Introduction
The objective of this network meta-analysis was to synthesize the evidence of the comparative effectiveness for various interventions used for orthodontic pain relief during peak pain intensity.
Methods
MEDLINE, EMBASE, and Cochrane Central Register of Controlled Trials databases were searched to December 31, 2014, to identify relevant studies. Additional studies were found by hand searching of journals and reference lists. Unpublished articles were also searched. Eligible studies were randomized controlled trials evaluating the effectiveness of pharmacologic or nonpharmacologic interventions for pain relief after placement of separators or an initial aligning archwire. A covariate-adjusted arm-based 3-level hierarchical Bayesian random-effects model was used for this network meta-analysis.
Results
Twenty-four randomized controlled trials (2273 participants; 997 male, 1276 female; mean age, 18.2 years; SD, 4.4 years) were included in this network meta-analysis. A total of 26 interventions were identified and classified into 6 classes based on their mechanism of action. Compared with placebo-class, nonsteroidal anti-inflammatory drug analgesics and lasers were the most effective intervention classes with a shared median rank of 2 (95% credible interval [CrI], 1-3), followed by “other” analgesics (median rank, 3; 95% CrI, 1-4), behavior therapy (median rank, 4; 95% CrI, 3-6), and miscellaneous (median rank, 5; 95% CrI 3-6). The most effective individual interventions in the nonsteroidal anti-inflammatory drug analgesics and lasers classes were etoricoxib (median rank, 1; 95% CrI, 1-3) and gallium-arsenide superpulsed lasers (median rank, 3; 95% CrI, 1-13), respectively. Assessment of transitivity and consistency assumption showed no threat to the network meta-analysis estimates. There was no evidence of significant publication bias. Heterogeneity was mild to moderate (tau-square, 0.044; 95% CrI, 0.040-0.055).
Conclusions
The results show that analgesics and lasers are effective in the management of orthodontic pain at its peak intensity. Further research is required to improve the quality of evidence, especially for analgesic interventions.
Highlights
- •
We conducted a comprehensive systematic review and a Bayesian network meta-analysis.
- •
We evaluated the effectiveness of various interventions for orthodontic pain relief.
- •
We used recent developments in network meta-analysis for a complex network of interventions.
- •
The 3-level hierarchical model allowed ranking of interventions and intervention classes.
The prevalence of pain during fixed orthodontic treatment is high, and fear of pain is a major concern for many prospective orthodontic patients. It is well known that the placement of orthodontic separators and initial aligning archwires induces pain that reaches peak intensity at 24 hours, or 1 day, after orthodontic force application. Therefore, management of orthodontic pain at its peak intensity is of paramount clinical importance.
Recently, pairwise meta-analyses were conducted to provide answers related to the effectiveness of pharmacologic and laser interventions for orthodontic pain management after placement of separators or initial archwires. However, pairwise meta-analyses have an inherent limitation in terms of not using all the available evidence if direct comparisons are not provided by all studies included in the pairwise meta-analyses. Furthermore, many interventions such as cognitive behavioral therapy, structured phone calls, and text messages, which are often used in the management of orthodontic pain, have never been included in any previous pairwise meta-analyses.
Comparative effectiveness research relies on the accurate assessment of the treatment effectiveness of all possible interventions to provide evidence to inform health care decision makers. The network meta-analysis, also called mixed treatment comparison, has extended this concept of comparative effectiveness research by providing estimates for comparative effectiveness of all competing treatments even when no head-to-head comparisons are available. Synthesizing all available evidence (direct and indirect) also usually improves the precision of the estimates. Therefore, it is recommended that even when pairwise meta-analyses exist for any condition, the results obtained from network meta-analyses are more precise.
The statistical methods for conducting a network meta-analysis are broadly classified into 2 groups: Bayesian and frequentist. Recently, Pandis et al introduced the frequentist models of network meta-analyses in orthodontics. Both Bayesian and frequentist models are well-accepted for conducting network meta-analyses; however, Bayesian methods offer certain advantages over the frequentist method. For example, compared with the frequentist method, Bayesian methods allow greater flexibility in fitting diverse and complex networks of interventions, estimating the uncertainty in heterogeneity and the associated credible intervals based on prior distributions, and ranking each intervention included in the network as best, second best, and so on. In Bayesian methods, ranking is straightforward, based on the joint posterior distribution of all relative treatment effects. Furthermore, the most recent development of a 3-level hierarchical modeling approach in Bayesian network meta-analyses allows inclusion of sparse data in which even 1 study for any comparison can be included in the network meta-analyses without compromising the precision of the estimates. This approach allows strength to be borrowed within the classes of interventions, potentially reducing the uncertainty around the individual intervention effects, and consequently allowing the ability to rank the interventions and classes independently and inform the decision-making frameworks.
This network meta-analyses was undertaken with the objective to assess the comparative effectiveness of different interventions and intervention classes used for pain relief after orthodontic separator or initial archwire placement by combining direct and indirect evidence in an arm-based covariate-adjusted 3-level hierarchical Bayesian network meta-analysis model. The motivation to apply a 3-level hierarchical modeling is the scarcity of data because of the large number of interventions of interest and relatively few trials, which could compromise the precision of the effect estimates and the estimation of heterogeneity.
Material and methods
We followed a standard systematic review protocol according to the PRISMA guidelines and its recent adaptation for network meta-analyses. Excluding treatments from network meta-analyses can adversely affect the findings. Therefore, the eligibility criteria and search strategy were designed to ensure that studies included in this network meta-analyses would enable us to compare all possible interventions used for orthodontic pain management at the peak pain intensity level.
Eligibility criteria (PICOT)
We considered the population (patients), interventions, comparators, outcomes, and types of study (PICOT) to define the eligibility criteria for studies to be included in this network meta-analyses. Eligible studies were prospective randomized controlled trials (RCTs) evaluating the effectiveness of any pharmacologic or nonpharmacologic interventions for pain relief. The quality of evidence derived from RCTs is considered the gold standard in evaluating intervention effects. We did not specify a minimum sample size for inclusion; therefore, studies with all sample sizes were included.
To safeguard against violation of the transitivity assumption in network meta-analyses, we included studies with comparable design characteristics and plausible range of covariate distribution. The target population was defined as children and adults of both sexes with orthodontic separators or an initial archwire placed as a part of fixed orthodontic treatment. We decided to include studies with orthodontic separators or initial archwires because the pattern and magnitude of pain after their placement are similar. The index for comparative effectiveness (outcome) was the pain intensity at 24 hours, or 1 day, after separators or initial archwire placement. A control group (no treatment) was considered the reference group for comparisons of the effectiveness of interventions. Considering the diversity of interventions included in this network meta-analyses, the interventions would be classified based on the mechanism of action. Details are provided in the “Results” section.
