In the next series of articles, I will discuss correlation and linear regression.
Correlation indicates whether there is any association between 2 quantitative variables and the strength of that association. Linear regression is a statistical tool that allows us to investigate the relationship between a causal variable and a variable of interest: eg, the effect of the amount of pretreatment crowding (causal variable) on the number of days required to reach alignment (variable of interest).
Example
We will investigate the effect of the amount of pretreatment crowding on the number of days required to reach alignment. Days to alignment is a continuous variable expressed in days, and the irregularity index is also a continuous variable expressed in millimeters. The assumption is that the greater the initial crowding, the longer it will take to align the dentition. Table I gives summary information of the 2 variables.
Variable | Observations | Mean | SD | Minimum | Maximum |
---|---|---|---|---|---|
Irptx | 74 | 6.96 | 0.82 | 5.26 | 8.63 |
Days to align | 74 | 150.97 | 38.86 | 88 | 242 |
The first step is to see whether the 2 variables are correlated. We can assess this using the Pearson correlation coefficient r (also termed product moment correlation coefficient), which expresses the strength of the linear relationship between 2 variables, and it takes values from −1 to 1.
If the correlation coefficient is −1 or +1, then the points in a scatter plot will lie exactly on a straight line, indicating a strong correlation between the variables. The correlation is positive if higher values of one variable are associated with higher values of the other variable, but the points do not have to lie exactly on a straight line. The correlation is negative if the values of one variable decrease as the values of the other variable increase. Again, the points do not have to lie exactly on a straight line.
If there is no linear relationship, then the correlation is zero, and the points in the plot are randomly scattered. However, a nonlinear relationship does not necessarily entail no association between the variables. These variables might have, for example, a quadratic relationship that is represented by a parabola (U-shaped curve). Therefore, you should always examine the data graphically first. One problem with r is that it tends to be smaller when the range of one variable is restricted; this makes comparisons between different studies difficult.
A strong correlation between variables does not imply that one has a causal effect on the other, since many variables rise and fall over time, and thus are correlated. For example, as ice cream consumption increases, the risk of death due to drowning increases. We cannot infer that ice cream consumption causes drowning.
To apply the Pearson r, the variables must be normally distributed (even approximately). When normality does not hold, then the Spearman rank order correlation coefficient (a nonparametric correlation coefficient) can be used. The Spearman correlation works on the ranks of the variables.
Table II shows that the 2 variables are correlated. The strength of this association is represented by the correlation coefficient r = 0.9460, and it is considered a strong association. The Figure clearly shows that as pretreatment crowding increases, so does the number of days to reach alignment.
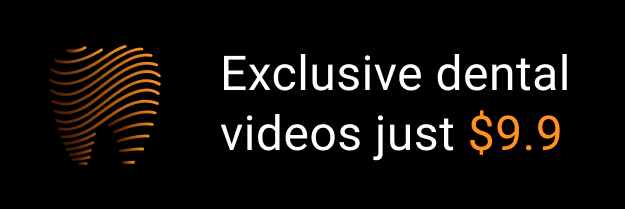