Introduction
Our objectives were to assess reliability, validity, and time efficiency of semiautomatic segmentation using Segura software of the nasal and pharyngeal airways, against manual segmentation with point-based analysis with color mapping.
Methods
Pharyngeal and nasal airways from 10 cone-beam computed tomography image sets were segmented manually and semiautomatically using Segura (University of Alberta, Edmonton, Alberta, Canada). To test intraexaminer and interexaminer reliabilities, semiautomatic segmentation was repeated 3 times by 1 examiner and then by 3 examiners. In addition to volume and surface area, point-based analysis was completed to assess the reconstructed 3-dimensional models from Segura against manual segmentation. The times of both methods of segmentation were also recorded to assess time efficiency.
Results
The reliability and validity of Segura were excellent (intraclass correlation coefficient, >0.9 for volume and surface area). Part analysis showed small differences between the Segura and manually segmented 3-dimensional models (greatest difference did not exceed 4.3 mm). Time of segmentation using Segura was significantly shorter than that for manual segmentation, 49 ± 11.0 vs 109 ± 9.4 minutes ( P <0.001).
Conclusions
Semiautomatic segmentation of the pharyngeal and nasal airways using Segura was found to be reliable, valid, and time efficient. Part analysis with color mapping was the key to explaining differences in upper airway volume and provides meaningful and clinically relevant analysis of 3-dimensional changes.
Highlights
- •
Cone-beam computed tomography is a popular tool for airway analysis.
- •
Accurate reconstruction of the nasal airway and analysis beyond volume are limitations.
- •
A new segmentation algorithm, LEDGES, provided reliable 3D rendering of the airway.
- •
LEDGES reduced segmentation time by 55% compared with manual segmentation.
- •
Analysis of the 3D airways helped explain differences in global measures.
Three-dimensional (3D) models of the upper airway segmented from cone-beam computed tomography (CBCT) images have been used to visualize and analyze treatment efficiency in subjects with snoring and obstructive sleep apnea. Providing accurate modalities for morphofunctional analysis are essential for improving diagnosis, treatment planning, and assessing treatment outcomes.
Commercial dental software products mainly focus on pharyngeal airway segmentation and largely neglect appropriate segmentation of the nasal cavity airway. Commercial software products that segment the nasal cavity apply automatic segmentation of the upper airway with global thresholding. This method relies on setting an intensity range (gray threshold) so that voxels having intensity values outside that range are set to zero. This provides fast but potentially inaccurate segmentation. This means that depending on the gray threshold, values similar to air (eg, thin mucous tissues or secretions) or identical to air (eg, noise), or air surrounding the patient, will be erroneously added in the segmentation and 3D reconstruction. These automated products do not allow the necessary operator control to correct the segmentation or threshold per 2-dimensional slice. Segmentation of the nasal cavity using commercial dental software yielded unacceptable results compared with the standard method of manual segmentation. In the ear, nose, and throat literature, segmentation of the nasal cavity is addressed using different semiautomatic methods; although these were found to be accurate compared with manual segmentation, they were not time efficient (up to 3.5 hours to segment 1 airway).
Moreover, commercial products have not been adequately tested for accuracy. Validating these methods should be completed, ideally, against manual segmentation of the CBCT images of actual airways such as in the study by El and Palomo. Instead, most studies have tended to validate automatic segmentation methods using phantoms constructed into uniform geometries (eg, cylinder) or replicating the pharynx. Since the shape of the pharyngeal airway is similar to a simple hollow tube, studies that focus only on this part of the airway will likely overrepresent the true validity of the evaluated tools. In addition, volumetric measurement was the most popular parameter assessed. This parameter is not specific because it disregards the distribution of the changes in the airway, despite supplementing it with local, linear, or cross-sectional area measurements.
In the scope of sleep-disordered breathing, the nasal cavity is as important as the pharyngeal airway because it houses several findings that could obstruct the air flow before it reaches the pharynx: eg, nasal septum deviations and pneumatized or hypertrophied conchae. Current methods for full segmentation of the upper airway are either time-consuming (manual segmentation) or inaccurate to segment the nose (automated commercial products). Therefore, the purposes of this study were (1) to establish a semiautomatic segmentation technique that is reliable, valid, and time efficient for both the pharyngeal and nasal airways against manual segmentation; and (2) to use measures more meaningful than volume to assess the 3D airway models to reflect their shape characteristics.