Search strategy
The MEDLINE, Cochrane Central Register of Controlled Trials, and EMBASE databases were searched to identify the RCTs. These databases were searched until December 31, 2014, without restrictions for starting date of search or publication language.
To eliminate the possibility of excluding any intervention used for orthodontic pain relief and its impact on the network meta-analyses findings, we did not use interventions as search items ; rather, we searched for studies with “pain” or “discomfort” in the title or abstract. All such retrieved studies were then searched to determine whether an intervention for orthodontic pain relief was used.
Additional studies were identified by hand searching of 4 major orthodontic journals (1980 to December 2014): American Journal of Orthodontics and Dentofacial Orthopedics , Angle Orthodontist , European Journal of Orthodontics , and Journal of Orthodontics . Reference lists of the included studies and previously published systematic reviews and meta-analyses related to the topic were screened for identification of any additional studies.
Unpublished articles were found by electronically searching Pro-Quest Dissertations and Theses database, ClinicalTrials.gov , and National Research Register using “orthodontic” and “pain” as search terms. Conference proceedings and abstracts were also accessed when possible.
Study selection and data extraction
The titles and abstracts of all studies identified by the search strategies were independently screened by the first author (S.S.S.) to remove duplicate entries and studies that failed to meet the objectives of this network meta-analyses. Full-text articles of the remaining studies were assessed independently by 2 other authors (M.S.C., H.S.K.) for eligibility based on the predefined eligibility criteria. When disagreement occurred, the article was reread and discussed until consensus was obtained among the authors. A record of all decisions about the identified studies was kept. The review authors were not blinded to authors, institutions, or the publication sites of the studies.
Study characteristic data were extracted using a pilot-tested data extraction form: (1) study identification: first author’s name and year of publication; (2) study design; (3) population (participants): sample size, mean age, numbers of male and female participants, and proportion of female subjects; (4) interventions: details of pharmacologic or nonpharmacologic interventions including dose, frequency, mode, and timing of administration; (5) comparator assessment; and (6) outcome assessment.
Data were also extracted for potential confounders and effect modifiers that would be included in the network meta-analyses. Based on recent evidence, we identified 3 effect modifiers (age, sex, and baseline pain) that could have affected the estimates and therefore were included as a priori covariates in our network meta-analyses. Furthermore, we also decided to include another potential confounder, orthodontic procedure (separator vs initial archwire), as a covariate to adjust for the possible effect of the orthodontic procedure on the network meta-analyses estimates. Hereafter, all 4 are referred to as “covariates.”
Primary outcome assessment
The predefined primary outcome of interest was the patient-reported pain intensity at 24 hours, or 1 day, after the orthodontic procedure. For the statistical analysis, we required a mean pain score and a level of precision (standard deviation, standard error, or 95% confidence interval [CI]) estimate. If a trial did not report these summary measures, we contacted the authors for these data. When data were reported only graphically, we contacted the corresponding author for the numeric data. If this was unsuccessful, the corresponding numeric data were extracted using the Windows-based digitizing computer program UnGraph (version 5.0; Biosoft, Cambridge, United Kingdom). Data extraction with UnGraph has good reliability and validity.
We included trials that assessed pain intensity using a 100-mm visual analog scale (VAS), a 10-cm VAS scale, or a 10-point numeric rating scale. To standardize to a single scale, we assumed that the numeric rating scale (0-10) and the VAS (0-10 cm) were equivalent, and these scales were converted to the VAS (0-100 mm) by multiplying pain scores by 10. This method of combining the 100-mm VAS, 10-cm VAS, and 10-point numeric rating scale has been used in a recent meta-analysis. If a trial reported multiple effect sizes (eg, at rest, during fitting teeth together), we combined these effect sizes to obtain a single estimate, as recommended.
Assessment of the quality of the trials
Risk of bias was assessed independently by 2 authors (M.S.C., H.S.K.), and all disagreements were resolved by discussion. The Cochrane Collaboration risk of bias tool was used to assess the risk of bias based on the following domains: random sequence generation, allocation concealment, blinding of participants and personnel, blinding of assessors, incomplete outcome data, selective reporting of outcomes, and other source of bias. The other source of bias was based on the assessment of whether male and female participants were similar with respect to their mean age. This other source of bias was related to the assessment of clinical heterogeneity.
Grading the quality of evidence
The Grading of Recommendations Assessment, Development and Evaluation approach recently extended to the network meta-analysis was used to grade the quality of evidence. The quality of evidence was synthesized for the comparative effectiveness of each intervention compared with the reference group: ie, the control. Although this grading approach can be (or rather, should be) applied to all comparisons, however, depending on the objective of the network meta-analysis, it can be applied only to interventions compared with a reference group such as a control group in our network meta-analysis. The domains considered for evaluating the quality of evidence were study limitations (risk of bias), intransitivity, inconsistency or indirectness, statistical heterogeneity, imprecision (wide credible intervals), and publication bias.
Statistical analysis
A conventional pairwise meta-analysis was undertaken to compare the interventions if 2 or more studies included the same pair of interventions as a head-to-head comparison. A random-effects model (DerSimonian and Laird method) was applied using the R “meta” package (version 4.3-0). The statistical heterogeneity was assessed by the Cochran Q test and the I 2 statistic. The I 2 values of 25%, 50%, and 75% correspond to low, moderate, and high levels of heterogeneity, respectively. If more than 10 studies contributed to the same pairwise comparison, a funnel plot was drawn to assess the publication bias.
For network meta-analyses, a recently developed arm-based 3-level hierarchical Bayesian random-effects model with ordering constraints was used ; it fully accounted for the correlations between comparisons in multiarm trials. Four study-level covariates (mean age, proportion of female subjects, mean baseline pain, and orthodontic procedure) were incorporated into the network meta-analysis as part of network meta-regression. The statistical model including the assumptions used, inclusion of covariates as part of the network meta-regression, the model run-in and fit evaluation, and the prior distribution and its sensitivity analysis is described in detail in Supplementary Appendix 1 .
The network meta-analysis was implemented with the WinBUGS software (version 1.4.3) by running it from within the R software (version 3.1.2) using the R2WinBUGs package. Four individual chains with disparate starting values were analyzed, and convergence was assessed using Brooks-Gelman-Rubin statistics. The results are based on 100,000 samples, where the first 40,000 samples were discarded from the analyses as a “burn-in.” The graphic presentation for ranking the interventions and intervention classes was done using the surface under the cumulative ranking (SUCRA) values and Rankograms. SUCRA allows for ranking the interventions based on the overall percentage of surface under the curve. SUCRA would be 1 (or 100%) when an intervention is certain to be the best (ie, always ranks first) and 0 (or 0%) when an intervention is certain to be the worst. For presenting the probability that each intervention or intervention class is best, second best, third best, and so on, Rankograms were used.
