Introduction
In this article, we present an evaluation of user acceptance of our innovative hand-gesture–based touchless sterile system for interaction with and control of a set of 3-dimensional digitized orthodontic study models using the Kinect motion-capture sensor (Microsoft, Redmond, Wash).
Methods
The system was tested on a cohort of 201 participants. Using our validated questionnaire, the participants evaluated 7 hand-gesture–based commands that allowed the user to adjust the model in size, position, and aspect and to switch the image on the screen to view the maxillary arch, the mandibular arch, or models in occlusion. Participants’ responses were assessed using Rasch analysis so that their perceptions of the usefulness of the hand gestures for the commands could be directly referenced against their acceptance of the gestures. Their perceptions of the potential value of this system for cross-infection control were also evaluated.
Results
Most participants endorsed these commands as accurate. Our designated hand gestures for these commands were generally accepted. We also found a positive and significant correlation between our participants’ level of awareness of cross infection and their endorsement to use this system in clinical practice.
Conclusions
This study supports the adoption of this promising development for a sterile touch-free patient record-management system.
Highlights
- •
We have developed a novel sterile, low-cost method to assess 3-dimensional digital models.
- •
Touchless commands to manipulate the models were based on unique hand gestures.
- •
Our subjects endorsed the hand gestures as suitable commands to control the system.
- •
Subjects found the gestures comfortable, and the touchless commands were accurate.
- •
Awareness of cross-infection issues correlated with the acceptance of this method.
Many countries across the world require electronic patient records. Accessing these records via keyboard, mouse, touch screen, or pad raises the risk of cross infection. Infectious pathogenic microorganisms such as cytomegalovirus, herpes simplex virus types 1 and 2, hepatitis B virus, human immunodeficiency virus, hepatitis C virus, and bacteria that colonize or infect the oral cavity and respiratory tract such as staphylococci, streptococci, and Mycobacterium tuberculosis are occupational hazards that can be transmitted by contact, direct or indirect, between dental health care personnel and patients. Although gloves are a personal protective barrier between clinicians and patients, they still need to be frequently removed, at some inconvenience, when health care personnel are operating computer input devices during treatment.
This problem of touch-induced risk of cross infection during navigation of medical records may be minimized via a touch-free gesture interface with motion-capture camera devices such as Kinect (Microsoft, Redmond, Wash), with the ability to distinguish color images and the associated depth data, coupled with innovative programming strategies, and has furthered the development of an accurate contact-independent controlling device. This low-cost and easy-to-set-up device may also encourage improved cross-infection prevention in practice, especially in countries where inaffordability is a limiting factor, leading to poorer cross-infection control and safety practices.
Prototypes of gesture-based programs for Kinect have been presented for navigation of radiologic images during operating procedures. Furthermore, such prototype programs have been successfully pilot tested during surgical procedures while maintaining operator sterility, demonstrating the potential for improved cross-infection strategies.
In view of this potential, we have developed a hand-gesture–based program for practical cross-infection control when accessing patients’ electronic records in dental settings. Our prototype was designed to facilitate assessment of the 3-dimensional (3D) digitized dental study models from the sagittal, vertical, and transverse planes. The dental study model was chosen as the object of interest for this program because it is commonly used for baseline records, treatment planning, and monitoring changes. Most currently used digital forms of study models reproduce dental features with an acceptable level of clinical accuracy. It is expected that the future of dentistry will involve their use in place of stone study models for easy access and space-saving storage. Our prototype uses Kinect to provide a sterile method for the dentist to naturally and efficiently manipulate the 3D digital study models with specific noncontact hand-gesture commands.
The rationale of this study was to evaluate the developed system as a method to lower the risk of cross infection in clinical practice. We assessed user acceptance of our proposed hand-gesture user interface for interaction and manipulation of a 3D digital object. We investigated whether the prototype accurately discriminated each hand gesture to be translated for each specific command to the program and at the same time whether these gestures and the system were acceptable to the users.
Material and methods
Ethical approval for this study was obtained from the Medical Ethics Committee, Faculty of Dentistry, University of Malaya, Kuala Lumpur (DF OTI306/0078[U]).
