Highlights
- •
Simple randomization leads most of the time to unequal group sizes.
- •
Simple randomization is suggested for large samples, and block randomization is suggested for smaller samples.
- •
Sample size influences the power more than the group imbalance.
It is virtually impossible to control all the factors that may confound results in research. Factors unknown to the researcher can create differences between groups that are not related to the primary factor under analysis. Randomization is perhaps one of the best ways to try to overcome such unknown factors. It homogenizes groups so that each specimen, participant, or intervention has the same chance of being allocated to each experimental group. Therefore, it is important to include randomization in experimental designs of orthodontic studies when possible.
Imagine a research project where 2 adhesive systems from 2 manufacturers are being compared for shear bond strength. The researcher may collect data of the adhesive system from manufacturer A in the morning and from manufacturer B in the afternoon. It is possible that the researcher might be tired toward the end of the day and therefore could, unconsciously, be less precise when collecting data. Thus, if differences were found between the groups, would those be attributed only to the adhesive system or to the researcher’s fatigue as well?
Similarly, imagine a clinical study aiming to compare the bracket debonding rates using 2 composites. To compare them in the same patient in a split-mouth design, the researcher could bond the right-side brackets with composite A and the left-side ones with composite B. Because the orthodontist might have a different perspective between the patient’s right and left sides, the bonding quality could be slightly different between the sides, and this may result in differences that are not really attributed to the composites. This could undermine the results because it is expected that differences between 2 interventions are explained only by the factors being evaluated.
In these 2 examples, one might think that the biases mentioned could be controlled if the adhesive systems (in the in-vitro test) or composite types (in the in-vivo test) were evaluated or applied in an alternate manner. Even though this idea may seem interesting, it could also lead to selection bias: eg, if the operator knows which adhesive is being evaluated, a systematic bias might be introduced. Besides eliminating biases and giving all specimens the same chance of allocation to any of the groups, randomization is a fundamental premise that justifies most of the statistical procedures used in data analysis.
Thus, any attempt at randomization that has a logical pattern of allocation and that deviates from pure chance (eg, chart identification numbers, day of the week, date of birth) can possibly, even if unconsciously, introduce unknown factors into the groups being studied. This could confound the intervention investigated and compromise the research results.
In orthodontics, these problems are usually circumvented by generating random numbers that do not follow any pattern by a method called simple randomization. Specific softwares or functions, such as RANDBETWEEN (Excel; Microsoft, Redmond, Wash), are normally used to produce a list of random numbers that leave all expected or unforeseen biases to chance alone.
Nevertheless, simple randomization has a significant problem in studies with small sample sizes, because there is a high probability that the groups will be in a state of imbalance ; in most of the current orthodontic literature, sample sizes are usually small. Orthodontic randomized controlled trials published from 1992 through 2012 were shown to have a median sample size of 46 to obtain adequate power, and a median sample size of 60 was necessary to produce results. However, the percentages of these studies with groups in a state of imbalance, or whether they used any methods to restrict randomization, decreasing the chance of producing groups in a state of imbalance, are unknown. This imbalance can produce differences in distribution and variances, decreasing the statistical power.
One way to overcome this problem is to use block randomization, which produces equal numbers of specimens in each group. In such a method, the specimens are distributed into blocks of multiple numbers related to the number of groups under study, containing all possible combinations of allocation but maintaining a 1:1 balance. Thus, in the aforementioned clinical research example, when determining which composite, A or B, will be tested on the right or left side of 24 patients in a split-mouth design, 4 allocations are arranged into 6 blocks (AABB, BBAA, BABA, ABAB, ABBA, and BAAB). These blocks will then undergo simple randomization to determine the sequence in which they will be applied until all patients are bonded. The investigator must be careful, though, when using blocks of the same size, which are easier to manage, because that could lead to a prediction of which treatment will be allocated next. Different block sizes can be used to overcome that issue.
Therefore, the aim of this article was to determine when block or simple randomization is necessary, based on the probability of imbalance between groups and on the influence of the statistical power.
Material and methods
Four hypothetical research designs were analyzed, varying the numbers of subjects (20, 30, 60, and 90) allocated into 2 groups, using an independent 2-tailed t test, with α = 0.05, by simple randomization using the RANDBETWEEN function of the Excel 2011 software.
A total of 100 allocation simulations were made for each research design to describe the differences between specimens in the groups, their frequencies, and the balance ratios. Statistical power was also calculated for each balance ratio using the G*Power software ( http://www.gpower.hhu.de/en.html ). The effect sizes were varied from small and medium to large using Cohen’s d (d = 0.2, d = 0.5, and d = 0.8, respectively) and the aforementioned parameters.
The values obtained with block randomization simulations, with a fixed equilibrium ratio of 1:1, were compared with those using simple randomization.