Transitivity and consistency are the important assumptions related to the validity of indirect and mixed estimates in network meta-analysis. The plausibility of the transitivity assumption was evaluated based on the design characteristics and the methodology of the studies included in the network meta-analysis, as recommended. The covariates distribution was assessed at the study level as well as for the loop-specific comparisons and direct comparisons involved in the indirect and mixed estimates. The consistencies between the direct and indirect comparisons for all closed loops were evaluated using the node-splitting method. The node-splitting method is based on the calculation of 2 posterior distributions: one derived from trials that directly compared the interventions (eg, interventions X and Y) and the other indirectly derived from the remaining trials. To test for consistency between direct and indirect estimates—ie, the probability that the direct estimate surpasses the indirect estimate—a Bayesian P value was calculated.
The heterogeneity was estimated from the median of the posterior distribution of the between-trial variance, tau-square, which is a valid parameter to assess and quantify the magnitude of heterogeneity in a random-effects meta-analysis. The square root of tau-square (ie, tau) shows the standard deviation of underlying effects across the studies. In the Bayesian hierarchical random-effects models, the definition and interpretation of tau-square is similar to conventional random-effects models. Similar to a recently published network meta-analysis, we defined heterogeneity as mild if tau-square was less than 0.04, moderate if it was 0.04 to 0.14, and severe if it was greater than 0.40.
Small-study effects were assessed using the meta-regression–based approach recently introduced to Bayesian network meta-analyses. This approach is based on the assumption that biases are exchangeable across the network; ie, biases, if present, operate in a similar way in trials across the network. Therefore, we extracted the pairwise estimates from the network meta-analysis in a way that would reflect that in trials comparing active and inactive treatments (eg, placebo, control group), it could be reasonably assumed that the active treatment would be favored by a small-study bias. A similar approach was used to extract pairwise estimates to draw the comparison-adjusted funnel plot to assess small-study effects. The comparison-adjusted funnel plot was drawn using the “netfunnel” command in Stata software (version 13; StataCorp, College Station, Tex). Since interventions in our network meta-analysis were beneficial, therefore, funnel plot asymmetry with fewer studies on the left side of zero would indicate that small-study effects favor the active treatments.
Results
Search results and characteristics of studies included in the network meta-analysis
The search strategy details are provided in Supplementary Appendix 2 . A total of 236 RCTs were identified from the search strategy. After removing duplicates, 99 remained, from which we identified 24 relevant for our review. The PRISMA flow diagram is shown in Figure 1 . Five articles were excluded.
The characteristics of the studies included in the network meta-analysis are given in Supplementary Appendix 3 and summarized in Tables I and II . A total of 2273 participants (997 male, 43.86%; 1276 females, 56.14%) were included, with a mean age of 18.22 years (SD, 4.40 years), a female proportion of .54 (SD, .05), and a mean baseline VAS score of 2.56 (SD, 3.17). Fourteen RCTs used orthodontic separators as the orthodontic procedure, and the remaining 10 RCTs used initial archwires as the orthodontic procedure. The VAS was used for the assessment of pain in all studies. Two RCTs had a split-mouth design, and 1 was a crossover design. We followed the recommended procedure to extract the relevant data so that these 3 nonparallel-design RCTs could also be included in the network meta-analysis along with the rest of the parallel arm–design RCTs.
ID | Study | Study design | Intervention | Intervention class ∗ |
---|---|---|---|---|
1 | Bartlett et al, 2005 | 3 arms parallel | Control | |
Structured phone call | Behavior therapy | |||
Placebo procedural | Placebo | |||
2 | Bird et al, 2007 | 2 arms parallel | Acetaminophen preemptive | NSAID analgesic |
Ibuprofen preemptive | NSAID analgesic | |||
3 | Bradley et al, 2007 | 2 arms parallel | Ibuprofen preemptive postoperative | NSAID analgesic |
Acetaminophen preemptive postoperative | Other analgesic | |||
4 | Bruno et al, 2011 | 3 arms parallel | Control | |
Placebo pharmacological | Placebo | |||
Lumiracoxib preemptive | NSAID analgesic | |||
5 | Eslamian et al, 2013 | Split mouth | Benzocaine postoperative | Miscellaneous |
Placebo procedural | Placebo | |||
6 | Gupta et al, 2014 | 3 arms parallel | Placebo pharmacological | Placebo |
Acetaminophen preemptive postoperative | Other analgesic | |||
Etoricoxib Preemptive Postoperative | NSAID analgesic | |||
7 | Keith et al, 2013 | 2 arms parallel | Control | |
Structured text message | Behavior therapy | |||
8 | Kim et al, 2013 | 3 arms parallel | Control | |
Placebo procedural | Placebo | |||
AlGaInP semiconductor lasers | Lasers | |||
9 | Kohli and Kohli, 2011 | 3 arms parallel | Placebo pharmacological | Placebo |
Ibuprofen preemptive | NSAID analgesic | |||
Piroxicam preemptive | NSAID analgesic | |||
10 | Marini et al, 2013 | Split mouth | Control | |
Placebo procedural | Placebo | |||
GaAs superpulsed lasers | Lasers | |||
11 | Miles et al, 2012 | 2 arms parallel | Control | |
Vibrational appliance | Miscellaneous | |||
12 | Minor et al, 2009 | 3 arms parallel | Placebo pharmacological | Placebo |
Ibuprofen postoperative | NSAID analgesic | |||
Ibuprofen preemptive postoperative | NSAID analgesic | |||
13 | Ngan et al, 1994 | 3 arms parallel | Placebo pharmacological | Placebo |
Aspirin postoperative | Other analgesic | |||
Ibuprofen postoperative | NSAID analgesic | |||
14 | Nobrega et al, 2013 | 2 arms parallel | Placebo procedural | Placebo |
AlGaAs semiconductor lasers | Lasers | |||
15 | Patel et al, 2011 | Cross-over | Placebo pharmacological | Placebo |
Ibuprofen preemptive postoperative | NSAID analgesic | |||
Naproxen preemptive postoperative | NSAID analgesic | |||
Acetaminophen preemptive postoperative | Other analgesic | |||
16 | Polat et al, 2005 | 3 arms parallel | Placebo pharmacological | Placebo |
Ibuprofen preemptive | NSAID analgesic | |||
Naproxen preemptive | NSAID analgesic | |||
17 | Polat and Karaman, 2005 | 6 arms parallel | Placebo pharmacological | Placebo |
Ibuprofen preemptive postoperative | NSAID analgesic | |||
Flurbiprofen preemptive postoperative | NSAID analgesic | |||
Acetaminophen preemptive postoperative | Other analgesic | |||
Naproxen preemptive postoperative | NSAID analgesic | |||
Aspirin preemptive postoperative | Other analgesic | |||
18 | Roth and Thrash, 1986 | 3 arms parallel | Control | |
Placebo procedural | Placebo | |||
Transcutaneous electrical nerve stimulation | Miscellaneous | |||
19 | Salmassian et al, 2009 | 3 arms parallel | Placebo pharmacological | Placebo |
Ibuprofen postoperative | NSAID analgesic | |||
Acetaminophen postoperative | Other analgesic | |||
20 | Steen Law et al, 2000 | 3 arms parallel | Placebo pharmacological | Placebo |
Ibuprofen postoperative | NSAID analgesic | |||
Ibuprofen preemptive | NSAID analgesic | |||
21 | Sudhakar et al, 2014 | 4 arms parallel | Placebo pharmacological | Placebo |
Aspirin preemptive postoperative | Other analgesic | |||
Ibuprofen preemptive postoperative | NSAID analgesic | |||
Acetaminophen preemptive postoperative | Other analgesic | |||
22 | Tortamano et al, 2009 | 3 arms parallel | Control | |
Placebo procedural | Placebo | |||
AlGaAs semiconductor lasers | Lasers | |||
23 | Turhani et al, 2006 | 2 arms parallel | Placebo procedural | Placebo |
AlGaAs semiconductor lasers | Lasers | |||
24 | Wang et al, 2012 | 3 arms parallel | Control | |
Ibuprofen postoperative | NSAID analgesic | |||
Cognitive behavioral therapy | Behavior therapy |
∗ Refer to the text for details of the Interventions classification.
