Introduction
Currently, few methods are available to measure orthodontic treatment need and treatment outcome from the lay perspective. The objective of this study was to explore the function of an eye-tracking method to evaluate orthodontic treatment need and treatment outcome from the lay perspective as a novel and objective way when compared with traditional assessments.
Methods
The scanpaths of 88 laypersons observing the repose and smiling photographs of normal subjects and pretreatment and posttreatment malocclusion patients were recorded by an eye-tracking device. The total fixation time and the first fixation time on the areas of interest (eyes, nose, and mouth) for each group of faces were compared and analyzed using mixed-effects linear regression and a support vector machine. The aesthetic component of the Index of Orthodontic Treatment Need was used to categorize treatment need and outcome levels to determine the accuracy of the support vector machine in identifying these variables.
Results
Significant deviations in the scanpaths of laypersons viewing pretreatment smiling faces were noted, with less fixation time ( P <0.05) and later attention capture ( P <0.05) on the eyes, and more fixation time ( P <0.05) and earlier attention capture ( P <0.05) on the mouth than for the scanpaths of laypersons viewing normal smiling subjects. The same results were obtained when comparing posttreatment smiling patients, with less fixation time ( P <0.05) and later attention capture on the eyes ( P <0.05), and more fixation time ( P <0.05) and earlier attention capture on the mouth ( P <0.05). The pretreatment repose faces exhibited an earlier attention capture on the mouth than did the normal subjects ( P <0.05) and posttreatment patients ( P <0.05). Linear support vector machine classification showed accuracies of 97.2% and 93.4% in distinguishing pretreatment patients from normal subjects (treatment need), and pretreatment patients from posttreatment patients (treatment outcome), respectively.
Conclusions
The eye-tracking device was able to objectively quantify the effect of malocclusion on facial perception and the impact of orthodontic treatment on malocclusion from the lay perspective. The support vector machine for classification of selected features achieved high accuracy of judging treatment need and treatment outcome. This approach may represent a new method for objectively evaluating orthodontic treatment need and treatment outcome from the perspective of laypersons.
Highlights
- •
Eye tracking technique was used to record scanpaths of observers viewing repose and smiling images of pretreatment orthodontic patients, posttreatment malocclusion patients and subjects with normal occlusion.
- •
First fixation time and total fixation time of areas of interest (eyes, nose, and mouth) were analyzed.
- •
Scanpaths of pretreatment patients differed significantly from those of posttreatment patients and normal subjects.
- •
SVM classification of selected eye tracking data achieved high accuracy of judging orthodontic treatment need and treatment outcome.
Treatment need for patients with malocclusion and the outcome of orthodontic treatment are typically evaluated by orthodontists or patients. Orthodontists use cephalometry, model analysis, and the Index of Complexity, Outcome and Need (ICON) in their evaluations. Self-evaluation methods from the perspective of the patient include the Psychosocial Impact of Dental Aesthetics Questionnaire and the aesthetic component of the Index of Orthodontic Treatment Need. Although these methods are effective at assessing treatment need, treatment outcome, and patient psychology, they do not evaluate the perspectives or opinions of a third party. Additionally, the available methods do not help clinicians to understand the origin of social deprivation among malocclusion patients and how casual observers view them. Because one motive for seeking treatment among malocclusion patients is to change how they are perceived by others, and because treatment outcome is evaluated not only by patients and orthodontists but also by society, we propose an objective and sensitive method for evaluating the perspectives of laypersons as a supplement to current assessment tools to improve the evaluation of orthodontic treatment need and treatment outcome.
