Diagnostics and Epidemiology: 7 Caries Risk Assessment and Prediction
The terminology associated with assessing or predicting future development of caries can easily lead to confusion. Throughout this chapter we will define and use only very few terms and show their practical utility in dental clinics. Caries risk can be defined as the probability of an individual developing at least a certain number of carious lesions reaching a given stage of disease progression during a specified period, conditional on the exposure status remaining stable during the period at issue.1–3 Caries risk assessment or caries prediction can be based on several predictors, namely risk factors, risk indicators, and risk markers: the first is involved in the caries process, the second and third are only associated with the process itself. We will use the terms predictor and risk factors in this chapter and both terms can mean environmental, behavioral, or biological factors.
This chapter will cover:
-
the following concepts in caries prediction: prevalence, sensitivity, specificity, crude hit rate, expected utility, and receiver operating characteristics (ROC) curves;
-
the usefulness of various predictors such as past signs of caries experience;
-
the Cariogram computer program for caries prediction;
-
the value of early caries diagnosis for the preventive treatment planning for individual occlusal surfaces; and
-
the Nexø program.
The Dentist′s Clinical Judgment
For the benefit of the patients and a responsible use of resources, dentistry should avoid both overtreatment and undertreatment as much as possible. For caries preventive measures this means that the dental professional should try to differentiate between those people who will develop caries, including new lesions in addition to existing ones (high risk: high focus on prevention), and those who will not develop new caries (low risk: no or minor focus on prevention). In daily practice this prediction is frequently based on the clinical judgment of the dental professional. What is this prediction worth?
NOTE
Caries risk is the probability of an individual developing new caries lesions during a specified period.
In a study where 15 dentists were asked to predict whether children would develop dentinal caries lesions needing restoration within the next year, it turned out that dentists recognized 35%–79% of the children who developed dentinal caries and 75%–97% of the children who remained free of new caries into the dentin.4,5 The terminology for this is that dentists showed a sensitivity, meaning the ability to recognize those who would develop dentin caries (the disease) varying from 35% to 79%, and a specificity, meaning the ability to recognize those who will not develop dentin caries (no disease) of 75%–94% ( Table 7.1 ). When looking at the individual dentists, the one (dentist A) with the lowest sensitivity (35%: the dentist recognized 35% of those who later developed dentinal caries) recognized 92% of those who remained free of caries into the dentin (specificity 92%) and the one (dentist B) with the highest sensitivity (79%) showed a specificity of 75%. It was interesting to note that the prediction tended to be only slightly better if the dentist had treated the same children for several years as compared with when the prediction was done at first examination; the average sensitivity of the dentists increased from 40% to 50%, but the average specificity remained at about 90%.4 When the children were without cavities or restorations at baseline (DMF = 0, see Chapter 8) the mean sensitivity decreased to 23%, indicating a high number of false negative predictions (dentin caries is present but the clinical judgment failed to predict it) suggesting that the dentists were misled by the caries-free status.
Based on the above, several questions arise:
-
Should (these) dentists follow their clinical judgment when selecting caries-active children for preventive measures?
-
What do the differences between the se dentists A and B mean for their patients? Would you rather be a patient of dentist A or of dentist B?
-
Which type of dentist are you, yourself?
-
Are there any tests using risk factors systematically that perform better than the clinical judgment of these two dentists?
In spite of the mass of literature on caries prediction, the last question is difficult to answer because the outcome measures of the studies differ in the amount of caries to predict and the periods over which the predictions are made. In discussing the differences between dentists A and B, this chapter will introduce crude hit rate and expected utility, two methods to evaluate the performance of clinical judgment, and frequently suggested tests to predict caries and compare their performances against using no test at all.
Crude Hit Rate of a Predictor
There are different methods to express the accuracy of the prediction—for example, the sensitivity, specificity, and the crude hit rate. All of them require that data are dichotomized, meaning that data are structured in a two-by two table where, for example, the prediction is divided into “caries increment expected” or “no caries increment expected” and the actual caries increment at a certain threshold level (the truth observed during the study) is yes or no ( Table 7.1 ). The crude hit rate is the number of true positive predictions plus the number of true negative predictions divided by the total number of predictions ( Table 7.1 ). The crude hit rate can be expressed as a percentage by multiplying the fraction by 100%.

Figure 7.1 shows the crude hit rates (in this case the percentage of true allocations of preventive measures) in relation to the prevalence of caries development when preventive measures are either routinely or never applied. If, for example, 20 out of 100 persons who all routinely are given preventive measures developed dentin caries (prevalence of caries development: 20%), the crude hit rate of this strategy is 20. So in case of routine preventive measures the crude hit rate equals the prevalence of caries development. On the other hand, if none of the persons received preventive measures and 20 out of 100 were to develop caries, the crude hit rate is 80%: thus 80% (1 − prevalence of caries development) of the persons correctly receive no treatment.
BACKGROUND
In this chapter we use the term “prevalence of caries development” instead of “caries incidence” to correspond better to the 2×2-table presented in Table 7.1 .