ID | Study | Sample (n) | Male (n) | Female (n) | Female proportion | Female proportion, SE ∗ | Mean age (y) | Mean age SD (y) | Mean baseline pain | Mean baseline pain, SD | Orthodontic procedure † |
---|---|---|---|---|---|---|---|---|---|---|---|
1 | Bartlett et al, 2005 | 150 | 69 | 81 | 0.54 | 0.04 | 15.97 | 6.73 | 0.4 | 1.83 | Archwire |
2 | Bird et al, 2007 | 33 | 16 | 17 | 0.52 | 0.09 | 14.05 | 2.75 | 2.75 | 6.2 | Separator |
3 | Bradley et al, 2007 | 159 | 56 | 103 | 0.65 | 0.04 | 13.75 | 1.1 | 0 | 0 | Separator |
4 | Bruno et al, 2011 | 51 | 13 | 38 | 0.75 | 0.06 | 23.25 | 4.23 | 0 | 0 | Separator |
5 | Eslamian et al, 2013 | 60 | 36 | 24 | 0.4 | 0.06 | 23 | 3.75 | 5.63 | 6.37 | Separator |
6 | Gupta et al, 2014 | 45 | 22 | 23 | 0.51 | 0.07 | 18.27 | 2.07 | 0 | 0 | Archwire |
7 | Keith et al, 2013 | 39 | 14 | 25 | 0.64 | 0.08 | 13.4 | 2.15 | 5.58 | 10.12 | Archwire |
8 | Kim et al, 2013 | 88 | 23 | 65 | 0.74 | 0.05 | 22.7 | 6.14 | 7.28 | 4.22 | Separator |
9 | Kohli and Kohli, 2011 | 90 | 45 | 45 | 0.5 | 0.05 | 14.67 | 7.07 | 0 | 0 | Separator |
10 | Marini et al, 2013 | 120 | 64 | 56 | 0.47 | 0.05 | 23.01 | 1.39 | 7.01 | 8.73 | Separator |
11 | Miles et al, 2012 | 66 | 26 | 40 | 0.61 | 0.06 | 13.05 | 0.96 | 6.19 | 8.53 | Archwire |
12 | Minor et al, 2009 | 51 | 21 | 30 | 0.59 | 0.07 | 16.3 | 3.77 | 10.57 | 8.79 | Separator |
13 | Ngan et al, 1994 | 77 | 43 | 34 | 0.44 | 0.06 | 16.53 | 6.57 | 10.55 | 7.99 | Separator |
14 | Nobrega et al, 2013 | 60 | 22 | 38 | 0.63 | 0.06 | 17.5 | 3.9 | 0 | 0 | Separator |
15 | Patel et al, 2011 | 96 | 52 | 44 | 0.46 | 0.05 | 26.4 | 2.5 | 0 | 0 | Separator |
16 | Polat et al, 2005 | 60 | 37 | 23 | 0.38 | 0.06 | 16 | 5.1 | 0 | 0 | Archwire |
17 | Polat and Karaman, 2005 | 120 | 76 | 44 | 0.37 | 0.04 | 15.33 | 4.1 | 0 | 0 | Archwire |
18 | Rothand Thrash, 1986 | 45 | 27 | 18 | 0.4 | 0.07 | 28 | 3.7 | 0 | 0 | Separator |
19 | Salmassian et al, 2009 | 60 | 31 | 29 | 0.48 | 0.06 | 14.75 | 1.6 | 2.4 | 5.8 | Archwire |
20 | Steen Law et al, 2000 | 63 | 25 | 38 | 0.6 | 0.06 | 13.27 | 1.63 | 0 | 0 | Separator |
21 | Sudhakar et al, 58 2014 | 154 | 77 | 77 | 0.5 | 0.04 | 19 | 1.63 | 0 | 0 | Separator |
22 | Tortamano et al, 2009 | 60 | 18 | 42 | 0.7 | 0.06 | 15.88 | 1.4 | 0 | 0 | Archwire |
23 | Turhani et al, 2006 | 76 | 30 | 46 | 0.61 | 0.06 | 23.05 | 3.35 | 0 | 0 | Archwire |
24 | Wang et al, 2012 | 450 | 154 | 296 | 0.66 | 0.02 | 16.84 | 5.45 | 3.28 | 7.54 | Archwire |
The evidence network plot is shown in Figure 2 . In the 24 RCTs, we identified a total of 26 different interventions, which were further classified into 6 classes based on their mechanism of action: (1) placebo, (2) nonsteroidal anti-inflammatory drugs (NSAID) analgesics, (3) “other” analgesics, (4) lasers, (5) behavior therapy, and (6) miscellaneous. The details of the interventions and intervention classes are provided in Table I . The rationale and justification for the intervention classifications are given in Section C of Supplementary Appendix 1 .