It is well established that eye movements are a surrogate of attention. The eye-tracking technique has been widely used in facial studies, such as studies of facial expression, sex, and race judgment. The major advantage of this technique is that it can record the movements of the eyeballs while multiple stimuli compete for attention. The changing hierarchy of attention is considered to reflect cognitive strategies for extracting facial information. Previous eye-tracking research has characterized the scanpaths of casual observers when viewing normal faces. The typical scanpath pattern for normal faces is triangular. Observers place most of their attention on the internal facial features, such as eyes, nose, and mouth, and converging evidence on the typical scanpath pattern has been obtained. In a study of the attention placed on the internal facial features, observers spent most of their time (43%) on the eyes and 13% of their time on the mouth. A subsequent study examined the characteristics of the scanpaths of casual observers viewing the faces of dental patients. Hickman et al concluded that patients with a perfect smile after orthodontic treatment received only approximately 10% of the attention of the observers. More of the initial fixations were on the mouth, and fixations on the mouth and nose regions were longer when observers were viewing patients with a cleft lip and palate than when they were viewing the control faces. This finding indicates that faces with anomalies cause changes to the typical scanpath pattern. Additional studies have demonstrated that observers spent more time on the facial structure that they perceived to be abnormal when viewing pretreatment faces and that the scanpath was normalized when viewing posttreatment faces. Researchers have proposed a novel method for objectively evaluating observer attention as an indicator of the success of surgical procedures to help minimize the appearance of facial deformities. Studies of the eye-tracking pattern of observers of faces with anomalies and how surgery can normalize the scanpath have provided insight into the evaluation of the effectiveness of facial reanimation surgery. By comparing pretreatment patients, posttreatment patients, and normal subjects, we can determine how orthodontic treatment can change the scanpath of observers, and the results of such studies maybe useful for the evaluation of orthodontic treatment.
Machine learning is a subfield of computer science that allows for predictions of data by building a model from given inputs. The support vector machine (SVM), a machine learning method in computer science, is efficient at generalizing to unseen data and making data-driven predictions. Therefore, SVMs are widely used in the early diagnosis and classification of diseases such as Alzheimer’s and colorectal tumors. SVMs have also been used to manage eye-tracking data and boost diagnostic accuracy. The principle of data prediction is that the machine can classify the data by identifying a hyperplane decision boundary with minimal errors by studying the innate features in each data type provided. In this study, the eye-tracking technique was used to quantify the attention bias on malocclusion patients and measure the effectiveness of orthodontic treatment at normalizing the scanpath of observers viewing malocclusion patients. The eye-tracking data of observers viewing normal subjects, pretreatment patients, and posttreatment patients were subsequently studied by SVM to improve the accuracy of assessing treatment need and treatment outcome of malocclusion by modeling eye movement characteristics.
The aims of this study were to (1) quantify attention bias toward malocclusion using the eye-tracking technique, (2) compare scanpath measurements among persons with normal occlusion (normal subjects) and pretreatment and posttreatment malocclusion patients when assessed by laypersons, (3) determine the accuracy of the assessment of orthodontic treatment need and outcome based on the SVM, and (4) explore the general ability of eye tracking in assessing orthodontic treatment need and outcome from the lay perspective. We explored an alternative method of obtaining a third-party assessment of orthodontic treatment need and outcome.
Material and methods
This study was approved by the ethical review board at Sun Yat-sen University, Guangzhou, China (approval number: ERC-[2015]-1). From February to March 2015, a total of 88 participants were recruited via advertisement at Sun Yat-sen University. The inclusion criteria were (1) no visual impairment, (2) older than 18 years of age, (3) no psychological problems (eg, autism, schizophrenia), and (4) not a dental student or dentist. Once recruited, the participants signed the informed consent form, which described the potential harms, outcome assessments, and data analyses, and were subsequently randomly assigned to 1 of the 4 groups using a simple randomization procedure (computerized random numbers). The participants were not told the purpose of the study before the experiment. The participants were financially compensated, and the purpose of the study was thoroughly explained to them after they completed their participation in the research.
