In the previous articles of this column , , we introduced 2 important analyses as a mean to investigate statistical heterogeneity in a meta-analysis: subgroup analysis and meta-regression analysis. A subgroup analysis is performed when the characteristic of interest is a categorical variable (eg, design of the trial as randomized controlled trial or clinical controlled trial). A meta-regression analysis is performed when the characteristic of interest is a metric variable (eg, sample size of the trials). Before applying a subgroup analysis or meta-regression, there are some important parameters to consider, such as the number of studies included in a meta-analysis, the selection of the covariates, and the number of covariates. Lack of careful planning and proper implementation of the subgroup analysis and meta-regression can cause crucial problems that might lead to erroneous conclusions. In the following text, we outline the most common problems and pitfalls in both analyses.
The findings of subgroup analysis and meta-regression should be interpreted with caution because of their observational nature. Although patients are randomly allocated to 1 intervention or another within a clinical trial, they are not randomly “allocated” across the trials included in the subgroup and meta-regression analyses. Therefore, subgroup analysis and meta-regression suffer from the same problems and pitfalls as observational studies, such as confounding. ,
Under confounding, an association might not be casual when another (known or unknown) covariate is associated with both the covariate of interest and the treatment effects. , For example, the impact of mechanical against electric toothbrush on dental caries can be confounded by sugar consumption. Confounding is common in subgroup analysis and meta-regression because some covariates might be correlated with each other. Confounding should not be ignored because it complicates the interpretation of the subgroup analysis and the meta-regression and can lead to misleading conclusions.
A subgroup analysis with many subgroups might lead to false-positive results. If a characteristic has k independent subgroups, the probability of observing at least 1 significant result by chance is 1−(1− a ) k , where a is the level of significance. For example, if k =4, the probability of a false positive is almost 19% and not 5% as the α level of statistical significance usually suggests. It is essential to avoid multiple applications of the subgroup analysis because it is likely to observe a significant result only by chance. Misleading results increase the risk of treating patients with an ineffective (or even a harmful) intervention or omitting a truly effective intervention.
Subgroup analysis and meta-regression will have low power to detect statistically significant associations when there is a small number of studies. Even if a subgroup analysis or a meta-regression contains only a few studies with many patients, testing can be underpowered. A typical guide for the implementation of meta-regression is to include 10 studies for each covariate. A larger number of smaller studies is likely to yield more power than a small number of large studies.
Patient characteristics are summarized at the level of a study, such as average age, and the proportion of female subjects. Some patient characteristics might vary within the studies rather than among the studies. The age of the patients is a common example. There can be a strong association between age and size of the intervention effect within each study. By contrast, if the average age is similar across the studies, there will be no association between the average age and the effect sizes. Therefore, an association between a patient characteristic and the effect at the patient level does not necessarily imply an association between this summarized patient characteristic and the effect sizes at the study level. This paradox is known as aggregation bias, ecological bias, or ecological fallacy.
To determine whether aggregation bias exists, we need to investigate individual patient data, namely, data on the investigated outcome and important characteristics as measured in all randomized patients in each included study. Consider that we have available 6 studies in a meta-analysis and are interested in examining the impact of 2 interventions on nickel hypersensitivity. The effectiveness of the interventions is measured in the risk ratio scale. There is evidence from other studies that the age of the participants tends to influence the effectiveness of the interventions for nickel hypersensitivity. Figure 1 is a scatter plot of these 6 trials. The size of the circles corresponds to the weight of the studies; the larger the sample size, the larger the circle. The association between the age and the effect size at the patient level is reflected by the slope of the black lines in each study. The association between the average age and the effect size at the study level is indicated by a red dotted line. As shown in Figure 1 , the effect size is positively related to the age in each study; as the participants’ age increases, so does the risk ratio. By contrast, there seems to be no association between the average age and the effect sizes at the study level. Figure 2 depicts the 6 studies of the aforementioned example, but it shows different results. Now, the intervention effect is positively related to the average age across the studies and not to the age of the participants within each study.
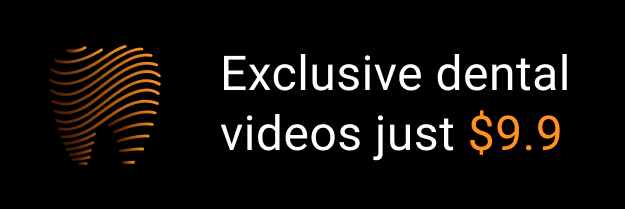