Under the random-effects model, the true effect size is assumed to be different across studies. These studies are not identical (as in the case of the fixed-effect model), but they have enough in common to be included in the meta-analysis and synthesize their information. For example, we are interested in identifying studies that examine the clinical effectiveness, such as bond failures of plasma vs halogen curing lights. We randomly sampled patients from different practices (also randomly selected). If plasma light is actually more effective than halogen, we expect the effect size (eg, risk ratio [RR] or odds ratio [OR]) to be similar but not identical across the practices. Because these practices can differ in their populations (ie, crowding severity, cooperation, age, and sex), implementation of interventions (ie, practitioner expertise, adhesives, and type of brackets used), outcomes (duration of follow-up), and settings (geographic area), among other reasons (known as covariates), the magnitude of the effect size is likely to vary across the studies; the effect size may be lower in practices with more expertise and older participants and vice versa. In practice, the existence of such covariates that affect the effect size is innate and leads to variations in the magnitude of the effect.
The random-effects model addresses the variations in the effect size across the studies beyond mere chance. According to this model, there is not a common effect size, but true study-specific effect sizes that are normally distributed about a mean (known as mean effect size) with variance that reflects the variation of these effect sizes. Our goal was to estimate the mean and the variance of this distribution. The summary effect size is actually the mean effect size. Because in the random-effects model we study a sample of true effect sizes and not a common one, we use the plural (effects). Figure 1 presents a forest plot of 4 fictional studies under the random-effects model. In the random-effects model, each study provides a different effect size because of both within- and between-study variances (the latter implies the degree of dissimilarity of the included studies), unlike in the fixed-effect model in which only within-study variation is expected. Therefore, the weighting scheme of this model is based on 2 sources of variance in each study. The detailed steps to perform a random-effects meta-analysis are provided in the Supplementary Appendices 1 and 2 .