This section describes the building of a robust hand-gesture recognition system using, as the input device, the Kinect sensor, which captures the color image and the depth map at 640 × 480 pixel resolution. Because the depth sensor of Kinect is an infrared camera, the lighting conditions, the background, and the colors of a patient’s skin and clothing have little impact on its performance. In this study, the Kinect sensor was connected via a 2.0 USB connector to an Ideapad Z460 (14-in screen size; Lenovo, Beijing, China), a Core i5-380M processor (Intel, Santa Clara, Calif), a graphic engine (Windows 7 Home Basic; Microsoft), and GeForce with CUDA (Nvidia, Santa Clara, Calif). We used Visual Studio 2010 (Microsoft) with our developed hand-gesture recognition program as detailed in the next paragraph. The aim was to provide a more natural human-computer interface, allowing the dentist to “pick up” the 3D digitized orthodontic study model by moving the hands within the working area, which detects the action as an initiative to move the model, and to “examine” the model by maneuvering the hands in the air.
The system comprises a touchless 2-hand-gesture navigational scheme for 7 commands: translation, zoom in, zoom out, rotate up and down, rotate side to side, select menu, and reset. Translation moves the 3D study model from one location on the screen to another. Zoom in and zoom out increase and reduce the model size, respectively. Rotate up and down refers to the rotation of the model around a horizontal axis, whereas rotate side to side refers to its rotation around a vertical axis. Select menu allows 1 of the 3 options on the right side (menu bars) of the screen to be activated and displayed on the main screen. The options include select the maxillary arch, mandibular arch, or both arches in occlusion. Reset returns the object to its original position and size on the active main screen, as shown in Figure 1 , A .
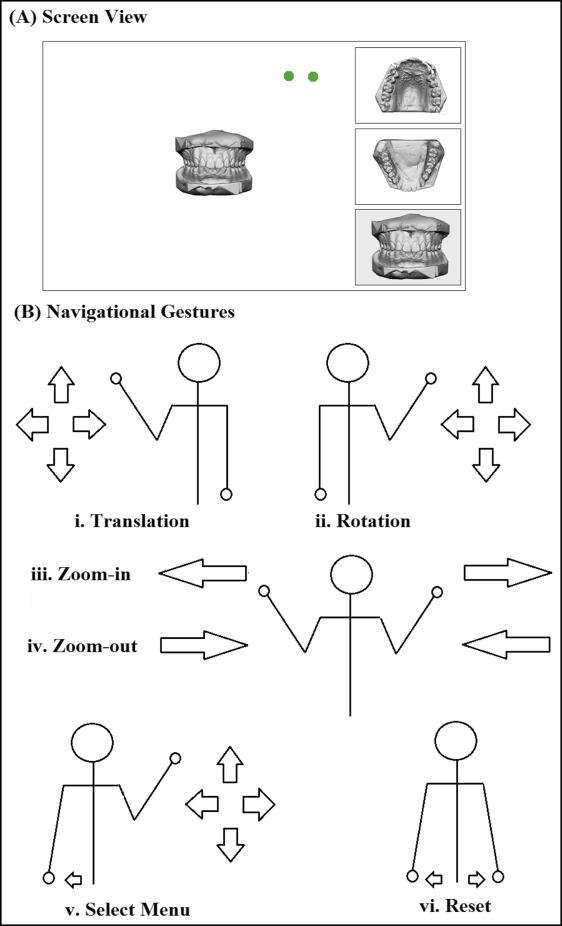
The working distance between the Kinect sensor and the participants was approximately 2 meters. Two green circles, representing each hand, appeared onscreen when the users were within the working area ( Fig 1 , A ). Detection of unintended movement was avoided by moving the hands out of the working area, as indicated by the disappearance of the green circles. As shown in Figure 1 , B , translational movement was controlled by moving the left hand in the vertical or horizontal direction. Rotations in the up-and-down and side-to-side directions were achieved by right-hand vertical and horizontal shifts, respectively. For zooming in to the models, the hands moved apart from the center of the body. For zooming out of the models, the hands moved toward the center of the body. Placing the left hand slightly apart from the side of the user’s body activated the side menu, as indicated by a color change on the side menu bar. An active cursor then appeared for the right hand to guide toward the desired menu, which is selected by a gentle tapping movement of the hand. Reset was activated when both hands were moved apart slightly from the sides of the body.