All patient images were obtained from the database of the Department of Orthodontics at Guanghua School of Stomatology, Hospital of Stomatology, Sun Yat-sen University. A total of 20 patients were randomly selected (10 male, 10 female) based on the following inclusion criteria: (1) orthodontic treatment was initiated after the eruption of the canines and completed at 13 to 25 years of age; (2) no need for orthodontic-orthognathic treatment; (3) no cleft lip or palate, severe facial anomaly, or facial asymmetry; (4) no special facial characteristics (eg, scars, unusual hairstyle); and (5) pretreatment patients with treatment need, and posttreatment patients with acceptable treatment outcome or no treatment need as assessed by orthodontists and laypersons. Three orthodontic specialists (B.B., B.C., Y.C.) with more than 5 years of clinical experience assessed the images of the pretreatment and posttreatment patients using the ICON. A pretreatment ICON score greater than 42 indicated treatment need. A posttreatment ICON score less than 31 indicated that the treatment was successful. A total of 20 university students with no dental expertise assessed the intraoral photos of the pretreatment and posttreatment patients using the aesthetic component of the Index or Orthodontic Treatment Need. Grades 3 to 10 indicated treatment need, whereas grades 1 and 2 indicated no treatment need. The patients were reassessed 2 weeks later to determine the reliability of the results. The intrarater kappa statistic was 0.86 (95% confidence interval [CI], 0.84-0.88), and the interrater kappa statistic was 0.82 (95% CI, 0.81-0.83). In addition to the 20 patients, 10 normal subjects who were matched for age and sex to the patients were randomly selected from the database of Guanghua School of Stomatology, Hospital of Stomatology, Sun Yat-sen University. The normal subjects were judged by the same 3 orthodontic specialists to have a normal occlusion and no facial anomalies. The repose and smiling images before and after treatment of 20 patients as well as the repose and smiling images of 10 normal subjects were used as the stimuli. Since the familiarity of faces can affect the scanpath, no observer could view the same person in different states. Thus, the images were randomly and equally divided into 4 groups under the condition that only 1 image of each subject could appear in the same trial. To reduce background interference, the images were cropped so that only the head was included. In addition, the images were standardized to 400 × 33 pixels, with a resolution of 96 pixels per inch (Adobe PhotoShop CS6; Adobe Systems, San Jose, Calif). Randomization was achieved using computer-generated randomization.
The Eyelink 1000 eye tracker (SR Research, Ottawa, Ontario, Canada) was used in this study. The images were displayed in the middle of a 17-in screen, and an infrared sensor was placed directly below the screen. The observers viewed the images at a distance of 60 cm, with their heads stabilized using a chin support to eliminate movement. The observers were told that they could freely view the images and that each image would appear for 10 seconds. Nine-point calibration and validation were conducted, and 2 images were used to familiarize the observers with the procedure. Then, the experiment began, and the gaze of the observer was recorded while he or she viewed the images in random order.
Statistical analysis
The eyes, nose, and mouth were each predetermined as an area of interest (AOI) according to anthropometric landmarks. The eye-tracking data were superimposed on the image, and the data for each AOI were examined. The total fixation time and first fixation on an AOI were extracted from the raw data and analyzed by mixed-effects linear regression in Stata 12 software (StataCorp, College Station, Tex) and SVM in MATLAB (version 7.0; MathWorks, Natick, Mass). The alpha level was set at 0.05.
The eye tracking data of 10 control images, 20 pretreatment images, and 20 posttreatment images were statistically analyzed to determine the difference between pretreatment patients and normal subjects as well as pretreatment patients and posttreatment patients. The difference between the normal group and the pretreatment group was considered to indicate treatment need, and the difference between the pretreatment group and the posttreatment group was considered to indicate treatment outcome. The eye tracking data that can be used to separate the pretreatment group from the normal group and the pretreatment group from the posttreatment group were considered features. Feature sets were real subsets (excluding the empty set) of feature 1, feature 2, and so on. Feature sets were analyzed by linear SVM in order to find the eye tracking data that achieve the best classification results. To increase the accuracy of the discrimination between groups, leave-1-out cross-validation of SVM was used. Given that the goal was to distinguish pretreatment patients from posttreatment patients, and pretreatment patients from normal subjects, the procedure included training regarding the algorithms of the normal and pretreatment groups as well as those of the pretreatment and posttreatment groups. For each trial, 1 original data set from each group was used as the test data, and the remaining data were used as the training data. The training data were used to generate a model that was then tested using the test data. The cross-validation trials were repeated 1000 times to obtain better estimates of classifier performance.
A post hoc power analysis was performed using G*power (Heinrich Heine University, Düsseldorf, Germany). The effect size of each model was calculated, and the minimal effect size (f) was 0.131, which was the f for the comparison of total fixation time on the nose in the posttreatment group to that in the normal control group. The type I error was set at 0.05. The total sample size was the number of observers multiplied by the number of images viewed, and this value was 440. With an effect size of 0.131, a type I error of 0.05, a total sample size of 440 and 2 groups, the minimum statistical power was calculated to be 0.783 using G*power.
