show great inter‐subject variability. For structural connectivity, one may define the region based on some anatomical landmarks (e.g. gyri and sulci). However, the same definition may not reflect the same brain functions across subjects. For example, it may be invalid to claim that the same area of the dorsolateral prefrontal cortex is ‘the node for attention control’ to all subjects. Secondly, back to the problem of the resolution of a network, we need to consider how a structure should be defined as a functionally distinct unit. For example, it seems reasonable to define the thalamus and the amygdala as different nodes because they are associated with sensorimotor and cognitive–affective processing, respectively. However, in the thalamus, different sub‐nuclei (e.g. the ventromedial or lateral nuclei) are associated with different roles in sensorimotor processing. Should we include the whole thalamus as a node? Or should each of the sub‐nuclei be investigated as an individual node? This will lead to different biological interpretations of a brain network.

Figure 2.9 Graph‐based analysis of brain connectivity. (a) The pattern of functional and structural connections between brain regions can be translated from the ‘brain space’ to the ‘network space’ with applications of graph theory. In a graph, the nodes represent brain regions and the links represent the functional and structural connectivity between regions, which can be quantified by the correlation coefficient between blood‐oxygen‐level‐dependent (BOLD) time series and the streamlines identified by tractography, respectively. (b) In the network analysis, the global metrics quantify the degree of integration of a network. For example, characteristic path length can be calculated by finding the shortest path length between a pair of nodes, such as the path A–B–D (but not A–B–E–D) between the nodes A and D. (c) The local metrics quantify the degree of segregation of a network. For example, the clustering coefficient is used to quantify the fraction of the triangular architecture in the whole network (e.g. A–B–C and E–G–H), which represents a pattern of clustered nodes. Notably, a small‐world network offers a balance between the efficiency of global and local communication. A highly regular network (i.e. the middle‐right panel) and a highly random network (i.e. the middle‐left panel) may suffer from a lower global and local efficiency, respectively.
2.4.5.2 The Definition of a Link
As noted above, the link between nodes can be quantified using different indices of functional or structural connectivity. However, different background settings of analysis will have a great impact on the resulting connectivity. For example, if we define the correlation between the BOLD time series as the link in a network of functional connectivity, we may find both positive and negative correlations within all the pairs of nodes. What is the biological meaning of the ‘negative connectivity’ (i.e. a link with a negative correlation coefficient)? Should we include the results of negative connectivity or exclude them so that only the links with positive connectivity are investigated? A network with both negative and positive connectivity and a network composed of only positive connectivity should be interpreted differently. Another analytical issue is the binarization of the value of a link. On the one hand, we may binarize the value of correlation as 0 or 1 (i.e. to classify a link as ‘presence’ vs. ‘absence’) by a pre‐defined threshold. On the other hand, we may keep the value to form in a weighted network so that the strength of connectivity can be treated as a continuous variable. The issues highlight the fact that the results of network analysis are sensitive to the analytical steps of defining a link (Murphy and Fox 2017).
2.4.6 Metrics for Assessing a Brain Network
In recent years, graph‐based network analysis has become an important tool for investigating the pattern of brain connectivity. When a network is defined, there are many well‐developed mathematical tools for assessing the topological features of the network. Critically, these topological metrics derived from a graph may help us elucidate the biological meaning of brain networks, such as the efficiency of information exchange between brain regions. In the following sections, we outline several widely used metrics that are associated with the analysis of brain networks.
2.4.6.1 Global Metrics
Characteristic path length (PL) and global efficiency (GE) are two widely used metrics to quantify the degree of integration of a network (Rubinov and Sporns 2010) (Figure 2.9b). PL is the average ‘shortest path length’ between all pairs of nodes. A smaller value of PL means averagely fewer ‘steps’ to pass through between each pair of nodes. The GE, in contrast, is calculated based on the inverse PL. Therefore, a network with a shorter PL and a higher GE reflects the better efficiency of integrating information between nodes. Notably, GE or PL is also the major feature for evaluating if a network demonstrates a ‘small‐world’ architecture (Figure 2.9b). A ‘small‐world network’ is both highly integrated and segregated – it is more clustered than random networks (i.e. increased segregation) while preserving short PL, compared to regular networks (i.e. increased integration) (Watts and Strogatz 1998). Based on the analysis of PL and GE, researchers have identified that the structural and functional networks of the human brain also demonstrate a small‐world architecture (Bassett and Bullmore 2017).