Our subject inclusion criteria specified health care associates between 21 and 40 years old with fully functional bilateral hands and good eyesight.
The exclusion criteria included those with medical conditions such as arthritis that may be affected by repetitive hand movements and those, such as epileptics, who may be affected by the displayed images. We also excluded those who could not read English.
Since Rasch analysis was planned for this study, it was estimated that a sample of 108 to 243 participants would be sufficient to give 99% confidence that the estimated value is within ±0.5 logits. For this study, we targeted a sample of 201 participants.
Data collection and instrumentation
The questionnaire comprised 4 parts: part A, demography of the participants; part B, their feedback on the accuracy and comfort with the hand-gesture system; part C, their feedback on suitability, offensiveness, and maintainability of the hand-gesture system; and part D, their perception of the usefulness of the system in relation to cross-infection control. Opinions were inventoried with Likert-scale answers.
In part B, the participants were introduced to the navigation interface of the system. They were allowed to navigate the digital study model freely until they were satisfied with their familiarity of the hand gestures. They were then required to control the model using the appropriate hand gestures according to randomly selected commands called out by the investigator (N.M.S). After they had randomly performed all 7 commands (T1), the process was repeated immediately 2 additional times. The participants later rated their perceived accuracy of the hand-gesture–based commands and their comfort level when performing the hand gestures to achieve the outcome. A 7-point Likert scale was used to rate the perceived accuracy of each participant’s use of the gestures, with 1 as “absolutely not able to achieve the action as accurately as desired at all,” 4 as “neutral,” and 7 as “absolutely able to achieve the action as accurately as desired.” Similarly, the level of comfort with which the participants used the system was evaluated with 1 as “extremely uncomfortable,” 4 as “neutral,” and 7 as “extremely comfortable.”
Part C required the participants to rate whether the hand gestures of each command were either suitable or offensive, and whether they should be maintained. Suitability measured the participants’ perceptions of the appropriateness of the gestures to their natural intuitiveness of actions to achieve the commands, whereas offensive assessed whether the gestures would be culturally unacceptable. The maintenance question inquired whether they thought that the system is acceptable as it is or whether it should be changed. Participants were also encouraged to suggest other preferred gestures for each command at the end of the questionnaire. Responses were measured using a 7-point Likert scale with 1 as “strongly disagree,” 2 as “quite disagree,” 3 as “slightly disagree,” 4 as “neutral,” 5 “slightly agree,” 6 as “quite agree,” and 7 as “strongly agree.”
Finally, they were asked questions that assessed their awareness of cross-infection risks and sought their endorsement of the system for cross-infection control. The awareness subscale comprised 2 item measures: “I am aware that I can get infectious diseases when I go the hospital or clinic (including the dental clinic)” and “I worry that I may get infectious diseases when I go the hospital or clinic (including the dental clinic).” The endorsement subscale comprised 4 item measures: “It is important for the people working at the hospital or clinic (including the dental clinic) to practice good cross-infection control,” “The motion-capture camera system may be a practical way as an input device to access patient records on computers,” “The motion-capture camera system is a good method to prevent cross infection,” and “The use of the motion-capture camera system should be encouraged for cross-infection control.” The Likert scales used to rate their answers were similar to those in part C.
The questionnaire was validated for content by 3 experts (N.L.A.K. and 2 others). It was piloted on 20 participants. Minimal language amendments were made for cultural propriety while maintaining the validity of the content. It was further tested on another 20 participants twice at least 7 days apart. One participant dropped out. The questionnaire was considered reliable, since the weighted kappa showed no significant differences for at least 70% of the responses. The validated questionnaire was given to the recruited participants, none of whom had served in the validation studies.
Statistical analysis
Winsteps (version 3.80.1; Winsteps, Beaverton, Ore; available at www.winsteps.com ) was used to analyze the raw ordinal ratings. Rasch analysis was used to allow our participants to be ranked along the same linear logit scale of the perceived rated qualities of the items measured.