Results
A total of 90 laypersons applied for participation in the study (48% male, 52% female; age range, 18-30 years [24.3 ± 4.6 years]). Two laypersons were excluded because they failed to complete the validation and calibration steps.
The ages of the patients (10 male 10 female) ranged from 15 to 24 years (18.8 ± 2.4 years). From 2009 to 2013, the same orthodontist (B.B.) performed orthodontic treatments using a straight wire appliance. The subjects with normal occlusion consisted of 5 men and 5 women, whose ages ranged from 18 to 22 years (20.1 ± 1.2 years).
Heat maps of repose and smiling images of the normal occlusion subjects and the pretreatment and posttreatment patients are presented in Figure 1 .
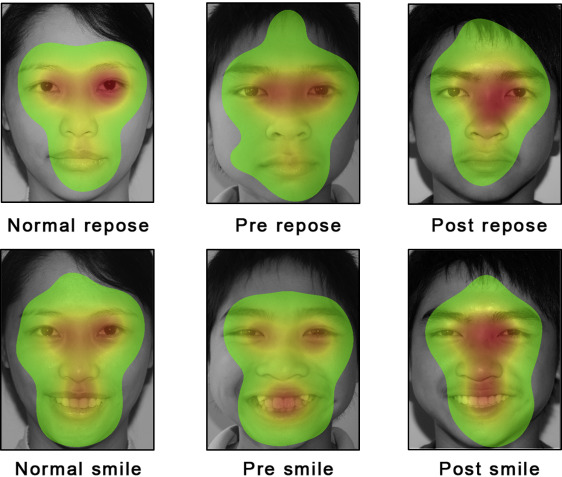
Total fixation time and first fixation time were chosen as parameters to analyze the observation differences between the groups. The total fixation time for each AOI was the total time spent on that AOI. For the smiling and repose faces of normal subjects and repose faces of pretreatment patients and posttreatment patients, the AOI with the longest total fixation time was the eyes, followed by the nose and the mouth. In contrast, for the smiling faces of the pretreatment and posttreatment patients, the eyes had the longest total fixation time, followed by the mouth and then the nose. Compared with normal smiling subjects, the total fixation times for the pretreatment smiling patients were longer for the mouth (1200.49 ms; P <0.05) and shorter for the eyes (249.9 ms; P <0.05). Compared with the posttreatment smiling patients, the total fixation times for the pretreatment smiling patients were longer for the mouth (975.52 ms; P <0.05) and shorter for the eyes (604.11 ms; P <0.05). The total fixation times for the eyes and mouth did not significantly differ between the posttreatment patients and the normal subjects.
The results of the mixed-effects linear regression analysis of the total fixation time of the smiling mouth are presented in Tables I–III . The fixed effects presented in Table I account for the effects of malocclusion. The constant term was the mouth fixation time of the normal occlusion group. Positive coefficients represent greater fixation on the mouths of the pretreatment patients compared with the controls (normal subjects). According to the coefficients, the estimated fixation time of the normal subjects was 1239.624 ms, whereas the estimated fixation time of the pretreatment patients determined by the mixed model was 1239.624 + 1061.344 = 2300.968 ms. In Table II , the fixed effects account for the effects of treatment. The constant term is the mouth fixation time of the normal occlusion group. Therefore, no difference was noted between the mouth fixation time of the normal occlusion and posttreatment groups. In Table III , the fixed effects account for the effects of treatment. The constant term is the mouth fixation time of the pretreatment group. Negative coefficients represent decreased fixation on the mouth compared with the pretreatment group. According to the coefficient, the estimated fixation time of the pretreatment patients was 2319.889 ms, whereas that of the posttreatment patients determined by the mixed model was 2319.889 − 999.598 = 1320.291 ms. The random effects included observer effect and residual effect. The observer effect was the variance within each observer, and the residual effect was the variance in images and the inherent error in the model. The observer and residual effects were considered in this model.
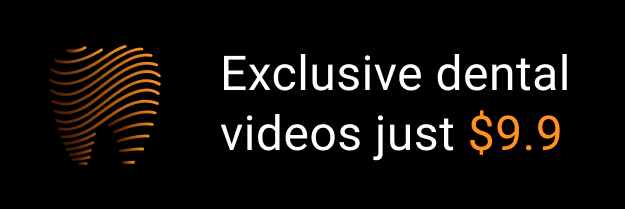