In the previous article, I discussed linear regression using a single independent continuous variable.
In linear regression analysis, we can include more parameters in an effort to find factors that better predict an outcome. This analysis is known as multiple linear regression analysis. Additionally, multiple linear regression analysis can be used to adjust for confounders. In randomized controlled trials, confounding is minimized by randomization, but weak confounding might still be present because of small imbalances of outcome predictors.
In a multiple regression model, we can add a mixture of continuous and categorical predictors as well as interaction terms among categorical predictors, or among categorical and continuous predictors, to assess simultaneously the combined effect of those parameters on the outcome.
To the previous simple linear regression in which the effect of initial crowding on days to alignment was assessed, we can add sex to see whether it is a significant outcome predictor. The Table shows that in the adjusted model, initial crowding remains a significant predictor ( P = 0.001), whereas sex shows a nonsignificant association ( P = 0.62) with days to alignment. The interpretation is as follows: for boys compared with girls, it will take on average 6.7 days more to reach alignment after adjusting for initial irregularity. This difference ranges from about 20 (−19.89) days less to 33 (33.24) days more, a statistically nonsignificant finding ( P = 0.62). This model can be also described by using this equation:
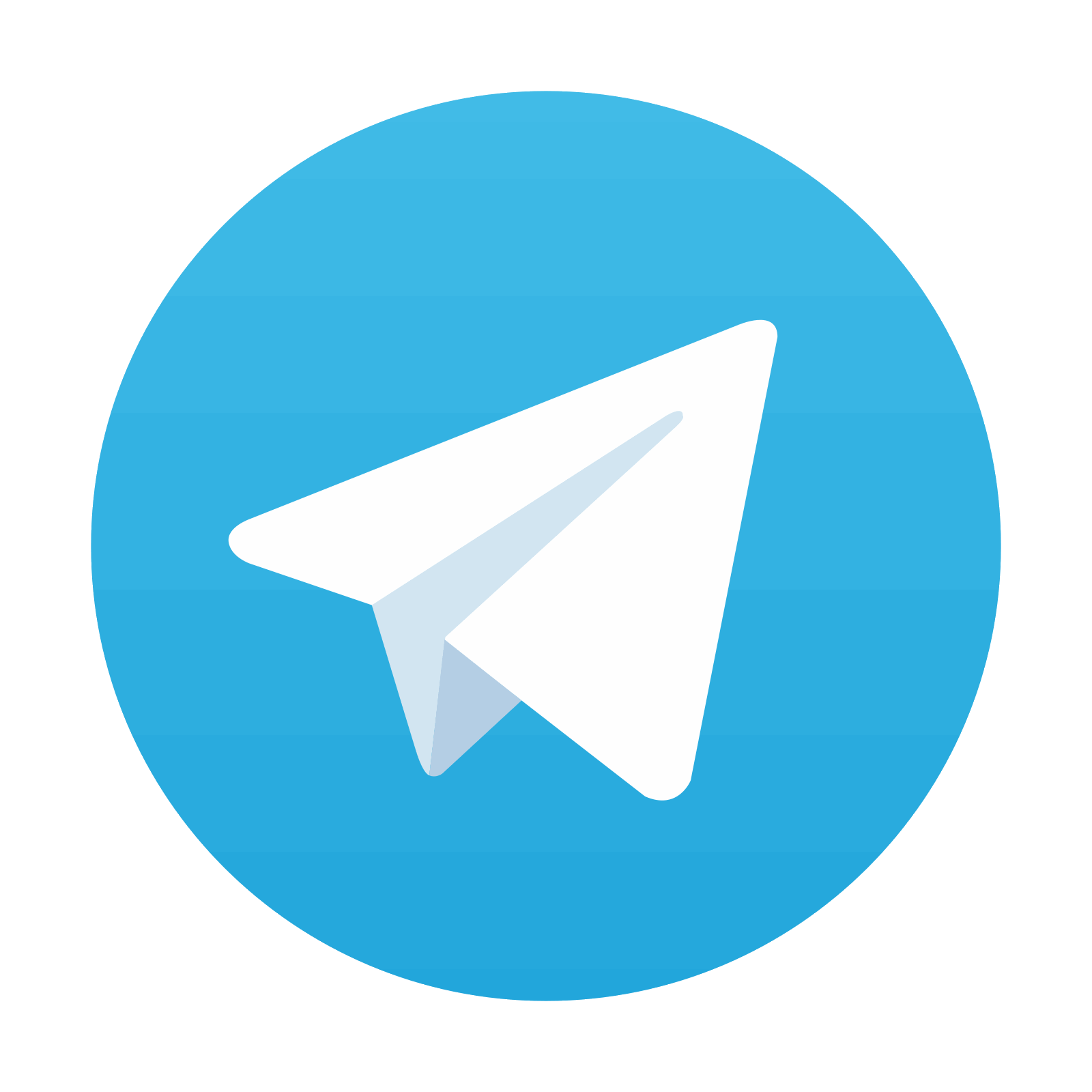
Stay updated, free dental videos. Join our Telegram channel

VIDEdental - Online dental courses
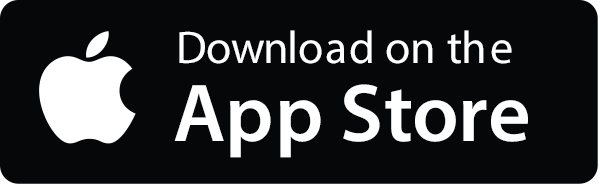