When only the number of good decisions counts and they count equally, strategies with high crude hit rates are better than strategies with lower crude hit rates. It is clear that when the prevalence of caries development is above 50% it is better to apply preventive measures routinely rather than never, but when the prevalence is below 50%, it is better never to apply a preventive measure than to do it routinely.
The crude hit rate can also be estimated for dentist A and dentist B; however, it requires calculations, as we initially only know the sensitivity and specificity of the dentists and the prevalence of caries development. The number of true positive predictions (TP) equals the prevalence × sensitivity ( Table 7.1 ), and the number of true negative predictions (TN) equals (1 − prevalence) × specificity. Thus, the number of true positive and true negative predictions can be calculated for dentist A, remembering that his sensitivity was 35% and specificity was 92%, and for dentist B for whom the sensitivity was 79% and the specificity 75%. The crude hit rates for dentists A and Bare calculated in Table 7.2 for increasing prevalence of caries development. For example, if the prevalence of developing dentin caries is 50%, the number of true positive predictions of dentist A is 50 × 0.35 = 17.5 and his number of true negative predictions is 50 × 0.92 = 46 and added together the crude hit rate is 63.5. In Fig. 7.2a, b the crude hit rates of dentists A and B are added to the crude hit rates when preventive measures are given to all or to none of the patients as depicted in Fig. 7.1 . Again, when strategies with high crude hit rates are better than strategies with lower crude hit rates, it is clear that dentist A should not use his clinical judgment when caries prevalence is less than 18% (crude hit rate <83 against the crude hit rate for never doing prevention at > 84), and dentist B should not when caries prevalence is below 25%. Under those conditions, in practices with these low prevalences of caries development, the dentists should follow the strategy of never applying the preventive strategy. At higher prevalences, >18% and >25% respectively, dentists A and B should use their clinical judgment. However, when prevalences of caries development increase above 60% or 78%, dentists A and B, respectively, should no longer use their clinical judgment but always apply the preventive strategy. When superimposing Fig. 7.2a, b , it can be noted that with prevalences <28%, the crude hit rate of dentist A is higher than that of dentist B ( Fig. 7.2c ). So dentist A, then, would be the more accurate dentist. With prevalences >28%, the crude hit rate of dentist B is higher than that of dentist A, thus making dentist B the “better” dentist.
NOTE
When only the number of good decisions counts and they count equally, strategies with high crude hit rates are better than strategies with lower crude hit rates.
Utilities of Predictors
The above outcome, viz. that dentist A is better than dentist B when caries development prevalence is below 28%, relates not to the fact that dentist A has more true positive predictions, but to the fact that dentist A has more true negative predictions (see columns in Table 7.2 ). One can argue that true positive predictions (patients who will develop new caries are identified) are more important than true negative predictions (patients who will not develop new caries are identified). Then, in the formula of the crude hit rate a higher value can be given to the true positive prediction compared with the true negative prediction. This will change the comparison between dentist A and dentist B. A numerical value given to a (health) outcome is called a utility. Utilities can also be given to false positive and false negative predictions. Those predictions are wrong and that should be reflected in the utility assessment.
NOTE
A numerical value given to a (health) outcome is called a utility. Utilities can be given to true and false positive and true and false negative outcomes (TP, TN, FN, FP predictions). Unfortunately, there are no generally accepted values for utilities that should be assigned to the outcomes of a predictor.
Unfortunately, there are no generally accepted values for utilities that should be assigned to the outcomes of a predictor (TP, TN, FN, FP calls). It may be clear that dental professionals, patients, and finance officials may disagree on the utilities that should be given. In Table 7.3 suggestions are made for utilities, and these values are used in the calculations in this chapter. If readers do not agree with these values and want to change the ratio between the values, they have to redo the calculations in this chapter with their own values. By doing so, such a reader may reach other conclusions than those of the author.
When utilities are assigned, the expected utility (EU) of a prediction or predictor can be calculated (see Table 7.1 ). In Table 7.4 the expected utilities of dentist A are calculated (using the formula presented in Table 7.1 ), where the values for true positives are multiplied by the numerical value 2, true negatives by 1, false positives by −1and false negatives by −2. The justification of these multipliers is addressed in Table 7.3 .
The same calculations can be made for the strategies “treat never” and “treat routinely” for dentist B. The outcomes of these calculations are depicted in Fig. 7.3 , which shows that up to a prevalence of 10%and 15 % for dentists A and B, respectively, both dentists should go for the “no preventive treatment” strategy. From a prevalence of above 10% and 15% to, respectively, 42% (dentist A) and 65% (dentist B), they should follow their own judgment and above those prevalences they should follow the “treat all” strategy. When superimposing the expected utilities of dentist A and dentist B, it can be seen ( Fig. 7.3c ) that with a prevalence <18% dentist A is the more accurate dentist, and with a higher prevalence of caries development dentist B is the more accurate.