2.4.6.2 Local Metrics
While PL and GE contribute to assessing the degree of function integration, the metrics of clustering are helpful to quantify the degree of functional segregation. One of the simplest forms of ‘clustering’ of nodes is to form a triangular connection (Figure 2.9c), in which all three nodes fully connect. Clustering coefficient (CC) is used to quantify the fraction of the triangular architecture in the whole network. A higher CC represents that the pattern of clustered nodes is more prevalent in a network (Rubinov and Sporns 2010). Another common index for segregation is modularity, which reflects the degree that a network is organized into a modular architecture, which consists of multiple ‘modules’ (or communities). A module can be generally defined as a group of nodes sharing a high degree of within‐group connectivity and, at the same time, a low degree of between‐group connectivity (Rubinov and Sporns 2010). Just like the small‐world architecture, being modular is also a key feature of the functional or the structural network of the brain (Bassett and Bullmore 2017).
2.4.7 Methodological Considerations of Brain Connectivity Research
As one of the neuroimaging fields with the fastest advancement, research on brain connectivity has been revisited for many methodological issues. As outlined in the following sections, these methodological considerations are important to interpretations of the biological significance of brain networks.
2.4.7.1 Confounding Factors of Analyzing Functional Connectivity
Several factors may confound the findings of intrinsic functional connectivity. Firstly, the functional signals acquired during a resting state are associated with not only our baseline mental functions but also individual physiological processes (Kelly et al. 2012a; Murphy et al. 2013). It is estimated that 5–15% of the variance in intrinsic BOLD activity is accounted for by cardiac and respiratory processes (Kelly et al. 2012a). Therefore, individual differences in these physiological processes, which are especially pronounced in patients with systemic diseases, should be carefully evaluated when we interpret the results of functional connectivity. Secondly, head motion has a substantial impact on the results of functional connectivity analyses (Van Dijk et al. 2012). The effect of head motion may vary in different brain regions. Increased head motion is associated with stronger intrinsic functional connectivity of motor network but weaker connectivity of the default mode and frontoparietal networks (Van Dijk et al. 2012). Critically, the degree of head motion may differ between different groups of individuals, such as younger and older subjects. Therefore, the age factor should be carefully considered when one compares the pattern of connectivity between different groups. Thirdly, one issue that has been debated for years is the use of global signal regression, an analytical step to remove the global mean BOLD signal, which may be unrelated to neural activity (Murphy et al. 2013). However, the adoption of this step is associated with an increased proportion of negative connectivity in the functional network (i.e. more links with anti‐correlation between the BOLD time series), which may greatly influence how we interpret the results (Murphy and Fox 2017).
2.4.7.2 Misinterpretation of Findings of Structural Connectivity
A common myth of interpreting the local properties of molecular diffusion is that ‘if there is a greater FA in a voxel, there are more neural fibres’. Such a direct translation between the imaging findings at the voxel level and the biological features of neural fibres is not valid. Even for the findings of tractography, we need to keep in mind that some biological features, such as the size, shape and length of neural fibres, cannot be directly assessed from tractography (Jones et al. 2013). Therefore, one should be careful of generalizing the results of structural connectivity into clinical conditions. For example, a claim that ‘individuals show loss of white matter integrity’ merely based on a change of FA or MD value should be revisited cautiously (Jones et al. 2013). Similarly, the ‘tracts’ demonstrated by tractography merely reflect the pattern of streamlines passing through different brain regions. The neuroanatomical features of neural fibres need to be confirmed by other methods, such as tract‐tracing methods based on neurochemical agents.