Material and methods
This study was approved by the Health Research Ethics Board at the University of Alberta (number Pro00021181). Ten CBCT image sets of adolescents (ages, 11-15 years) were randomly, without age or sex restrictions, and retrospectively selected from the orthodontic graduate clinic database at the University of Alberta. The images were acquired by the Classic i-CAT CBCT scan (Imaging Sciences International, Hatfield, Pa). The CBCT images were acquired for orthodontic purposes where conventional radiography failed to provide sufficient information; eg, orthognathic surgery patients, mandibular asymmetries, or abnormal maxillary transverse growth. The CBCT machine is routinely calibrated, and the subjects used a lead apron. The CBCT protocol used a medium-large field of view (16 cm wide × 13 cm high), 120 kV(p), 24 mA, 20-second scan time, 0.3-mm voxel size, and 303 basis projections.
The upper airway region of interest (ROI) included the oronasopharynx, and the inferior and middle nasal meatuses. It extended from the anterior nasal nares to the level of the anterior-inferior point of the body of the third cervical vertebra. The maxillary sinuses, superior nasal meatus, and ethmoid air cells were not included. Each CBCT image set was segmented manually and semiautomatically, and the times of both methods of segmentation for each CBCT case (n = 10) were recorded in minutes to compare time efficiency.
Using the Mask tool in Mimics software (Mimics version 15.0; Materialise, Leuven, Belgium), the principal investigator (N.A.A.) manually selected the gray-level threshold on each axial slice for the entire ROI (400-430 slices; 0.3-mm slice thickness and interval). The gray threshold of the upper airway represents pixel intensity from i-CAT exposure, measured by Mimics software and ranged from approximately −1000 to −500 depending on the location of the slice and the quality of the scan. The investigator adjusted the mask by erasing or adding to the highlighted airway on each slice. Once the upper airway was defined and edited, a 3D model of the mask was created and saved in an ASCII STL format.
To overcome the difficulties related to the complex morphology of the nasal cavity, gray-level heterogeneity of the airway, and thin bony septae, we proposed a semiautomatic segmentation program called “Segura” developed at University of Alberta and specific to the upper airway. Segura is a custom-written program created using MATLAB (R2012b, MathWorks, Natick, Mass). It implements Localized Threshold Decomposition Gradient Segmentation (LEDGES) that takes into account the gradient edge strength (indicating a clear demarcation between the object and its background) to ultimately select the local gray threshold. This algorithm mitigates the major drawback of any global threshold method: that one threshold value cannot, in general, effectively segment an entire image. This provided excellent reproducibility and accuracy in preliminary testing; details of the algorithm and its testing are provided in the Supplemental Material . Using Segura, the investigator initiated seed points in the most inferior axial slice in the ROI (ie, within the oro/hypopharyngeal airway) and then allowed the seed points to automatically copy and adjust as the slice moved superiorly toward the nasal cavity in the ROI. The investigator would adjust the seed points, seal unwanted sinuses or airway passages, and edit the boundaries of segmentation when needed. Once the upper airway was defined and edited, a 3D model of the segmented ROI was created and saved in an ASCII STL format. Details of Segura are provided in the Supplemental Material .
This method of segmentation was completed 3 times for each CBCT data set by the same investigator, 1 week apart to assess intraexaminer reliability. To test interexaminer reliability, 2 additional examiners (M.A.Q.A., M.O.L.) with experience in CBCT anatomy were familiarized and lightly trained to use Segura with 1 case not included in the study. Both examiners segmented the same 10 upper airway cases, using the same computer.
Three-dimensional analysis was performed between the principal investigator’s 3 trials of semiautomatic segmentation using Segura and between the principal investigator’s second trial and those of the 2 other examiners to assess intraexaminer and interexaminer reliabilities, respectively. To assess validity, 3D analysis was performed between the principal investigator’s second trial using Segura and manual segmentation. The airway analysis was completed as follows.
The 3D airway models (in .STL format) were exported to 3-matic software (version 7.0; Materialise), smoothed by a factor of 0.7, and its surface wrapped. Then, each model pair for comparison was registered using N-point registration followed by global registration in 3-matic. Point registration allowed the principal investigator to manually select several points (n = 10) on the manually segmented model and their matches on the semiautomatic segmented model; the software then registered both models based on these points. Global registration then fine tuned the N-point registration.
