(1)
Department of Neurology Neurosciences Centre, and Clinical Epidemiology Unit, All India Institute of Medical Sciences, New Delhi Delhi, India
Abstract
Health practitioners are rightly interested to know whether a new treatment works, whether it makes any difference, difference in what, in the outcome of his case or the prognosis of his patient. But there is a problem. The problem is that there are many factors which influence the outcome in a given patient. Some such factors are called prognostic factors, like age, sex, nature of the disease, disease severity, and co-morbidities. The other factors are biases and chance. If we can eliminate these factors and other treatments as the possible cause of a particular outcome, then we can be sure that the new treatment given to the patient has caused the outcome – whether beneficial or adverse. But it is impossible to eliminate the role of these factors. Hence it is difficult, if not impossible, to know for sure whether a given treatment makes a difference in the outcome. Researchers use several strategies to control these extraneous factors; one of which is to use a control group.
Introduction
Health practitioners are rightly interested to know whether a new treatment works, whether it makes any difference. A question may arise: difference in what? The answer is: in the outcome of his case or the prognosis of his patient. But there is a problem. The problem is that there are many factors which influence the outcome in a given patient. Some such factors are called prognostic factors, like age, sex, nature of the disease, disease severity, and co-morbidities. The other factors are biases and chance. If we can eliminate these factors and other treatments as the possible cause of a particular outcome, then we can be sure that the new treatment given to the patient has caused the outcome – whether beneficial or adverse. But it is impossible to eliminate the role of these factors. Hence it is difficult, if not impossible, to know for sure whether a given treatment makes a difference in the outcome. Researchers use several strategies to control these extraneous factors; one of which is to use a control group.
Need for a Control Group
Give the treatment to a group of patients and observe the outcome. This strategy is the one most commonly used. But it has its own problems. First, the disease may be self-remitting in some or all patients. Thus, whether the patients recovered due to the treatment or on their own, you cannot say. Second, there is something called Hawthorne effect – that is, there is a change in response or behaviours of people when they are kept under observation. Third, there is placebo effect – that is, patients feel improvement even if something inactive (placebo) is given. If there is a control group who receives the same attention as the experimental one and also receive a placebo, then the Hawthorne and placebo effects cancel out in the comparison between the experimental and control group. For example, studies in early eighties with a single group of patients reported moderate to marked improvement with thyrotrophin releasing hormone in motor neurone disease, but subsequent controlled trials did not find any significant improvement. Fourth, there is something called ‘regression to the mean’. To understand this, let us consider a group of healthy subjects with a true mean systolic blood pressure (SBP) of 135 mmHg. They were attending your outpatient department (OPD). As you know, there is physiological fluctuation in SBP and it rises particularly when the patients are being examined by a health-care worker (so called white-coat hypertension). Suppose, if you did not know this and you wanted to test the effects of a new anti-hypertensive drug. You picked up some of the above patients with SBP above 140 mmHg in the OPD. You administered the drug to all such patients. After 1 h you checked their SBP again. Prior to treatment, mean of their SBP was 142 mm, but it came down to 137 after 1 h, and the difference turned out to be statistically significant. Does it mean the drug is effective? The answer is we don’t know. The SBP may have come down in normal course without treatment. The reason is that you picked up those with the upswing in their SBP. Such upswings are known to be normal. What happens after the upswings – SBP will come down towards its mean. This phenomenon is called ‘regression to the mean’. This happens spontaneously. So, you won’t know whether the drug did something or SBP came down because of ‘regression to the mean’ phenomenon. Patients selected because of high value of any characteristic can be expected to have lower value on subsequent measurements, purely because of phenomenon of ‘regression to the mean’.
Randomisation
Randomisation is a method to allocate individual patients or persons who have been accepted for a study into one of the groups (called arms) of a study. Usually, there are two arms: One arm is called experimental or treatment arm and the other is called control arm. Another term for randomisation is random allocation. This should not be confused with random selection, in which the investigator uses a process to recruit sample for the study. This is used to select a representative sample from the study population, usually in a survey.
Randomisation process is like tossing a coin to allocate the patients into the different arms of the study. Let us say there are two arms in a study. Mr. X is a patient who is eligible and has given consent. Now a decision has to be made to put him into either experimental or control arm of the study. You first set a rule that each time a patient is accepted into the study, you will toss a coin – if it comes head, he will go to (say) experimental arm; however, if it comes tail, he will go to control arm. Accordingly, Mr. X comes, you toss a coin – it comes tail; therefore, Mr. X goes to control arm. Likewise, whenever a patient comes, the same steps and rules are followed. Finally, you will have two groups totally created by tossing a coin. As you can imagine, if there are two hundred subjects, approximately 100 will be in experimental arm and another approximately 100 in control arm. Thus, you will have two groups created through randomisation.