NOTE
When utilities are assigned, the expected utility (EU) of a prediction or predictor is the sum of the products of the percentages TP, FN, TN and FP and their assigned utilities.
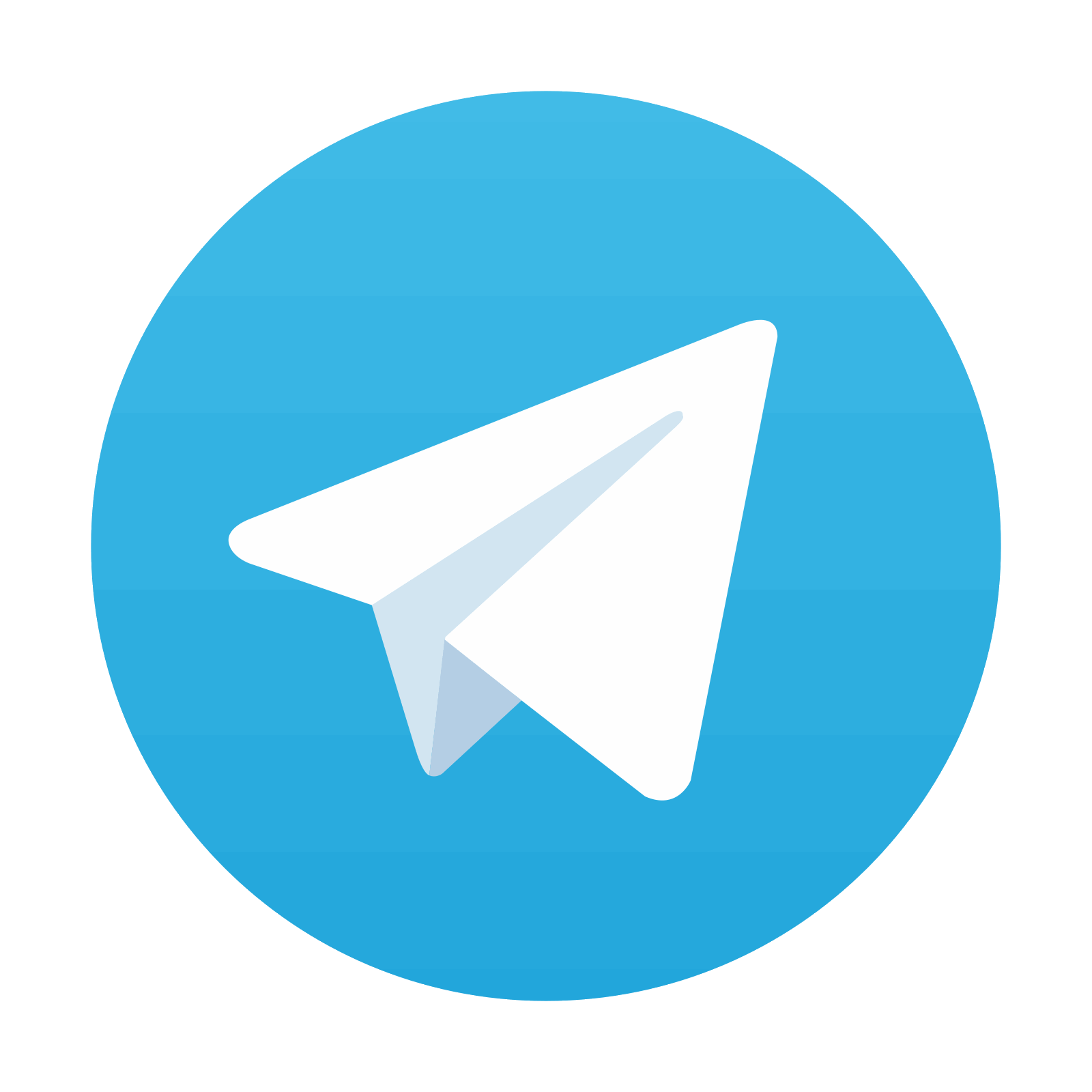
Stay updated, free dental videos. Join our Telegram channel

VIDEdental - Online dental courses
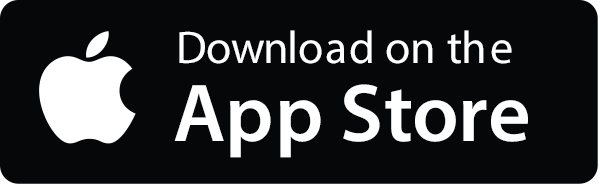

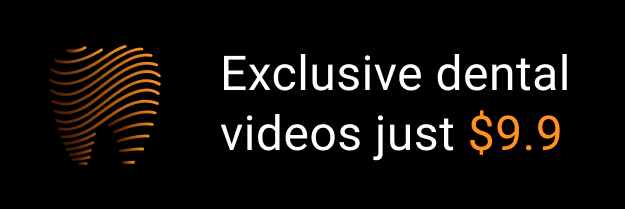