Application to real data—binary outcome
The application is based on the data set we used for the fixed-effect meta-analysis ( Table I ). The results under the random-effects model are almost the same (and slightly less precise) as those under the fixed-effect model (random-effects: RR = 0.92 with 95% confidence interval [CI] = 0.70-1.21 vs fixed-effect: RR = 0.90 with 95% CI = 0.69-1.19). This trivial difference was expected because the between-study variance was found to be trivial. The results of this worked example are illustrated in Figure 2 . To simplify this example, clustering owing to multiple teeth bonded within patients was not considered during this analysis. Accounting for clustering would have increased the width of the 95% CIs, and hence the uncertainty of the estimates with no difference in the conclusions presented here.
Study | Plasma | Halogen | ||||
---|---|---|---|---|---|---|
Events | Nonevents | Sample size | Events | Nonevents | Sample size | |
Cacciafesta, 2004 | 21 | 279 | 300 | 12 | 288 | 300 |
Manzo, 2004 | 12 | 292 | 304 | 12 | 292 | 304 |
Pettemerides, 2004 | 12 | 164 | 176 | 13 | 163 | 176 |
Russell, 2008 | 22 | 305 | 327 | 31 | 296 | 327 |
Sfondrini, 2004 | 31 | 686 | 717 | 39 | 678 | 717 |
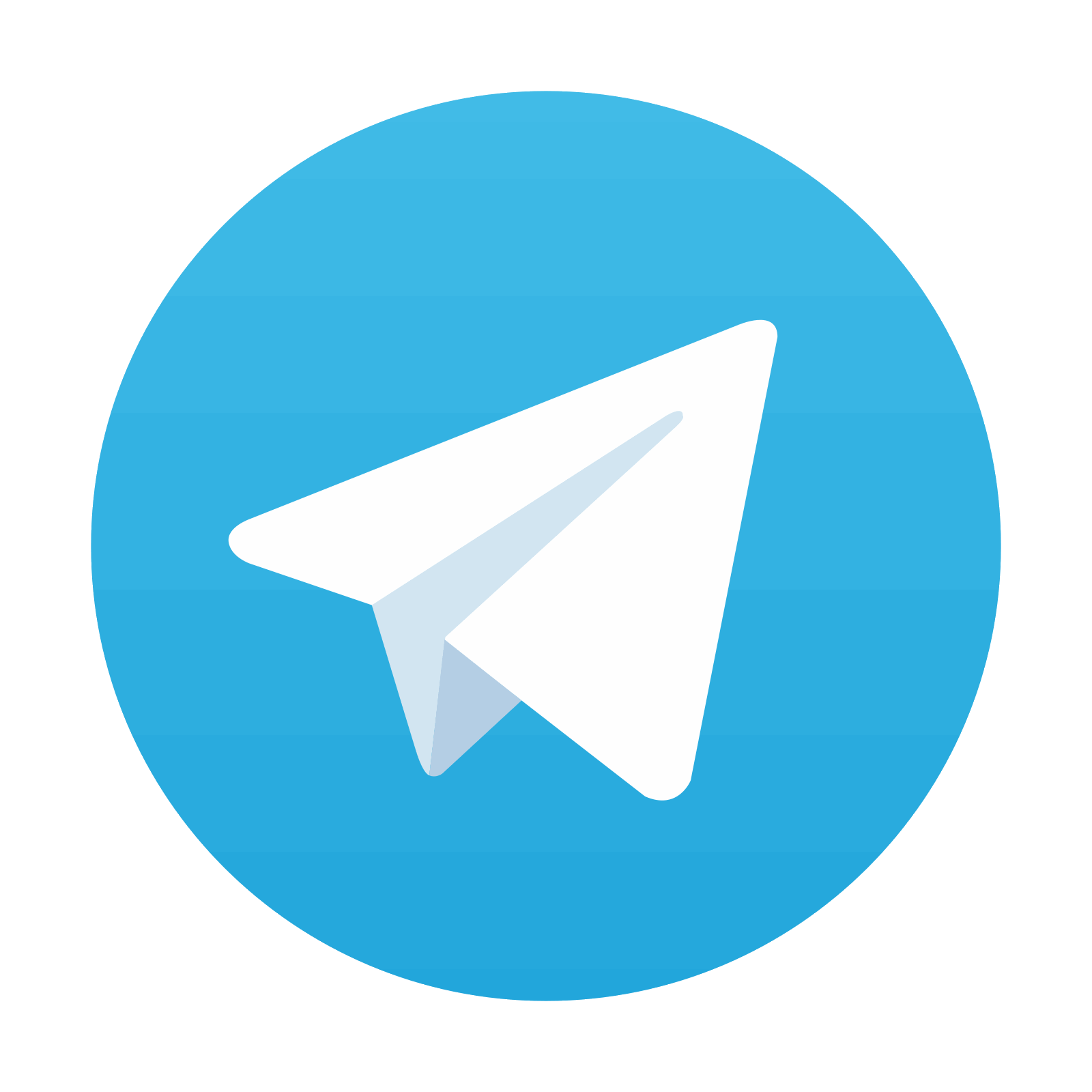
Stay updated, free dental videos. Join our Telegram channel

VIDEdental - Online dental courses
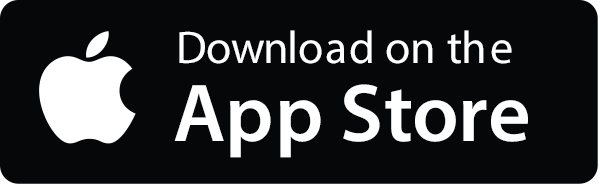

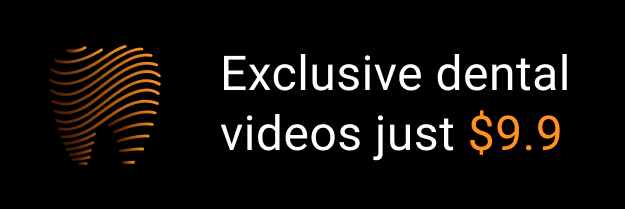