Highlights
- •
Different failure modes were considered as competing risks in statistical analyses.
- •
Conventional failure mode non-distinguishing models may underestimate bond strength.
- •
Competing risk models could estimate bond strength more accurately and reliably.
- •
Additional information can be obtained from the competing events’ strength estimates.
Abstract
Objectives
A competing risk (CR) model distinguishing adhesive, cohesive and mixed failures as competing events was used for the analysis of micro-tensile bond strength (μTBS) data and compared with a conventional failure mode non-distinguishing survival model.
Methods
Fifty human molars were bonded using five universal adhesives (n = 10) and subdivided according to aging conditions (24-h water storage, thermocycling). After μTBS to dentin was tested, a fractographic analysis was performed using scanning electron microscopy. Survival analyses of the μTBS data were performed using both a failure mode distinguishing Weibull CR model, and a conventional failure mode non-distinguishing Weibull model. Weibull shape ( m ) and scale (σ θ ) parameters were calculated for both models using the maximum likelihood estimation method, and strength at 10 % probability of failure, σ 0.10 , was estimated. Groups were compared using 95 % confidence intervals.
Results
CR-model estimates of σ θ and σ 0.10 for adhesive failures were higher than those of the conventional model, more markedly in groups with lower percentages of adhesive failures. CR-model strength estimates for cohesive failures were similar in all groups regardless of their bond strengths and failure mode distributions.
Significance
Merging all bond-strength data into one dataset irrespective of the failure mode may result in a severe underestimation of bond strength, especially in groups with low incidence of adhesive failures. Bond-strength data analysis using a CR model could provide more accurate estimates of bond strength, and strength estimates for cohesive failures which were apparently independent of bond strength could serve as an internal validity indicator of the CR model.
1
Introduction
The essence of bond strength testing is that two substrates are bonded together using an adhesive, and the adhesive joint is then stressed until it fails. While failures at the adhesive-substrate interface are indicative of bond strength, failures also occur outside of this interface [ ], especially when testing adhesion to brittle substances, such as hard dental tissues. In fact, when contemporary dental adhesives with improved properties are evaluated, cohesive and mixed failures are frequent, sometimes even prevailing over adhesive failures. However, the strength of non-adhesive failures does not equal bond strength [ ], because the adhesive-substrate interface has not fractured.
Although fractographic analysis is performed in most studies to determine the failure mode, all acquired strength data are usually merged into one dataset for statistical analysis [ ], which may lead to the misinterpretation of the effects studied. Some researchers have proposed the exclusion of non-adhesive failures from the statistical analysis [ , ], but that would overlook the information provided by non-adhesive failures and cause bias [ ]. Moreover, in groups with larger proportions of non-adhesive failures, their exclusion would notably reduce the sample size and thus the statistical power. The issue of non-adhesive failures will become even more important over time, as properties of modern adhesives are continuously improving. They should therefore be taken into account adequately in statistical analyses.
Recently, there has been an increase in the use of Weibull statistics in dental research for the evaluation of mechanical properties of dental materials [ , ]. The two-parameter Weibull distribution function of strength, σ, is considered more appropriate for brittle materials than the symmetric Gaussian distribution. It is defined by the probability density function:
fσ=mσθσσθm-1e-σσθm
where m is the shape parameter (modulus) which is inversely proportional to the scatter of strength values, and its high values are indicative of high material reliability [ ]. σ θ is the scale parameter, also known as characteristic strength, and it equals strength at a 63.2 % probability of failure. It follows from Eq. (1) that the probability of failure at a certain strength, P f , can be calculated using the cumulative distribution function:
Pf(σ)=1-e-σσθm
The Weibull distribution also allows for taking failure mode into account in the statistical analysis. Previously, non-adhesive failures have been considered right-censored data in an evaluation of denture repair [ ] and in our recent study of composite-to-composite repair bond strength [ ]. In general, censoring occurs when observations or measurements are unfinished, for example due to the withdrawal of a subject from the study, the end of the study, or exceeding the range of the measuring instrument. Consequently, a censored value is equal to the pre-set threshold, but the actual value is larger and can be estimated using a suitable statistical model. The rationale for considering non-adhesive failures to be right-censored data was that the adhesive joint has not fractured by the end of the measurement, indicating that the actual bond strength had to be higher than the strength recorded at failure. While this approach is more accurate than simply analyzing all strength data together, it is not optimal either, because the censoring threshold should be fixed and unrelated to failure. For example, the strength of a specimen which has not fractured even under the maximal stress that can be produced by the testing machine can be considered a right-censored value. However, non-adhesive failures terminate the testing at variable strength values and preclude the events of interest – adhesive failures. They should therefore be regarded as competing events rather than censored observations.