Rasch analysis estimated the participants’ perceived accuracy of the hand-gesture–based commands and comfort level when giving the hand gestures. Participants’ perceptions of the attributes of the hand gestures, whether they were “suitable” or “not offensive” (reversed value for the offensive ratings) and should be “maintained” for the related command, were also analyzed using Rasch analysis.
The internal consistency of items measuring the infection-control subscales were acceptable (Cronbach alpha = 0.74 and 0.87, for awareness and endorsement subscales, respectively). We calculated the person measure for each subscale by Rasch analysis. We then assessed the relationship between these subscales by Pearson correlation coefficient and by cross-plotting the person-measure values.
Results
We recruited 201 participants: 89 men and 112 women (mean age, 25.05 years; SD, 4.44 years). They comprised students (62.2%) and temporary (4.0%) and permanent (33.8%) employees at University of Malaya, Kuala Lumpur. The participants’ areas of expertise were medicine (55.7%), dentistry (27.9%), nursing (15.9%), or radiology (0.5%). The majority were right-handed (84.1%); others were left-handed (6.5%), mixed-handed (changing hands depending on the task) (8.0%), and ambidextrous (performing any task equally well with both hands) (1.5%).
The mean duration of each hand-gesture–based command was 8.61 seconds (SD, 2.78 seconds).
In the Rasch analysis, the participants’ underlying perceived agreeability toward the levels of accuracy and comfort of the hand-gesture–based commands were estimated based on the ratings that they gave to the total number of commands they performed (7 commands repeated trice). Item difficulty measure refers to the Rasch estimate of the level of difficulty of the item (ie, hand-gesture–based command). The measurement of the difficulty of each item was calibrated from the total ratings given to the respective hand-gesture–based command.
Figures 2 and 3 present the Rasch person-item map illustrating the relationship between participants’ agreeability to the accuracy and comfort levels of the hand-gesture–based command ( left columns ) and the level of accuracy and comfort of each hand-gesture–based command ( right columns ) on a common logit scale ( central bar ). As a general rule, the mean item difficulty estimate is centered on 0 logits. Participants and items were arranged according to their logit measurements so that the most affirmative