When first case examples presents to a dentist, a patient may have a specific complaint, be in need of routine evaluation, or arrive on referral from another health care provider. In all cases, proper diagnosis of existing problems is the essential first step in provision of appropriate oral health care. The clinician’s approach to diagnosis and the need to arrive at the appropriate diagnosis are daily challenges in dental practice. This article discusses the prescriptive and descriptive theories of diagnostic reasoning using 4 case examples.
When first presenting to a dentist, a patient may have a specific complaint, be in need of routine evaluation, or arrive on referral from another health care provider. In all cases, proper diagnosis of existing problems is the essential first step in the provision of appropriate oral health care. The clinician’s approach to diagnosis and the need to arrive at the appropriate diagnosis are daily challenges in dental practice. This challenge can be daunting given the complex anatomy of the oral cavity and its contiguous structures. Please consider the following case:
Case 1
A 42-year-old woman was referred by her physician for evaluation of a painful lesion on her tongue. This discomfort was associated with an ulcerated area on the lateral border ( Fig. 1 ). The lesion had been present for 6 weeks, and the patient consulted her dentist. As the lesion persisted, the patient began having difficulty eating and also developed hoarseness. The patient reported using an over-the-counter topical medication containing benzocaine to treat the lesion. She was otherwise well and was not using any medications.
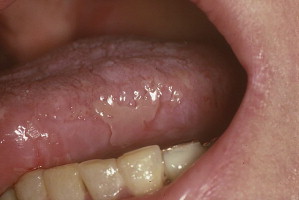
Initially, the dentist suspected several possibilities, but the leading diagnoses were primary herpetic gingivostomatitis and an aphthous ulcer. Other possible diagnoses included an autoimmune disorder and infection (syphilis or tuberculosis). The lesion did not have an erythematous base, making herpetic gingivostomatitis less likely. Certain characteristics of the lesion, primarily the persistence and severity of the ulceration, helped in establishing a diagnosis. The voice change, although not diagnostic, was also suggestive of an autoimmune disease that can affect the larynx. Pemphigus vulgaris was suspected, and a biopsy of the lesion was performed.
The results of the biopsy revealed an intraepithelial split. Direct immunofluorescence was positive within the epithelium. The biopsy results were consistent with pemphigus vulgaris, and the patient was ultimately treated with intraoral topical steroids. Close observation for the appearance of other lesions on the skin, and other symptoms suggestive of more advanced disease, was also recommended.
Prescriptive theories
Decision making had been a topic of study in cognitive psychology, philosophy, and mathematics for several decades before it became a topic of scholarly interest in clinical care. Within the field of clinical decision making, the initial wave of research was focused on developing rational or prescriptive approaches to diagnostic reasoning. Researchers sought to explore how practitioners can make the best and most rational diagnostic decisions.
Von Neumann and Morgenstern were among the first to publish in the area of decision sciences with their application of expected utility theory. They argued that people’s decisions are based on an individual’s assessment of the benefits of choices modified by personal preference, the probability of possible outcomes, and the assessment of and avoidance of risk. Decision analysis emerged as an extension of expected utility theory in which multistep decision making is analyzed. In decision analysis, each diagnostic choice is represented as a node or branching point on a decision tree. Each node is associated with risks, benefits, and utilities for each possible decision. Theoretically, given this set of assumptions, the clinician can proceed rationally through the possible diagnostic choices for a specific clinical situation and arrive at the best possible outcome. One application of decision analysis is in the development of diagnostic decision trees. Thus, if available, the dentist encountering the patient with an oral ulceration (case 1) might reason through the diagnostic possibilities and the need for further workup using a decision tree based on a rational assessment of the clinical factors and the utility of the clinical choices.
Case 2
A 54-year-old man presented to his dentist with a complaint of pain when chewing on the left side. His medical history included a history of stress, which requires occasional use of the anxiolytic alprazolam (Xanax), otherwise being noncontributory.
The intraoral examination demonstrated a slight swelling on the buccal mucosa at the midpoint of tooth No. 30. The examination also revealed a maintained dentition, with the posterior teeth restored with amalgam restorations and crowns. There was mild to moderate wear of the cusps of the posterior teeth.
Radiography of the mandibular left posterior sextant revealed that tooth No. 30 was treated endodontically, that a post was present in the distal canal, and a large radiolucency was present in the furcation area ( Fig. 2 A). The radiolucency was not localized to the area of the post and extended beyond the apices of both roots. Radiography also revealed that the endodontic obturation was inadequate for both roots. No obvious fracture was observed.
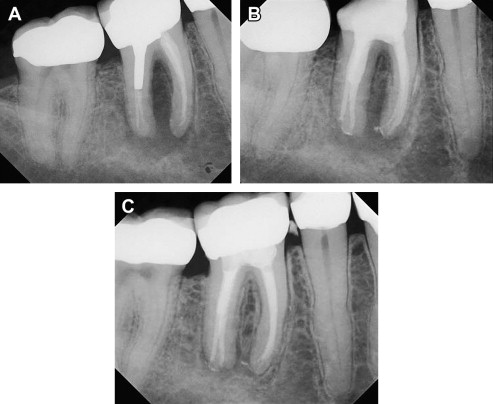
The differential diagnosis in this case included an inadequate endodontic obturation, a root perforation related to the post, as well as a localized periodontal lesion. Given the patient’s history of anxiety and bruxism, a fractured tooth was included in the differential diagnosis.