Results
When simulating with 20 specimens, 17% of the simulations showed a ratio of 1:1 between the samples. On the other hand, 70% of the simulations showed imbalances from 1.2:1 to 1.9:1, which caused a maximum reduction of 10% of the test power to evaluate large effects, but less than 1% when medium or small effects were evaluated. The remaining 13% of the simulations had larger imbalances (from 2.3:1 to 5.7:1), with gradually decreasing test power. This decrease was marked especially when a large effect was used in the simulation, causing a 17% decrease ( Table I ). The decrease in test power with imbalanced samples occurred in all effect sizes, with greater reductions for larger effect sizes. In block randomization, all groups have a 1:1 equilibrium rate; thus, the test power will be 40% with large effect sizes, 19% with medium effect sizes, and 7% with small effect sizes.
G1 | G2 | Imbalance | Imbalance ratio | Frequency | Power (%) | ||
---|---|---|---|---|---|---|---|
d = 0.8 | d = 0.5 | d = 0.2 | |||||
10 | 10 | 1:1 | 17% | 40 | 19 | 7 | |
11 | 9 | 0.8 | 1.2:1 | 25% | 39 | 18 | 7 |
12 | 8 | 0.7 | 1.5:1 | 25% | 38 | 18 | 7 |
13 | 7 | 0.5 | 1.9:1 | 20% | 37 | 17 | 7 |
14 | 6 | 0.4 | 2.3:1 | 6% | 34 | 16 | 7 |
15 | 5 | 0.3 | 3:1 | 4% | 31 | 15 | 7 |
16 | 4 | 0.3 | 4:1 | 2% | 27 | 14 | 6 |
17 | 3 | 0.2 | 5.7:1 | 1% | 23 | 12 | 6 |
Only 8% of the simple randomization simulations with 30 specimens showed balanced groups (1:1). In the remaining simulations, imbalance ranged from 1.1:1 to 2.3:1, with maximum decreases of 7% in the test power with a large effect size, 3% with a medium effect size, and less than 1% with a small effect size. In all effect sizes, the test power dropped, but the drop was more pronounced when a large effect size was used. As in the previous simulation, block randomization groups had a 1:1 equilibrium, but the test power was higher for these comparisons. Test powers were 56%, 26%, and 8% for large, medium, and small effect sizes, respectively ( Table II ).
G1 | G2 | Imbalance | Imbalance ratio | Frequency | Power (%) | ||
---|---|---|---|---|---|---|---|
d = 0.8 | d = 0.5 | d = 0.2 | |||||
15 | 15 | 1:1 | 8% | 56 | 26 | 8 | |
16 | 14 | 0.9 | 1.1:1 | 30% | 56 | 26 | 8 |
17 | 13 | 0.8 | 1.3:1 | 18% | 55 | 26 | 8 |
18 | 12 | 0.7 | 1.5:1 | 19% | 54 | 25 | 8 |
19 | 11 | 0.6 | 1.7:1 | 12% | 53 | 25 | 8 |
20 | 10 | 0.5 | 2:1 | 9% | 51 | 24 | 8 |
21 | 9 | 0.4 | 2.3:1 | 4% | 49 | 23 | 8 |
With 60 specimens, only 10% of the simulations had balanced groups, which resulted in an 86% power in a test with a large effect size. In the remaining simulations of large effect size, test power was above 80% even when the imbalance was 2.2:1. When a medium effect size was used, the maximum decrease of the test power was 5% (from 1:1 to 2.2:1); in small effect sizes, the test power decreased by only 1%. In the block randomization, the groups had a 1:1 equilibrium rate, and the test power values were 86%, 48%, and 12% with large, medium, and small effect sizes, respectively ( Table III ).
