Acommon question in orthodontic research is “how many patients do I need for my study?” The next articles will introduce relevant concepts that will help readers to understand how to appropriately plan the size of a trial.
The objective of a clinical trial is to provide reliable evidence regarding the effect or no effect of a treatment modality. A sufficient number of participants allows the researcher to detect a difference with reasonable precision (good power) if a difference exists, or allows one to be reasonably certain that no difference exists if the results show no difference. Small studies tend to be less convincing and inconclusive because they often have low power. Recruiting more patients than necessary is a waste of resources and even unethical, since more patients than necessary could be exposed to a potentially ineffective therapy. There is a close relationship between power and sample size; usually, as the sample size increases, study power is also expected to increase. Ideally, a balance between study power, a clinically important difference to be detected, trial feasibility, and credibility are required.
What is study power? Power is the probability of observing a difference between treatment groups when a difference exists. A study designed to detect a clinically important difference with, let’s say, a power of 80% assumes an 80% chance of observing a difference if there is a difference, and also assumes a 20% chance of missing the difference (false negative) when such a difference exists. Allowing a 20% (power 80%) or a 10% (power 90%) chance of a false negative (type II error or beta) is unavoidable, since a sample calculation with 100% power (type II error approaching zero) would require an infinite number of participants. Type I error, or α or alpha, refers to false-positive results and indicates that we are willing to accept a 5% (α = 0.05) chance of observing a statistically significant difference when no such difference exists between the treatment groups. See Table I for descriptions and relationships of error types and power.
Result of significance test | In reality, no difference exists | In reality, a difference exists |
---|---|---|
Not significant | 1 – α (= 0.95 or 95%) Correct conclusion, accepting the null hypothesis (Ho) when the Ho is true |
β or type II error (= 0.20 or 20%) β = 1 – power Incorrect conclusion, rejecting the alternative hypothesis (Ha) when the Ha is true |
Significant | α (= 0.05 or 5%) or type I error α = level of significance Incorrect conclusion, rejecting the Ho when the Ho is true |
1 – β (= 1 – 0.20 = 0.8 or 80%) 1 – β = power Correct conclusion, rejecting the Ho when the Ha is true |
In this article, we will perform a sample calculation for a normally distributed quantitative outcome for a 2-arm trial with 1:1 allocation ratio (2-sided test). Sample calculations are based on assumptions, and we should aim to detect differences between treatment groups, if they exist, that have clinical importance rather than statistical significance.
Before we proceed with the sample calculation, we need to define the following.
- •
The research question.
- •
The principal outcome measure of the trial.
- •
μ1, the anticipated mean response for the standard or control treatment.
- •
μ2, the anticipated mean response for the alternative treatment and hence the minimum clinically important difference (μ2 – μ1) between treatment arms that we would like to detect.
- •
The standard deviation (for continuous outcomes only).
- •
The degree of certainty with which we want to be able to detect the treatment difference (power) and the level of significance (type I error or α).
We will use an example trial to illustrate the process. Pandis et al, in a study assessing treatment time to alignment and dental changes between self-ligating and conventional appliances, found that the molar width difference at the end of the follow-up period was 2 mm (SD, 2 mm), a statistically significant finding ( Table II ). This study was not randomized, and the authors used different wires. Was the 2-mm difference in molar width genuine or was it observed because wires of different shapes were used for the treatment groups? We would like to confirm or refute those findings by adopting a randomized control trial design and using exactly the same wire shape and sequence for both treatment groups. As it was previously explained, to perform the sample calculation, we would need to decide what would be a clinically important difference that we want to detect. We can refer to the previous study and can assume that a molar width difference of 2 mm between the 2 appliances at a certain time after treatment initiation has clinical importance. Then we can design a randomized control trial with 90% power and a 5% level of significance, which will detect a 2-mm difference between the treatment groups if such a difference really exists. Therefore, μ2 – μ1 = 2 mm, power = 90%, and α = 0.05, and let us assume that the standard deviation (σ) is 2 mm for both treatment arms by also referring to the cited study. We will use the following formula for 2 means from Pocock.