Risk of bias assessment
The risk of bias assessed for each study is shown in Figure 3 . For 2 studies, the risk of bias was high for the randomization process. There was no evidence for a high risk of bias in any other domain, although a few studies did not provide relevant information; thus, we assigned an unclear risk of bias. Because many studies used nonpharmacologic interventions and it could have been impossible for the investigators to design double-blind trials, a judgment based on the consensus among the authors was made to assess the risk of bias for 2 domains related to the blinding process.
Pairwise meta-analysis findings
Results of random-effects pairwise meta-analysis for 10 available head-to-head pairwise comparisons (when 2 or more studies used the same comparison) is presented in Supplementary Appendix 4 . The heterogeneity (I 2 ) varied from 0% to 69%, and all effect sizes were associated with wide 95% CIs. Since there were few studies for each comparison (maximum of 5 studies per comparisons), we could not use conventional funnel plots to assess the small-study effect.
Network meta-analysis findings
Table III shows the summary of the network meta-analysis results. The covariate-adjusted model had a substantially better fit than the nonadjusted model as shown by the deviance information criterion value, which was smaller by approximately 68 units. The distribution of the study-level covariates included in the network meta-analysis is shown in Table II . The models incorporating ordering constraints had a better fit than the nonconstrained models; thus, the results are based on estimates derived from the constrained network meta-analysis. The summary of network meta-analysis estimates with and without the ordering constraints is given in Supplementary Appendix 5 .
Intervention | Covariate adjusted NMA estimates ∗ | Nonadjusted NMA estimates | ||
---|---|---|---|---|
Median rank (95% CrI) | SUCRA % (95% CrI) | Median rank (95% CrI) | SUCRA % (95% CrI) | |
Control | 26 (26-26) | 0 (0-0) | 26 (26-26) | 0 (0-0) |
Placebo pharmacological | 23 (19-25) | 11.55 (4-28) | 22 (18-24) | 15.61 (6-33) |
Placebo procedural | 24 (21-25) | 8.27 (4-20) | 22 (19-24) | 12.24 (5-31) |
Ibuprofen preemptive | 15 (7-22) | 44.75 (16-76) | 15 (7-22) | 44.67 (16-76) |
Ibuprofen postoperative | 12 (6-17) | 56.79 (36-80) | 12 (6-16) | 56.83 (36-80) |
Ibuprofen preemptive postoperative | 8 (3-15) | 69.66 (44-92) | 9 (4-16) | 64.83 (41-89) |
Acetaminophen preemptive | 13 (3-22) | 52.78 (16-92) | 12 (4-23) | 58.78 (16-92) |
Acetaminophen postoperative | 14 (4-22) | 50.44 (16-88) | 13 (5-23) | 50.4 (16-88) |
Acetaminophen preemptive postoperative | 11 (5-18) | 59.21 (32-84) | 11 (5-17) | 60.25 (34-87) |
Aspirin postoperative | 17 (9-23) | 36.77 (12-68) | 17 (9-23) | 36.73 (11-66) |
Aspirin preemptive postoperative | 6 (2-14) | 78.56 (48-96) | 6 (2-14) | 78.79 (48-96) |
Naproxen preemptive | 6 (2-17) | 74.91 (36-96) | 6 (2-17) | 74.82 (36-94) |
Naproxen preemptive postoperative | 6 (2-14) | 78.11 (48-96) | 6 (2-15) | 75.09 (45-93) |
Lumiracoxib preemptive | 8 (2-18) | 70.11 (32-96) | 8 (2-18) | 69.89 (31-94) |
Etoricoxib preemptive postoperative | 1 (1-3) | 98.91 (92-100) | 1 (1-4) | 99.01 (92-98) |
Piroxicam preemptive | 3 (1-12) | 86.38 (56-100) | 4 (1-12) | 86.32 (56-98) |
Flurbiprofen preemptive postoperative | 14 (5-22) | 48.77 (16-84) | 14 (5-23) | 49.06 (15-81) |
Benzocaine postoperative | 20 (11-25) | 25.61 (4-60) | 20 (11-25) | 25.57 (4-60) |
Structured text message | 21 (11-25) | 23.65 (4-60) | 22 (12-25) | 21.43 (4-51) |
Structured phone call | 20 (12-25) | 24.08 (4-56) | 19 (11-24) | 33.99 (14-69) |
Cognitive behavioral therapy | 16 (6-21) | 41.91 (20-80) | 16 (7-23) | 41.79 (16-80) |
Transcutaneous electrical nerve stimulation | 20 (12-24) | 24.17 (8-56) | 20 (13-25) | 23.12 (5-52) |
Vibrational appliance | 22 (14-25) | 16.67 (4-48) | 21 (13-23) | 18.9 (4-48) |
AlGaInP semiconductor lasers | 7 (2-17) | 71.64 (36-96) | 7 (2-16) | 71.71 (36-96) |
AlGaAs semiconductor lasers | 11 (3-19) | 59.7 (28-92) | 11 (3-20) | 59.62 (28-92) |
GaAs superpulsed lasers | 3 (1-13) | 86.51 (52-100) | 3 (1-14) | 86.46 (52-100) |
Intervention class | ||||
Placebo | 6 (4-6) | 6.27 (0-40) | 6 (4-6) | 6.18 (0-40) |
Analgesics (NSAIDs) | 2 (1-3) | 87.08 (60-100) | 2 (1-4) | 86.89 (60-100) |
Analgesics (other) | 3 (1-4) | 64.24 (40-100) | 3 (1-4) | 63.84 (40-100) |
Lasers | 2 (1-3) | 84.77 (60-100) | 2 (1-3) | 85.13 (60-100) |
Behavior therapy | 4 (3-6) | 33.96 (0-60) | 4 (3-6) | 34.27 (0-60) |
Miscellaneous | 5 (3-6) | 23.68 (0-60) | 5 (3-6) | 23.7 (0-60) |
Covariate estimates | Estimate (95% CrI) | Estimate (95% CrI) |
---|---|---|
Age (y; mean centered) | −0.147 (−2.616 to 2.087) | NA |
Sex (% females; mean centered) | −0.831 (−0.958 to −0.704) | NA |
Orthodontic procedure (binary: 0, 1) | −4.141 (−27.7 to 17) | NA |
Baseline pain (VAS score; mean centered) | −0.715 (−0.879 to −0.551) | NA |
Between study heterogeneity and fit statistics | Estimate (95% CrI) | Estimate (95% CrI) |
---|---|---|
Tau-square | 0.044 (0.040-0.055) | 0.099 (0.092-0.107) |
Deviance information criterion | 225.132 (203.813-248.623) | 293.639 (259.219-321.619) |
∗ Four study-level covariates (3 continuous, 1 binary) were included in the NMA as part of the network meta-regression analysis. The continuous covariates were mean age, proportion of female subjects, and mean baseline pain. Each continuous covariate was included as a mean centered standardized covariate (mean, 0; SD, 1). The binary covariate, orthodontic procedure, was coded as 1, archwire; 0, separator. Refer to Table II for a summary of each study-level covariate.