If competing events are treated as censored data, the resulting estimator tends to overestimate the probability of failure and hence underestimate bond strength. Therefore, statistical models designed for competing risks (CR), which can distinguish different failure modes, should be preferred. Firstly, CR models describe the actual situation more specifically than conventional (failure mode non-distinguishing) or censored models, so they can provide more accurate estimates of bond strength. Furthermore, failures of brittle materials are caused by stress concentration at intrinsic flaws and the Weibull distribution assumes that flaws within the tested specimen are of the same type and size distribution [ ]. However, in the case of bond-strength testing, the assumption of such a flaw distribution is not fully satisfied, because flaw populations in the adhesive, substrates and at the interface are different. This may result in different Weibull parameters for each failure mode, so the use of a separate Weibull distribution for each of them in a CR model could lead to a better fit. Lastly, CR models can estimate the strengths for the competing events which may be valuable in some cases. Suppose a situation with three competing events, the probability of failure for the first one would be:
Pf1(σ)=∫0σm1σθ1xσθ1m1-1e-xσθ1m1e-xσθ2m2e-xσθ3m3dx
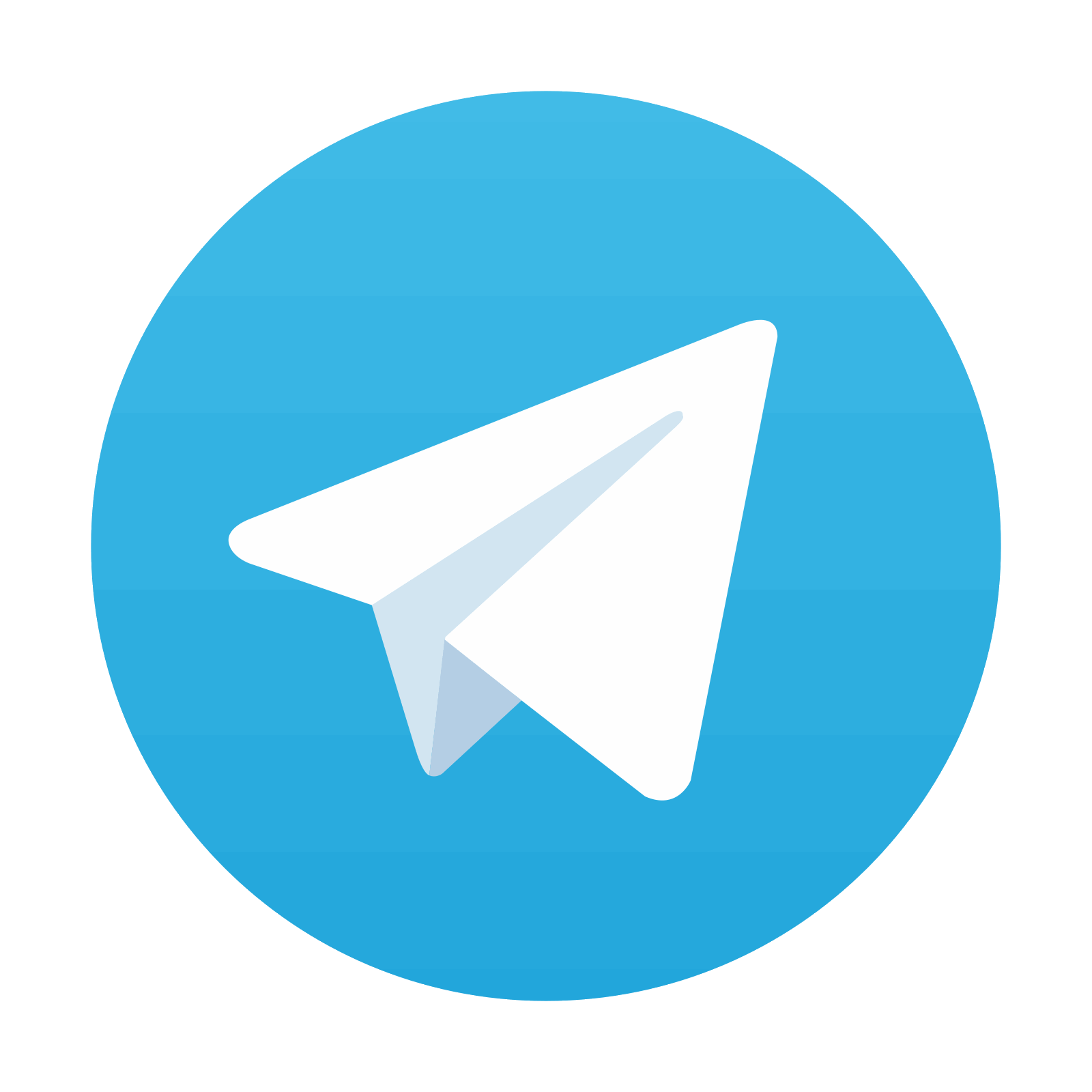
Stay updated, free dental videos. Join our Telegram channel

VIDEdental - Online dental courses
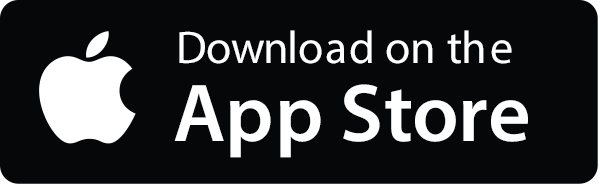

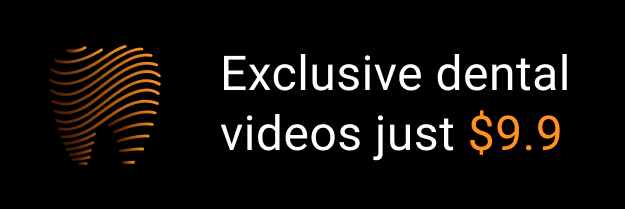