G1 | G2 | Imbalance | Imbalance ratio | Frequency | Power (%) | ||
---|---|---|---|---|---|---|---|
d = 0.8 | d = 0.5 | d = 0.2 | |||||
30 | 30 | 1:1 | 10% | 86 | 48 | 12 | |
31 | 29 | 0.9 | 1.1:1 | 14% | 86 | 48 | 12 |
33 | 27 | 0.8 | 1.2:1 | 18% | 86 | 47 | 12 |
34 | 26 | 0.8 | 1.3:1 | 23% | 86 | 47 | 12 |
35 | 25 | 0.7 | 1.4:1 | 7% | 85 | 47 | 12 |
36 | 24 | 0.7 | 1.5:1 | 7% | 85 | 46 | 12 |
37 | 23 | 0.6 | 1.6:1 | 3% | 84 | 46 | 11 |
39 | 21 | 0.5 | 1.9:1 | 4% | 83 | 44 | 11 |
41 | 19 | 0.5 | 2.2:1 | 1% | 81 | 43 | 11 |
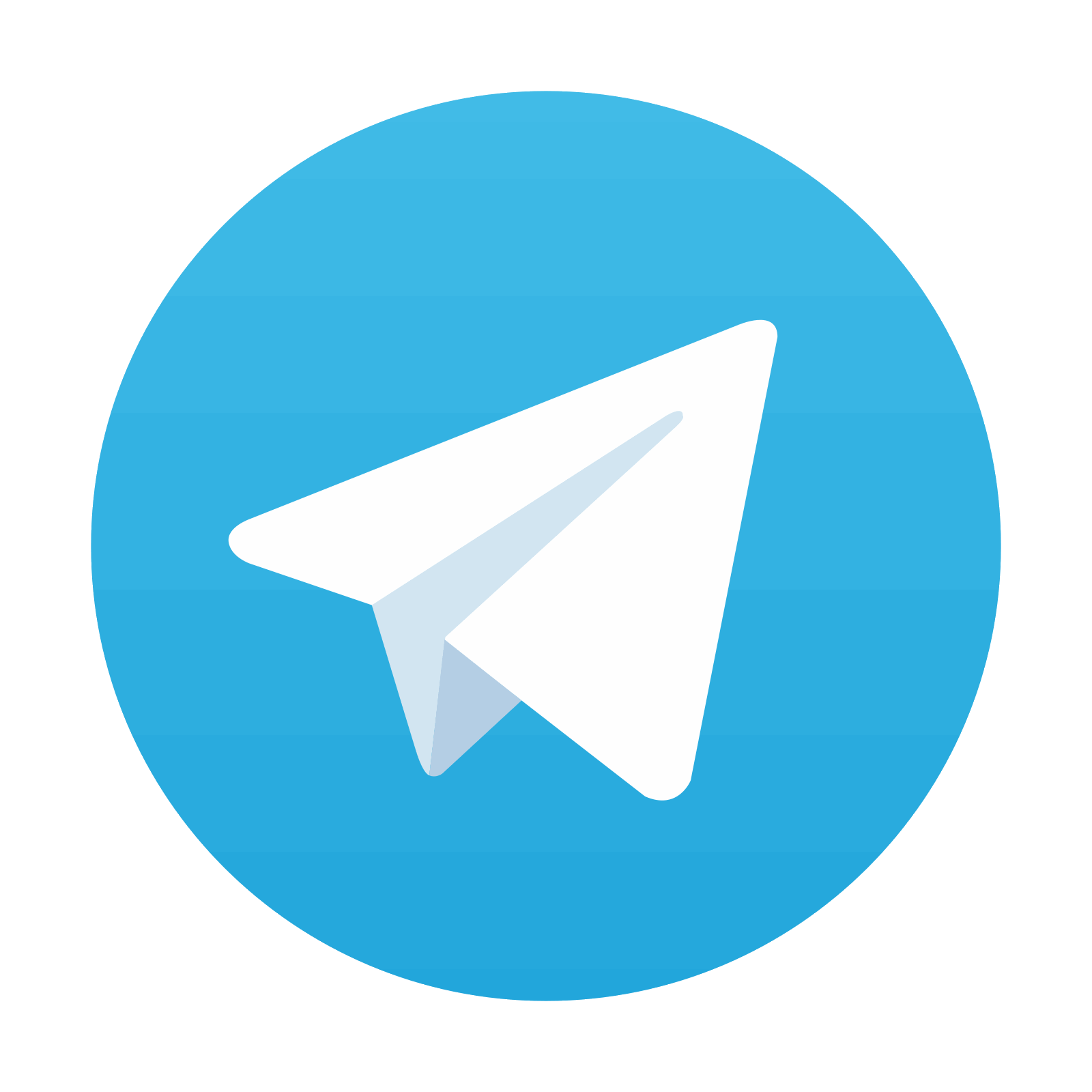
Stay updated, free dental videos. Join our Telegram channel

VIDEdental - Online dental courses
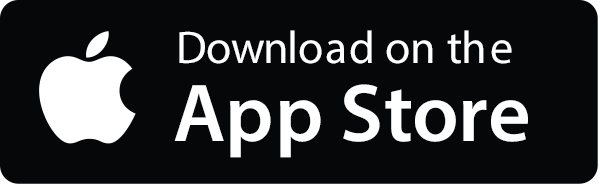

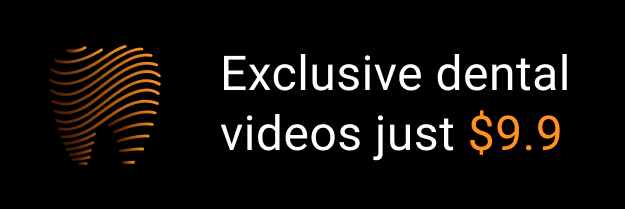