The question is why do we randomise? We randomise to create two prognostically similar groups. In the 200 subjects we had, if there were 50 females, you will find roughly (not exactly) half in each group; if there were forty old people, you will find roughly half in each group; if you had 80 diabetics, you will find roughly half of them in each group. It happens because each patient has equal (50–50) probability of going into one of the two arms. This divides all the characteristics, measured or non-measured, visible or invisible and known or unknown, approximately equally into the two arms, provided you have enough number (say hundreds) of patients. When is the number enough? The exact number depends on how many factors you want to balance. Of course, it will be roughly half and half, not exactly. In fact, if you are very unlucky and have small numbers, you might find one-third in one group and two-thirds in another group. This is why you need to check whether the randomisation worked well in your study or not.
There are two more advantages of randomisation – one, you cannot consciously or subconsciously introduce bias in selection of the patients. If you were relying on alternate patients going to one or the other arm – say, medical versus surgical – you may select only the low-risk patients into surgical and the high-risk cases into medical. This is possible if you are deciding the eligibility and taking consent from the patients and you also know that next patient goes to one particular arm (more details on this in Chap. 4). Second advantage of randomisation is that it meets the assumption of all the statistical tests used to compare the two groups.
Intention-to-Treat Analysis
In our hospital in India, serious patients with spontaneous supratentorial intracerebral haemorrhage (SSIH) were all admitted in neurology and treated medically with hyperventilation, mannitol, control of hypertension, etc. Our policy was to treat such patients medically. Our chief of neurosciences, a neurosurgeon, returned after visiting some neurocentres in the UK and USA and convened a meeting of neurologists. The discussion was on the following lines:
Neurosurgeon (NS):
I found in all the centres I visited that their policy is to admit all serious patients with SSIH to neurosurgery, particularly those with altered consciousness. Right from the emergency, neurosurgery is involved. Most, but not all, patients are operated upon. My impression is that this approach yields better outcome.
Neurologist (N):
I do not believe that surgery has a role in SSIH. I have looked at the available evidence from randomised studies. It does not favour a policy to treat such patients in neurosurgery.
NS:
OK, I understand your point. See, your intention is to continue the present policy – that is, all patients are admitted and cared for by neurology service – some of them may require surgery at a later date. My intention is to treat all patients with altered consciousness in neurosurgery so that such patients get early surgery. Let us compare the two policies in a randomised study, particularly for patients with altered consciousness: intention to treat medically with later surgery if required versus intention to treat with surgery early.
N:
What will you do with the conclusions?
NS:
If the policy of intention to do early surgery is associated with decreased mortality, we will adopt this policy so that right from emergency, patients will go to neurosurgery. Otherwise, the present policy will continue. My impression is that early surgery policy will reduce mortality rate of such patients in our hospital and this will have national or even international impact as regards treatment of such patients.
A randomised study was carried out with 200 patients. 100 patients of SSIH with altered consciousness were randomised to enter neurology and 100 to enter neurosurgery right from emergency. It turns out that 10 patients in the surgery arm died before surgery could be arranged, and 10 died after surgery. In the medical arm, 20 patients died in total.
The question is how to analyse the data – mainly how to analyse the deaths prior to surgery in the neurosurgery arm? If we ignore them, surgery looks better because 20 % died in medical and 10 % in surgical. If we include them in medical arm, surgery looks much better – 30/110 deaths in medical and 10/90 in surgical. If we count them as death in surgical arm, both medical and surgical arm looks similar – 20 % death in each arm.
The answer has to do with what will happen with the conclusions. If we conclude surgery is better, then all such patients will go to neurosurgery right from emergency (a new policy). If the study represents what normally happens, 10 % (or more) are likely to die before surgery, whereas 10 % will die after surgery. The mortality will be 20 %. Even with the present policy, the mortality is the same. Thus, the outcome for the hospital will remain the same but with a costly approach of surgery in at least 90 % of patients. In other words, the conclusion was falsely positive in favour of surgery. If we want to know what results to expect with change of policy from medical to surgical, then everything, which happens after randomisation to an arm, must be counted on that arm. The deaths occurring before surgery have to be counted in the surgical arm because this is what is likely to happen in real settings. Thus, the medical arm will have 20 % mortality and so will the surgical arm.
An analysis which counts all outcomes pertaining to an arm in a randomised trial in that arm only irrespective of whether the patients receive the intervention or not is called an ‘analysis based on intention-to-treat principle’.
What Does It Tell Us?
It tells us what outcomes to expect with one policy versus another. It tells us what happens with a policy under real (usual) circumstances. In other words, what does happen or what does an intervention do? This is often different from what can an intervention do. (Please see below).