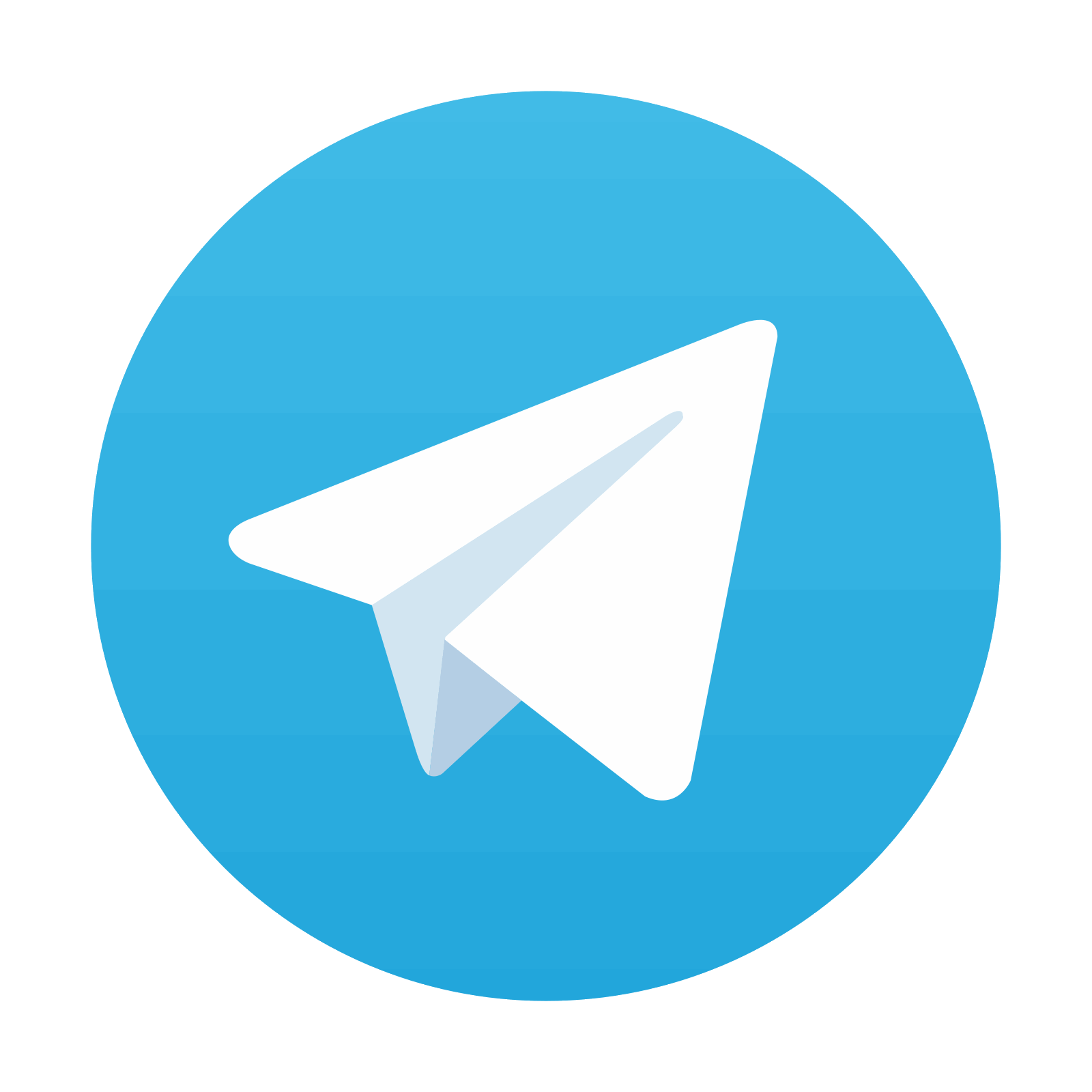
Stay updated, free dental videos. Join our Telegram channel

VIDEdental - Online dental courses
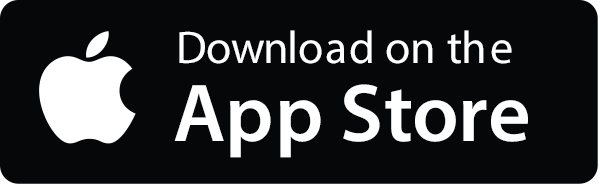