2.4.7.3 Methodological Variations of Graph‐based Analyses
As shown previously, graph‐based network analysis is a powerful tool for quantifying some topological metrics, which help us to understand the pattern of integration/segregation of brain networks. However, we should keep in mind that all the metrics are calculated based on the network. Therefore, it is critical to evaluate if the definitions of nodes and links represent the biological architecture of our brain. Specifically, the nodes of the brain network should represent a single entity that takes part in the relation of a network, and the links should reflect the biological meaning of the relations (Butts 2009). In the literature of graph‐based analysis of brain networks, there exists a great methodological variation in defining nodes and links for brain networks. Many studies defined the nodes based on structural features, which can be reliably reproduced between subjects. However, structural boundaries of different parts of the brain cannot be equated to their functional boundaries (Smith 2012). An alternative method is to define a functional region according to the map of brain activation derived from task‐based research (Fornito et al. 2013) (see Section 2.2). However, the size/location of these functional regions still show a substantial variability between subjects. The variability would be pronounced when it comes to mental functions regarding cognitive–affective processing, which usually shows a great variation in the location and size of task‐based brain activation.
The methods for defining links also exist with a great variation. One of the key issues is the use of a threshold on the value of a link. When a threshold is applied on a network, a link will be preserved if its value is above the threshold, and it will be removed if its value is below the threshold. In other words, after thresholding, only links of stronger connectivity will be preserved for graph‐based analysis. The criteria for an ‘optimal’ threshold are still under debate (Fornito et al. 2013; Smith 2012). If the threshold is too high (i.e. only the links of very strong connectivity will be preserved), the network will become very sparse, and the sparsity may influence the topological metrics. In general, one should interpret the results of brain networks only when the analytical methods of network analysis are elucidated.
2.4.8 Behavioural Considerations of Brain Connectivity Research
While the behavioural meaning of brain activation can be explained by the related task condition, the interpretation of findings of brain connectivity is sometimes obscure if the findings are not associated with some behavioural/clinical variables (Kelly et al. 2012a) (see Box 2.5). For example, intrinsic functional connectivity between the amygdala and the anterior insula merely reflects the temporal correlation of the BOLD time series between the two regions during a resting state. The meaning of the connectivity can be better interpreted when behavioural/clinical variables, such as subjects’ ratings of trait anxiety or symptoms of anxiety disorders, are included in the analysis. For example, one can investigate if increased anxiety is associated with stronger amygdala–insular connectivity at the individual level. In the following sections, we discuss several key issues for a better interpretation of the behavioural meaning of brain connectivity findings.
2.4.8.1 What Does ‘Resting‐state’ Function Connectivity Represent?
The term ‘resting state’ may give a false impression that subjects are ‘doing nothing’ during the scan. The meaning of ‘resting’ should be interpreted relative to a task condition. A resting state means subjects are less actively engaged with external stimuli or complex mental processing, i.e. a non‐task‐specific condition. It does not mean the brain is totally shut down – since the brain is always active (Raichle 2010). Even during a resting state, some essential mental functions are maintained for responding to environmental demands. Again, because individuals are not engaged with an explicit task in the resting state, it would be difficult to interpret the behavioural meaning of intrinsic functional connectivity. Behavioural/clinical variables of individual subjects should be carefully collected to clarify the association between intrinsic functional connectivity and individual variations in behavioural/clinical aspects.

VIDEdental - Online dental courses
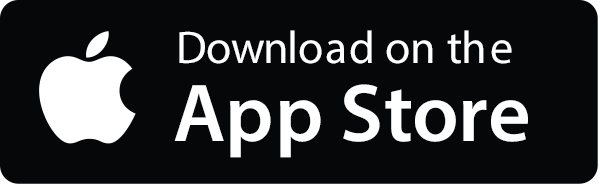