The pairwise summary forest plot for each intervention is shown in Supplementary Appendix 6 . Interestingly, the direct estimates derived from the network meta-analysis were more precise than the corresponding direct estimates from the pairwise meta-analyses. This was particularly true when heterogeneity was large in the pairwise meta-analyses. For example, the placebo pharmacologic vs naproxen preemptive postoperative comparison with moderate to high heterogeneity (I 2 , 69.1%) had a wide 95% CI for the pairwise meta-analysis (estimate, −19.62; 95% CI, −38.05 to −1.20) compared with the narrow 95% CrI in the network meta-analysis (estimate, −17.96; 95% CrI, −28.53 to −8.12). This probably is because the estimates from the network meta-analysis were adjusted for the covariates.
Interestingly, the direct estimates in the network meta-analysis were more precise than the indirect and mixed estimates. A reason for this paradox could be the common heterogeneity assumption in the model used for the network meta-analysis. Under the assumption of common heterogeneity, comparisons with little or no heterogeneity share the same heterogeneity with comparisons with large heterogeneity; as a result, the network meta-analysis estimates appear to be less precise than the direct estimates.
Table III summarizes the median rank and SUCRA values for each intervention and intervention class. Figure 4 summarizes the pairwise estimates of interventions compared with the reference (control) group. The etoricoxib was the most effective intervention compared with the control (estimate, −41.96; 95% CrI, −52.85 to −30.69) with a median rank of 1, followed by the gallium-arsenide superpulsed laser (estimate, −34.01; 95% CrI, −42.57 to −25.66) and preemptive piroxicam (estimate, −33.72; 95% CrI, −45.17 to −21.82) with a shared median rank of 3. Figure 5 shows the SUCRA plot for all interventions. The Rankograms for each intervention are shown in Supplementary Appendix 7 .
Compared with a placebo class, NSAID analgesics (estimate, −19.67; 95% CrI, −28.81 to −10.71) and lasers (estimate, −19.66; 95% CrI, −30.15 to −9.15) were the most effective intervention classes with a shared median rank of 2, followed by the other analgesics class (estimate, −15.26; 95% CrI, −25.44 to −5.41) with a median rank of 3. The behavior therapy and miscellaneous intervention classes were not significantly effective compared with the placebo class ( Fig 6 ). Figure 7 shows the SUCRA plot for all intervention classes. The Rankograms for each intervention class are shown in Supplementary Appendix 8 .
Sensitivity analysis for prior distribution
Sensitivity to the prior distribution for the between-study variances and the intervention class variances showed little evidence of an impact on the overall effect estimates and precision, as shown in Supplementary Appendix 9 . This suggests that all sets of analyses are insensitive to the choice of prior distributions and that network meta-analysis estimates are not affected by prior distribution.
Transitivity and inconsistency assessment
The assessment showed no major threat to the transitivity assumption from study design characteristics. We adjusted the network meta-analysis estimates by including covariates as part of network meta-regression; this further improved the plausibility of the transitivity assumption. The covariate distributions for loop-specific comparisons and direct pairwise comparisons are presented in Supplementary Appendix 10 .