Before obtaining the radiograph, the clinician thought that there was an intermediate probability of a fractured tooth. Once the radiograph was available, the clinician must consider the likelihood of a fracture. As mentioned, the radiograph did not demonstrate an obvious fracture. How likely is it that the patient has a fractured tooth, given this finding? The application of Bayes theorem, a statistical rule for combining probabilities, can be helpful in appreciating how information from additional testing affects the likelihood of a particular condition.
The following basic formula describes the concept of Bayes theorem:
Most clinicians can appreciate pretest chances of a disease being present, when it is described as a probability. For instance, if the dentist were to estimate the likelihood of a fractured tooth at 60% before obtaining the radiograph, he or she is describing a probability. The pretest probability refers to the clinician’s estimation of the chances that the patient has a given problem before an additional test is performed. In this case, that additional test is radiography. The pretest probability is based on several factors, including the prevalence of the condition in the patient’s demographic group, the patient’s history, clinical examination, and results from previous diagnostic tests. It is to be noted that this estimation is partly subjective and, thus, may vary from dentist to dentist. In this case, because of the history and physical findings, the clinician might have an intermediate suspicion for a fracture before the radiograph is obtained, estimating the pretest probability at 60%. To use Bayes theorem, however, this pretest probability must be converted to pretest odds. A pretest probability of 60% is converted to pretest odds of 1.5 using the following formula:
The next variable to consider in the Bayes equation is the likelihood ratio. The likelihood ratio reflects how much a positive or negative finding changes the odds of having a given condition. It is a variable that is based on the performance characteristics of the test being used, combining both the sensitivity and specificity values of a test. These test performance characteristics, the sensitivity and specificity, must be available to the clinician in order to use Bayes equation. In this case, the clinician performed a quick literature search to locate a study that reported the sensitivity and specificity of (digital) radiography of detecting a fractured tooth as 86% and 85%, respectively. The sensitivity can be thought of as the proportion of positive test results among all those patients with the disease. Specificity is the proportion of negative test results among those who do not have the disease. These concepts are represented in Table 1 .
Fractured Tooth Present | Fractured Tooth Absent | |
---|---|---|
Radiograph + | A | B |
Radiograph − | C | D |
Sensitivity is calculated as A/A + C and is a measure of how often disease is identified by the test in a total population with the disease. Specificity is calculated as D/B + D, and is a measure of how often the disease-free state is identified amongst those without the disease.
A likelihood ratio is the likelihood that a given test result would be expected in a patient with the disorder of interest compared with the likelihood that the same result would be expected in a patient without the disorder. The sensitivity and specificity of the test of interest can then be combined to calculate the positive and negative likelihood ratios.
The likelihood ratio of a positive test result (LR+) is calculated as
The likelihood ratio of a negative test result (LR−) is calculated as
Using 0.86 and 0.85 as sensitivity and specificity values, respectively, LR+ is 5.7 and LR− is 0.16.
With an intermediate pretest odds of 1.5, a negative test results in a posttest odds of 0.24 (1.5 × 0.16 = 0.24). This posttest odds can then be converted to posttest probability using the following formula:
This calculation results in a posttest probability of 0.19 or 19% of having a fractured tooth. The absence of an identifiable fracture in the radiograph lowered the probability of fractured tooth from intermediate to low. The test result, in this case, affected the overall clinical impression, and given these findings, the clinician chose to re-treat the tooth endodontically (see Fig. 2 B). The clinical swelling resolved, and the postoperative radiograph indicated increased density of the bone in the furcation area (see Fig. 2 C). On the other hand, if the radiograph had shown a fracture, using a LR+ of 5.7, the posttest odds become 8.55, with a resultant posttest probability of 0.90 or 90%. In such a scenario, the practitioner might have chosen to extract the tooth. Thus, in a clinical scenario with an intermediate pretest probability and a diagnostic test with fairly robust test characteristics (high sensitivity and specificity), a test result can have a large effect on the final impression.
It is worth considering the effect of the test result when the pretest probability is very high or very low. If a high pretest probability of 95% or 0.95 is assigned, a positive test result would increase the posttest probability to 0.99 or 99% and a negative test result would lower the probability to 0.75 or 75%. If the pretest probability had been very low, at 0.05 or 5%, a negative test result would reduce the posttest probability to 0.01 or 1% and a positive test result would only increase the probability to 0.22 or 22%.
Two lessons can be gleaned from this scenario. First, the pretest probability, or the patient’s history and the clinical examination, modifies the effect of subsequent testing on the final clinical impression. Second, in situations with a very low or very high pretest probability, further testing is not likely to change the clinical impression and may actually lead to false-positive or false-negative conclusions. Even when the sensitivity and specificity of a diagnostic test are fairly high, the test is most valuable in scenarios with intermediate pretest probabilities. Thus, an understanding of bayesian analysis can help the dentist estimate the effect of a diagnostic test on the final clinical impression. In some cases, this understanding might help the clinician decide whether or not to obtain a diagnostic test in the first place.