Another Example
A new drug to reduce cholesterol came into being. A study to assess the effectiveness and safety of the new drug randomised 1,103 patients to the treatment arm and 2,789 to placebo arm [1]. In the treatment arm, 746 patients complied to the protocol (treatment), of which 112 (15 %) died, whereas in the placebo group 585 (20.9 %) died, a statistically significant difference (P value = 0.0003). Analysed in this way, you may conclude that the treatment is effective in reducing mortality. However, this is a biased analysis. You have taken all patients in the placebo arm and only compliant ones in the treatment arm. In placebo arm also, there were compliants and non-compliants. The compliants in the placebo arm also had only 15.1 % mortality, practically no difference from those in the treatment arm. If you compare compliant patients only in both the treatment and placebo arm, there is no difference. If you compare all patients in the treatment (mortality 20 %) versus all patients in the placebo arm (mortality 20.9 %), there is practically no difference (P value = 0.55). This last analysis is called ‘intention-to-treat’ analysis.
The question is which of the above analysis is likely to be unbiased. Here you have to remember why, in the first place, you chose a randomised design. You did so because randomisation tends to balance the prognostic factors between the two arms on an average. Having done it, you must take its advantage. The only analysis which allows you to take the full advantage of randomisation is intention-to-treat analysis, that is, attributing all patients (and their outcomes) to the arm to which they were randomised, irrespective of whether they actually received their assigned treatment or not.
Why Intention-to-Treat Analysis?
If you analyse only the compliant patients, you are likely to get biased results. Why? The reason is that the compliant patients in the two arms may not be prognostically balanced.
You would mix up the effects of treatment and bias introduced by prognostic imbalance. Even if you show balance in known prognostic factors, there is no guarantee that unknown prognostic factors will be balanced. Therefore, the only analysis, which takes full advantage of a successful randomisation, is the one based on intention-to-treat principle. If you don’t, you may lose the benefits of randomisation and also the strength of a randomised design. Some experts say you convert a randomised study to a cohort one.
Principle of Intention to Treat (True ITT Analysis) Versus Intention-to-Treat Analysis (Quasi-ITT)
Guyatt and his group distinguish between the principle of intention to treat and the common usage of the term intention-to-treat analysis [2]. The principle of ITT requires that all patients randomised must be included in the analysis in their respective arms. On the other hand, the common usage of the term ‘ITT’ includes violations of the principle, which means withdrawing patients, after randomisation. There are three usual reasons for withdrawal:
(i)
Mistaken eligibility
(ii)
Non-compliance
(iii)
Losses to follow-up
(i)
Mistaken eligibility: Consider a trial of steroids in acute bacterial meningitis, in which some cases of viral (aseptic) meningitis are also included inadvertently. Withdrawing the latter may not threaten validity greatly, except if they had adverse effects of steroids. As such outcome of aseptic meningitis is universally good, and hence prognostically the withdrawn groups are likely to be balanced. No major threat to validity arises as the prognostic balance is not disturbed.
(ii)
Non-compliance: Non-compliance to the treatment to which the patients are randomised arises usually due to the following reasons:
(a)
Adverse effects: more often in experimental treatment group than placebo.
(b)
Perceived lack of efficacy: if the treatment is ineffective and patient is deteriorating.
(c)
Negligent behaviour of the patients: this is likely to be distributed equally in both the groups.
It is evident that the non-compliance due to (a) and (b) reflects on the risk–benefit profile of the new treatment and hence should not be neglected. In fact, this in itself can be one of the important outcomes to be analysed. Removing the non-compliant patients may in fact paint a rather unduly favourable picture of the new treatment.
(iii)
Losses to follow-up: This will be discussed under adequate follow-up in the next chapter.
From the above it is clear that non-compliant patients need to be included in the analysis and there should (ideally) be no losses to follow-up. All patients need to be included in the respective arms in the final analysis.
Limitations of ITT
You might have noticed that there are some problems in the concept of ITT. The benefit (and adverse effects) of the treatment can be experienced only by those who take it. If only 50 % of the patients comply with the treatment and all patients are counted in the analysis, then there is bound to be dilution of the effects, both beneficial and adverse, of the treatment. This will result in underestimation of the effects (sometimes, with two active treatments under comparison, there can be overestimation of the effects). Yes, this is right. ITT commonly results in underestimation of effects.
Is there a way to resolve this problem? Some experts think that analysing only the compliers (per-protocol analysis) can solve this problem. This is not right. Compliers in the two groups may not be similar. In the control group, moderately sick may leave the study to seek other treatments, while in the treatment group, such patients may benefit and therefore remain compliant, while some may develop adverse effects and leave. Thus, the kind of patients in the two groups remaining compliant would differ in prognosis. Thus, per-protocol analysis will give biased results. Experts are still working on methods, which will give the estimate of effects of treatment with 100 % compliance.