Results from the node-split method to estimate inconsistency showed no substantial evidence for inconsistency ( Table IV ). Out of a total 41 comparisons that provided both direct and indirect estimates and eventually were included in the node-split method inconsistency estimation, 3 comparisons—acetaminophen (preemptive and postoperative) vs etoricoxib (preemptive and postoperative), placebo (pharmacologic) vs aspirin (preemptive and postoperative), and placebo (pharmacologic) vs etoricoxib (preemptive and postoperative), constituting 7.3% of the total evidence (3/41 = 0.0731)—showed significant inconsistencies. The results showed that covariate adjustments improved the consistency of the network meta-analysis. For example, the number of comparisons with a significant inconsistency (Bayesian P value <0.05) was 8 (19.5%) for unadjusted network meta-analysis, whereas only 3 comparisons (7.3%) were inconsistent when covariates were included in the network meta-analysis. Furthermore, even for the 3 comparisons with inconsistency in the covariate-adjusted network meta-analysis, the inconsistency estimate was less than the nonadjusted network meta-analysis. The results for the node-split method to estimate the inconsistency without covariate adjustment are shown in Supplementary Appendix 11 .
Intervention comparison | NMA estimates | Direct estimates | Indirect estimates | IF (difference direct −indirect) | P | ||||||||
---|---|---|---|---|---|---|---|---|---|---|---|---|---|
Mean | 95% CrI | Mean | 95% CrI | Mean | 95% CrI | Mean | 95% CrI | ||||||
Lower | Upper | Lower | Upper | Lower | Upper | Lower | Upper | ||||||
Control vs placebo pharmacological | −10.48 | −18.80 | −2.12 | −6.60 | −28.31 | 15.11 | −7.92 | −17.83 | 2.09 | 1.32 | −26.62 | 29.45 | 1.000 |
Control vs placebo procedural | −8.97 | −13.97 | −3.88 | −8.40 | −13.24 | −3.61 | −8.54 | −20.28 | 3.06 | 0.14 | −14.59 | 14.43 | 1.000 |
Control vs ibuprofen postoperative | −25.37 | −33.14 | −17.43 | −32.22 | −37.09 | −27.35 | −21.47 | −34.77 | −7.36 | −10.75 | −33.23 | 13.08 | 0.400 |
Control vs lumiracoxib preemptive | −28.70 | −40.27 | −17.24 | −23.30 | −45.01 | −1.59 | −24.96 | −38.31 | −11.94 | 1.66 | −26.82 | 30.90 | 1.000 |
Control vs structured text message | −15.10 | −25.31 | −4.86 | −8.07 | −21.89 | 5.75 | −18.36 | −33.04 | −3.63 | 10.29 | −16.67 | 38.09 | 1.000 |
Control vs structured phone call | −15.45 | −24.15 | −6.85 | −11.17 | −18.95 | −3.39 | −17.71 | −33.62 | −1.78 | 6.54 | −19.20 | 31.87 | 1.000 |
Control vs cognitive behavioral therapy | −21.27 | −29.43 | −13.02 | −28.85 | −33.69 | −24.02 | −10.39 | −26.32 | 5.81 | −18.46 | −42.27 | 6.72 | 0.156 |
Control vs transcutaneous electrical nerve stimulation | −15.33 | −23.78 | −7.20 | −11.11 | −16.24 | −5.98 | −11.54 | −28.24 | 5.37 | 0.43 | −25.84 | 25.30 | 1.000 |
Control vs vibrational appliance | −12.22 | −22.44 | −2.06 | −6.10 | −18.59 | 6.39 | −16.76 | −32.55 | −0.93 | 10.66 | −16.21 | 37.76 | 1.000 |
Control vs AlGaInP semiconductor lasers | −29.09 | −36.90 | −21.42 | −28.83 | −31.90 | −25.76 | −29.42 | −43.31 | −15.67 | 0.59 | −22.45 | 23.53 | 1.000 |
Control vs AlGaAs semiconductor lasers | −25.95 | −33.39 | −18.37 | −31.00 | −42.50 | −19.50 | −28.67 | −40.23 | −16.64 | −2.33 | −24.51 | 23.89 | 0.980 |
Control vs GaAs superpulsed lasers | −34.02 | −42.57 | −25.66 | −47.30 | −56.77 | −37.83 | −25.32 | −38.93 | −11.66 | −21.98 | −43.05 | 5.21 | 0.120 |
Placebo pharmacological vs ibuprofen preemptive | −12.15 | −19.66 | −4.62 | −2.23 | −12.37 | 7.86 | −18.52 | −27.93 | −9.38 | 16.29 | −1.20 | 30.85 | 1.000 |
Placebo pharmacological vs ibuprofen postoperative | −14.89 | −21.12 | −8.74 | −4.72 | −11.76 | 2.41 | −18.58 | −28.30 | −9.24 | 13.86 | −2.56 | 26.90 | 1.000 |
Placebo pharmacological vs ibuprofen preemptive postoperative | −17.84 | −24.17 | −11.65 | −16.19 | −24.48 | −8.08 | −16.80 | −26.37 | −7.25 | 0.61 | −13.53 | 15.39 | 1.000 |
Placebo pharmacological vs acetaminophen postoperative | −13.30 | −23.04 | −3.48 | −4.80 | −21.70 | 12.10 | −15.45 | −26.73 | −3.99 | 10.65 | −17.73 | 35.03 | 1.000 |
Placebo pharmacological vs acetaminophen preemptive postoperative | −15.60 | −21.48 | −9.67 | −20.71 | −27.62 | −13.68 | −16.27 | −27.25 | −4.98 | −4.44 | −16.40 | 12.34 | 0.800 |
Placebo pharmacological vs aspirin postoperative | −9.83 | −17.82 | −1.57 | −0.10 | −7.04 | 6.84 | −17.11 | −28.88 | −5.64 | 17.01 | −6.26 | 39.09 | 1.000 |
Placebo pharmacological vs aspirin preemptive postoperative | −20.23 | −28.08 | −12.41 | −33.27 | −44.24 | −21.96 | −11.38 | −22.63 | 0.08 | −21.89 | −40.20 | −3.75 | 0.020 |