Limitations of Prescriptive Theories
The use of decision analysis, decision trees, bayesian analysis, and other normative approaches to diagnosis have their limitations, although they are logical and rational tools. For instance, when using bayesian analysis, one must first describe a pretest probability. As mentioned, this estimation is ultimately a clinician’s subjective assessment of the likelihood of disease. One practitioner’s estimation of the pretest probability of a fractured tooth may differ from another’s. The use of bayesian approach is also best suited for a diagnostic test that has a binary set of outcomes, with a positive or negative test result. It becomes problematic when a test result is more ambiguous or measured on a continuous scale (not dichotomous). How should the practitioner apply Bayes theorem when the test result is not conclusively positive or negative; for instance, when the radiograph suggests rather than definitively demonstrating a fracture? Some have argued that arriving at mathematically calculated posttest odds of disease might misleadingly imply a level of precision and scientific certainty to the diagnostic endeavor, which is ultimately subjective. Furthermore, the use of these normative strategies might require the oversimplification of often highly complex clinical scenarios.
The use of decision analysis to understand diagnostic reasoning also has its limitations. Although it seems reasonable to think that clinicians weigh each decision node in a logical fashion, as might be represented by a decision tree, this might not actually reflect how clinicians think in clinical settings. Rather, descriptive literature on diagnostic reasoning reveals that clinicians are often more likely to draw on past experience to make diagnoses and less frequently on carefully constructed logical pathways. Clinicians are sometimes resistant to using decision trees and may consider algorithmic approaches to diagnosis to be overly simplistic. Clinicians might also not think logically about utilities and probabilities. There is evidence that practitioners tend to overvalue risk and possible harm over possible benefits from a given decision. These behaviors are not limited to novice clinicians; even expert clinicians are noted to make decisions that are not in keeping with the logical decisions anticipated by decision analysis theories.
It is possible that these normative diagnostic approaches are not always followed in practice because they have significant costs. For instance, the application of bayesian analysis, evidence-based dentistry, or a rational approach suggested by decision analysis requires time, investment of cognitive effort, and access to real-time information. In practice, the clinician might not have the time, energy, or information needed to formally explore the diagnostic process in the way that is necessary in these approaches. Despite these limitations, later in this article, the use of prescriptive theories of diagnostic reasoning is considered as a solution to some of the pitfalls encountered in typical diagnostic thinking.
Prescriptive theories
Decision making had been a topic of study in cognitive psychology, philosophy, and mathematics for several decades before it became a topic of scholarly interest in clinical care. Within the field of clinical decision making, the initial wave of research was focused on developing rational or prescriptive approaches to diagnostic reasoning. Researchers sought to explore how practitioners can make the best and most rational diagnostic decisions.
Von Neumann and Morgenstern were among the first to publish in the area of decision sciences with their application of expected utility theory. They argued that people’s decisions are based on an individual’s assessment of the benefits of choices modified by personal preference, the probability of possible outcomes, and the assessment of and avoidance of risk. Decision analysis emerged as an extension of expected utility theory in which multistep decision making is analyzed. In decision analysis, each diagnostic choice is represented as a node or branching point on a decision tree. Each node is associated with risks, benefits, and utilities for each possible decision. Theoretically, given this set of assumptions, the clinician can proceed rationally through the possible diagnostic choices for a specific clinical situation and arrive at the best possible outcome. One application of decision analysis is in the development of diagnostic decision trees. Thus, if available, the dentist encountering the patient with an oral ulceration (case 1) might reason through the diagnostic possibilities and the need for further workup using a decision tree based on a rational assessment of the clinical factors and the utility of the clinical choices.
Case 2
A 54-year-old man presented to his dentist with a complaint of pain when chewing on the left side. His medical history included a history of stress, which requires occasional use of the anxiolytic alprazolam (Xanax), otherwise being noncontributory.
The intraoral examination demonstrated a slight swelling on the buccal mucosa at the midpoint of tooth No. 30. The examination also revealed a maintained dentition, with the posterior teeth restored with amalgam restorations and crowns. There was mild to moderate wear of the cusps of the posterior teeth.
Radiography of the mandibular left posterior sextant revealed that tooth No. 30 was treated endodontically, that a post was present in the distal canal, and a large radiolucency was present in the furcation area ( Fig. 2 A). The radiolucency was not localized to the area of the post and extended beyond the apices of both roots. Radiography also revealed that the endodontic obturation was inadequate for both roots. No obvious fracture was observed.
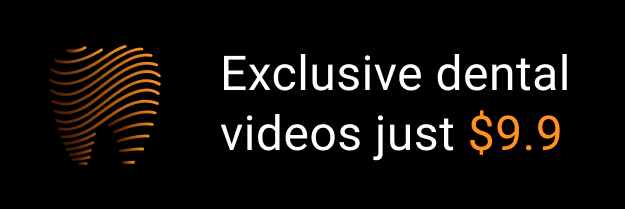