Note: Most drug controlling agencies (like FDA of USA) insist on ITT analysis for approving new drugs. The reason must be obvious to you. ITT analysis protects against biased results. This is also the reason why editors insist on ITT analysis for publishing a paper.
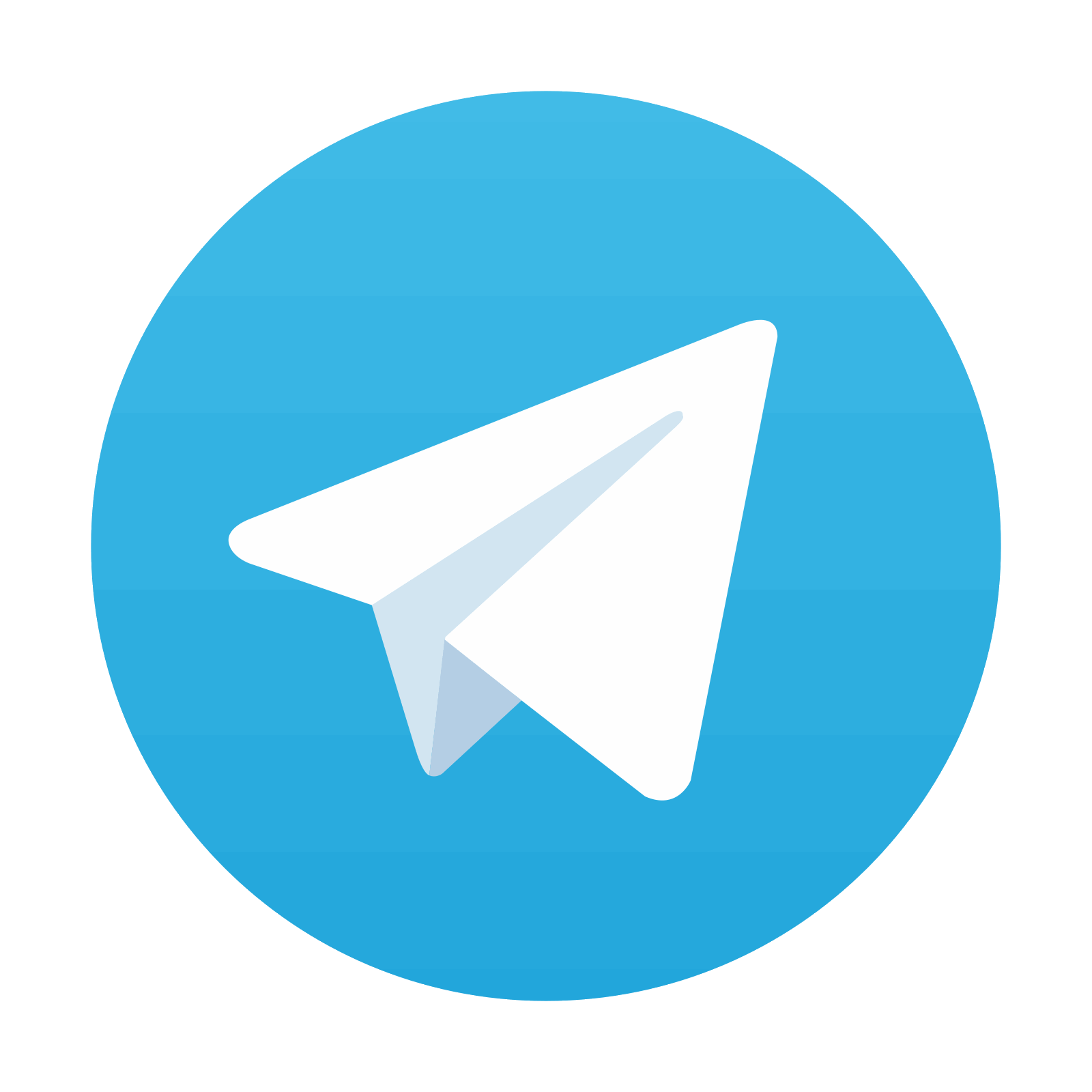
Stay updated, free dental videos. Join our Telegram channel

VIDEdental - Online dental courses
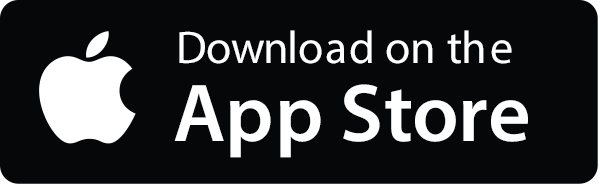