Placebo pharmacological vs naproxen preemptive | −19.55 | −29.15 | −9.85 | −19.10 | −38.51 | 0.31 | −16.70 | −26.79 | −7.00 | −2.40 | −31.68 | 23.32 | 0.768 |
Placebo pharmacological vs naproxen preemptive postoperative | −20.01 | −27.32 | −12.58 | −17.96 | −28.53 | −8.12 | −16.02 | −26.37 | −6.01 | −1.94 | −25.42 | 9.03 | 0.360 |
Placebo pharmacological vs lumiracoxib preemptive | −18.22 | −27.73 | −8.66 | −16.70 | −38.41 | 5.01 | −17.02 | −27.11 | −7.21 | 0.32 | −27.36 | 30.16 | 1.000 |
Placebo pharmacological vs etoricoxib preemptive postoperative | −31.43 | −39.49 | −23.56 | −54.00 | −60.34 | −47.66 | −13.80 | −22.87 | −4.89 | −40.20 | −59.18 | −17.62 | 0.000 |
Placebo pharmacological vs piroxicam preemptive | −23.17 | −32.20 | −14.02 | −33.65 | −48.82 | −18.48 | −15.78 | −25.60 | −6.27 | −17.87 | −43.42 | 6.28 | 0.140 |
Placebo pharmacological vs flurbiprofen preemptive postoperative | −13.00 | −22.45 | −3.29 | −13.48 | −33.96 | 7.01 | −18.02 | −27.97 | −8.50 | 4.54 | −9.98 | 44.38 | 1.000 |
Placebo procedural vs transcutaneous electrical nerve stimulation | −6.37 | −14.81 | 1.79 | −15.83 | −23.92 | −7.75 | −3.38 | −20.62 | 14.02 | −12.45 | −36.54 | 14.94 | 0.432 |
Placebo procedural vs AlGaInP semiconductor lasers | −20.12 | −27.70 | −12.68 | −19.57 | −22.75 | −16.39 | −21.14 | −34.43 | −8.20 | 1.57 | −20.71 | 24.57 | 1.000 |
Placebo procedural vs AlGaAs semiconductor lasers | −16.99 | −23.65 | −10.29 | −11.55 | −19.18 | −4.73 | −24.61 | −39.46 | −9.70 | 13.06 | −10.09 | 28.25 | 1.000 |
Placebo procedural vs GaAs superpulsed lasers | −25.06 | −33.22 | −17.01 | −25.60 | −33.29 | −17.91 | −17.47 | −30.29 | −4.67 | −8.13 | −32.62 | 14.09 | 0.438 |
Ibuprofen preemptive vs naproxen preemptive | −7.40 | −17.64 | 2.72 | −14.80 | −35.42 | 5.83 | −2.69 | −13.60 | 5.92 | −12.11 | −35.80 | 18.73 | 0.520 |
Ibuprofen preemptive vs piroxicam preemptive | −11.01 | −20.83 | −1.11 | −27.08 | −42.90 | −11.25 | −1.86 | −12.16 | 6.57 | −25.22 | −47.95 | 1.58 | 0.060 |
Ibuprofen postoperative vs ibuprofen preemptive postoperative | −2.95 | −10.50 | 4.57 | 9.00 | −20.40 | 38.40 | −1.62 | −10.25 | 6.01 | 10.62 | −19.40 | 46.32 | 1.000 |
Ibuprofen postoperative vs acetaminophen postoperative | 1.59 | −8.89 | 12.18 | −0.40 | −18.39 | 17.59 | 0.03 | −12.12 | 11.81 | −0.43 | −24.41 | 29.69 | 1.000 |
Ibuprofen postoperative vs cognitive behavioral therapy | 4.11 | −5.13 | 13.36 | 3.37 | −1.41 | 8.14 | 14.58 | −4.37 | 33.36 | −11.21 | −37.99 | 14.99 | 0.400 |
Ibuprofen preemptive postoperative vs acetaminophen preemptive postoperative | 2.24 | −3.53 | 8.04 | 2.62 | −2.94 | 8.18 | 1.74 | −9.55 | 13.12 | 0.88 | −13.59 | 15.00 | 1.000 |
Ibuprofen preemptive postoperative vs aspirin preemptive postoperative | −2.38 | −10.06 | 5.29 | −13.85 | −30.82 | 3.12 | 5.76 | −5.30 | 17.47 | −19.61 | −31.73 | 3.42 | 0.120 |
Ibuprofen preemptive postoperative vs naproxen preemptive postoperative | −2.17 | −9.62 | 5.27 | −2.57 | −11.99 | 6.67 | 0.19 | −9.28 | 9.87 | −2.76 | −24.21 | 8.77 | 0.360 |
Ibuprofen preemptive postoperative vs flurbiprofen preemptive postoperative | 4.84 | −4.70 | 14.46 | 22.82 | 2.36 | 43.29 | −1.08 | −10.61 | 7.33 | 23.90 | 0.10 | 52.38 | 1.000 |
Acetaminophen preemptive postoperative vs aspirin preemptive postoperative | −4.63 | −12.20 | 2.85 | −9.37 | −26.00 | 7.25 | 1.34 | −7.30 | 11.45 | −10.71 | −28.87 | 4.77 | 0.162 |
Acetaminophen preemptive postoperative vs naproxen preemptive postoperative | −4.41 | −11.79 | 2.86 | −11.25 | −27.66 | 5.16 | −2.47 | −13.44 | 8.21 | −8.78 | −20.44 | 12.46 | 0.640 |
Acetaminophen preemptive postoperative vs etoricoxib preemptive postoperative | −15.83 | −23.73 | −7.83 | −22.00 | −27.06 | −16.94 | 1.64 | −7.75 | 11.47 | −23.64 | −44.77 | −3.79 | 0.022 |
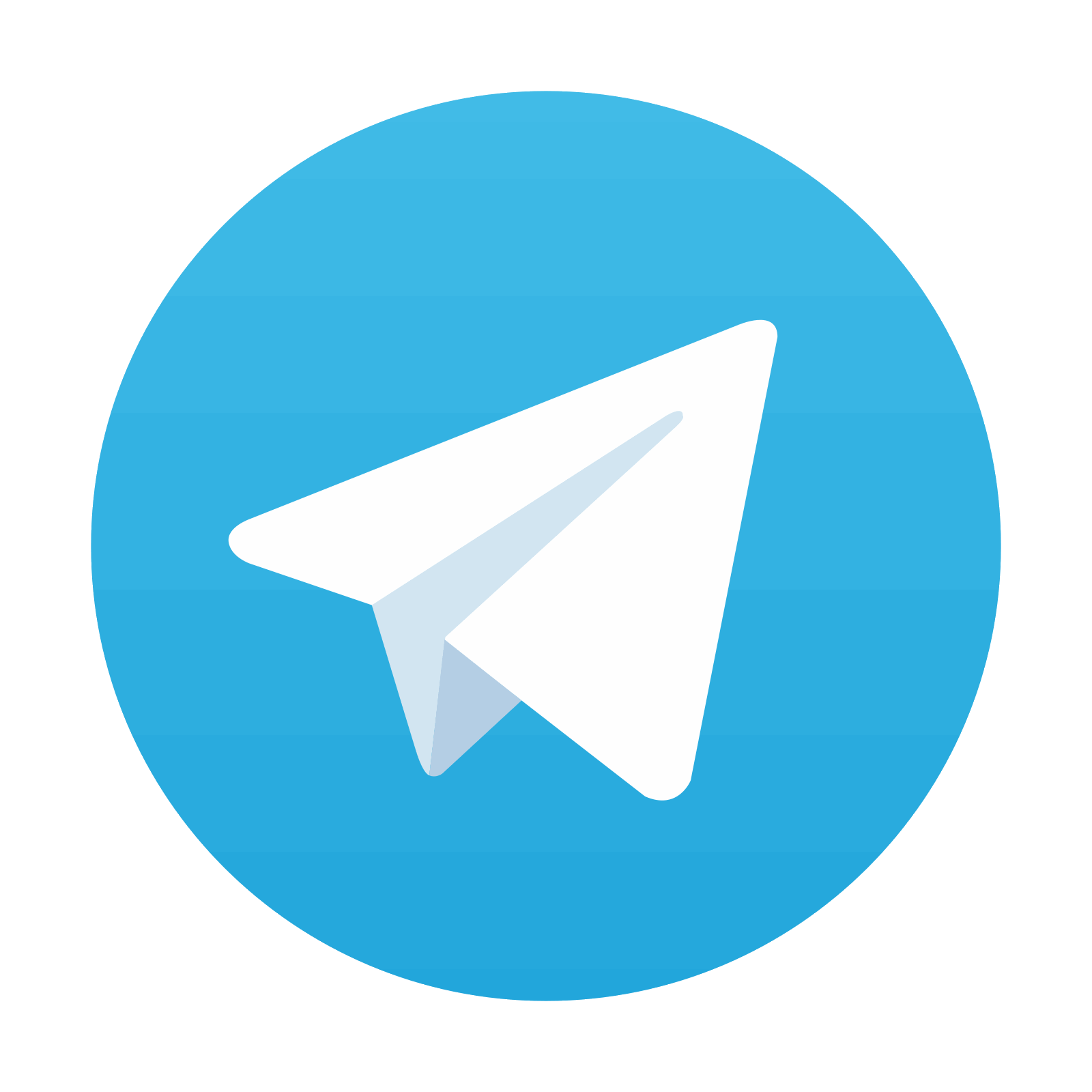
Stay updated, free dental videos. Join our Telegram channel

VIDEdental - Online dental courses
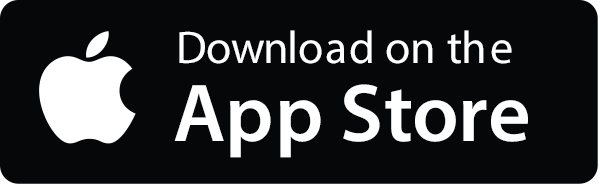

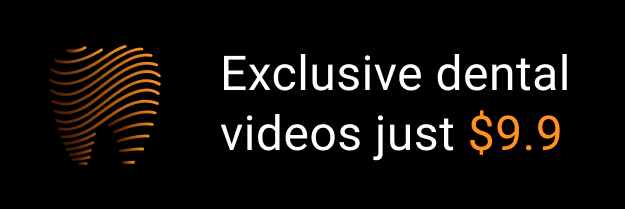