person (who gave high ratings to the items) and the most difficult item (which received the lowest ratings) are on the upper section of the map, and the least affirmative person and least difficult item are on the lower section of the map. Thus, the higher the participant is on the map, the more likely he or she would have been to endorse the accuracy or comfort level of the hand-gesture–based commands. Conversely, the lower the item is on the map, the more likely the item would have been to be endorsed by the participants as accurately and comfortably performed.
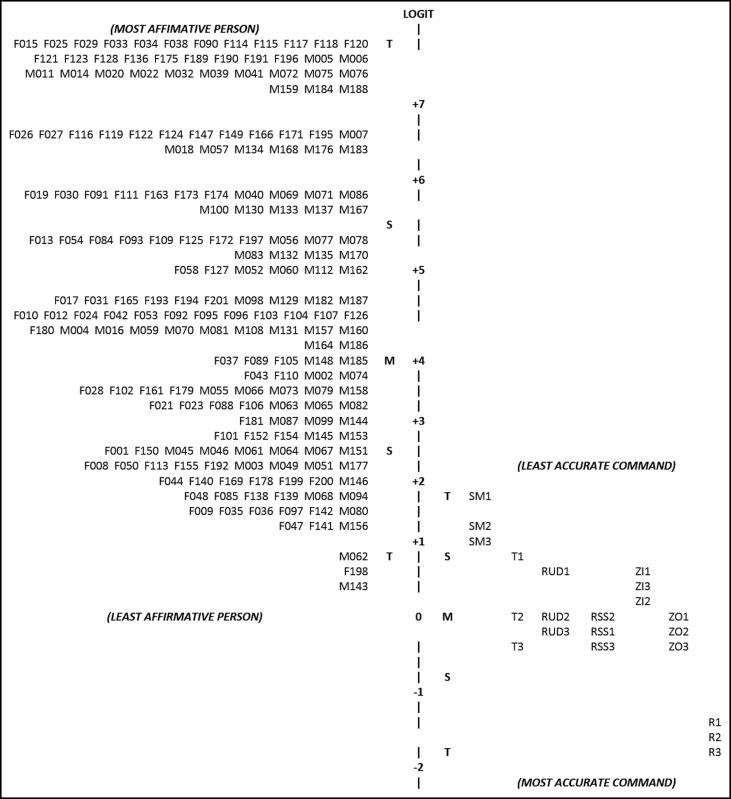
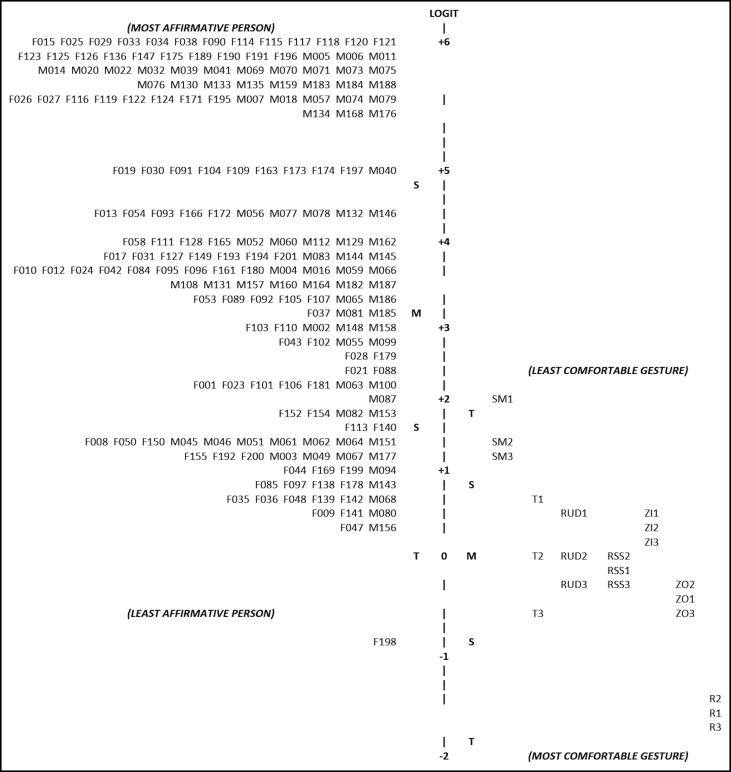
Figure 2 on accuracy demonstrates the spread of the participants (logit range, 7.69 to 0.35) and the corresponding commands (logit range, 1.76 to −1.68). Most participants (92.0%) had logit scores that were higher (≥1.79) than all commands, indicating that a large proportion of the participants affirmed that they were able to perform the hand-gesture commands accurately. In Figure 3 on comfort level, the distribution also showed that most participants (logit range, 6.97 to −0.75) had higher logit scores than the commands (logit range, 2.09 to −1.69). More than 3 quarters of the participants (77.6%) had logit scores of 2.14 and above. Similarly, this signified that they affirmed that the hand gestures were comfortable for most if not all commands. The reset command had logit scores that were lower than all of the participants’ logit values at all 3 attempts (−1.49 to −1.68 for accuracy, and −1.53 to −1.69 for comfort), showing that it was perceived to be the most accurate command and the most comfortable hand-gesture–based command to perform. In contrast, select menu was considered the least accurate (1.76 to 0.95) and the least comfortable (2.09 to 1.21) because it had the highest logit scores among the 7 commands. The other hand-gesture–based commands were perceived to be within 1 SD of the mean item difficulty estimates for both accuracy and comfort.
Figure 4 , A and B , present the category probability curves for the accuracy and comfort level rating scales, respectively. The diagrams show the predicted probability of each participant’s response, which is based on the difference between the logit measure estimates of the person and the item of interest. This information aided in determining the probable rating for each item by the most and least affirmative persons.
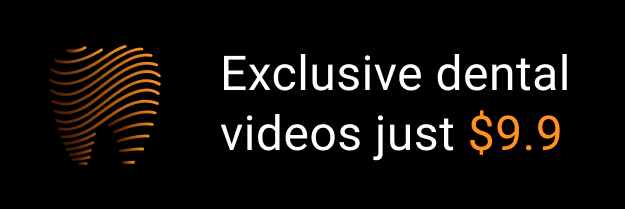