We have previously discussed the use of conventional logistic regression when our aim is to assess binary or, in other words, dichotomous categorical outcomes. That is when the possible answers after examining the outcome variable can be either “yes” or “no.” One such example in orthodontics is crowding alleviation after the use of different therapeutic approaches. Crowding alleviation can be either resolved—“yes”—or not resolved—“no.”
However, we are frequently confronted with questions that cannot be answered with a simple yes or no; a range of possible responses may apply. When these are characterized by a gradient of ranking across the levels of possible responses, then they are called ordered categorical variables . In such cases, ordinal logistic regression can be applied in the same way that conventional logistic regression is used for binary outcome variables.
We will use a simple example to show how ordinal logistic regression can be used in practice. Imagine that we would like to test patient satisfaction after the use of either activator or cervical headgear in a cohort of Class II Division 1 children. We have asked the children to rate how they feel about wearing the appliance in terms of: “unhappy,” “somewhat happy,” or “very happy.” Table I shows their responses across the 2 groups.
Level of satisfaction | Type of appliance | Total | |
---|---|---|---|
Activator, n (%) | Headgear, n (%) | ||
Unhappy | 30 (60) | 14 (28) | 44 (44) |
Somewhat happy | 14 (28) | 25 (50) | 39 (39) |
Very happy | 6 (12) | 11 (22) | 17 (17) |
Total | 50 (100) | 50 (100) | 100 (100) |
We can see that a higher percentage of children wearing headgear reported that they are either somewhat happy or very happy with their appliance, unlike those wearing an activator, where most children are unhappy (n = 30; 60%).
We may then use ordinal logistic regression to identify the effect of the type of appliance on the level of satisfaction and draw the estimate of the effect with confidence intervals ( Table II ).
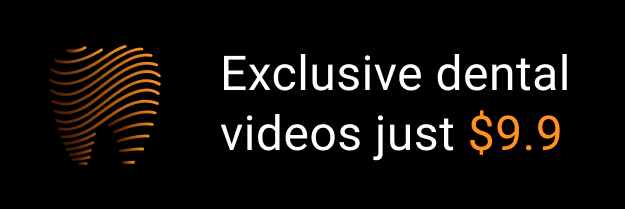