The total volume and surface area were measured for each airway model. In addition, a point-based analysis using the “part comparison analysis” tool in 3-matic was applied. This tool, previously used and described by Alsufyani et al, measures the distance (in millimeters) between each triangular node forming the 3D mesh from 1 airway model to the surfaces of the reference airway model. Because of insufficient studies reporting changes in airway measurements when clinical significance was noted, the threshold for “part comparison analysis” was conservatively set at 2 mm. On the color-mapped 3D models, green areas represented distances (differences) between 2 airway models within the threshold boundaries (between 2 and −2 mm) and are expressed as “median part analysis.” Blue areas represented distances less than −2 mm and are expressed as “minimum part analysis.” Red areas represented distances greater than 2 mm and are reported as “Maximum part analysis.” A desirable analysis was more green areas and fewer red and blue areas because these represent large distances or differences between both models.
This comparison was completed between the different models created by intraexaminer and interexaminer semiautomatic segmentation, and then between pairs of semiautomatic and manual segmentation airway models. For all 3 variables—median, minimum, maximum part analyses—descriptive values are reported.
Based on paired t tests for a power of 80% and a significance level of 5%, using the volume means and standard deviations between the manual and automatic segmentations of the upper airway reported by El and Palomo, the sample size average was 14.5 (range, 8-21). Power analysis was recalculated based on the means and standard deviations of the results of our 10 subjects.
Statistical analysis
Intraexaminer and interexaminer reliabilities were tested using intraclass correlation coefficient (ICC) values of volume and surface areas. The mean differences in volume, surface area, and part analyses of the resultant 3D models were assessed with repeated-measures analysis of variance (ANOVA).
To test the validity between the second segmentation of Segura against that of manual segmentation, the ICC values of volume and surface areas were calculated. The differences in volume and surface areas of the resultant 3D models were assessed with paired t tests.
All statistical analyses were completed using SPSS software (version 21.0; IBM, Armonk, NY), and significance levels for the paired t tests and repeated-measures ANOVA were set at P <0.05.
Results
Despite the intent to exclude the superior nasal meatus and ethmoid air cells, there were intraexaminer and interexaminer variabilities in segmenting superior extensions. Thus, a superior “cutting” plane through the middle nasal meatus was created to limit the superior extent of segmentation. This cutting plane was applied postsegmentation on the resultant 3D models of the airways. The superior cutting plane was based on the right and left sphenopalatine foramina posteriorly and a point bisecting the line between the tip of the nasal bone and nasion anteriorly ( Fig 1 ). The reliability of landmark identification of the sphenopalatine foramina and the tip of the nasal bone has been verified previously.
The intraexaminer reliability of Segura segmentation was excellent. The ICC values between the 3 trials of Segura were 0.99 (95% confidence interval [95% CI], 0.97-0.99) for volume and 0.99 (95% CI, 0.97-0.99) for surface area. Using the superior cutting plane reduced the differences in volume and surface area and minimally affected part analysis. The average intraexaminer differences in volume and surface area were 2.4% ± 1.3% and 1.2% ± 0.8%, respectively; in the median part analysis, the difference was 0.2 ± 0.1 mm. Greater part analyses (distances) ranged between 2.4 and 3.9 mm ( Table I ) and were localized ( Fig 2 ). The differences in minimum part analysis were statistically significant between the second and third trials.
Parameter measured | Before superior plane definition | After superior plane definition | ||||||
---|---|---|---|---|---|---|---|---|
Mean ± SD | Minimum | Maximum | P value | Mean ± SD | Minimum | Maximum | P value | |
Average volume difference | ||||||||
(cm 3 ) | 1.2 ± 1.4 | 0.2 | 4.87 | NS ∗ | 0.6 ± 0.3 | 0.2 | 1.1 | NS ∗ |
(%) | 3.6 ± 2.9 | 1 | 9 | 2.4 ± 1.3 | 1 | 4.7 | ||
Average surface area difference | ||||||||
(cm 2 ) | 3.17 ± 2.4 | 0.3 | 8.2 | NS ∗ | 2.6 ± 1.4 | 0.3 | 5 | NS ∗ |
(%) | 1.8 ± 1.4 | 0.2 | 4.8 | 1.2 ± 0.8 | 0.2 | 3.1 | ||
Average mean part analysis (mm) | 0.2 ± 0.1 | 0.0 | 0.4 | NS ∗ | 0.2 ± 0.1 | 0 | 0.4 | NS ∗ |
Average minimum part analysis (mm) | 1.6 ± 0.6 | 0.8 | 2.7 | <0.01 † | 1.6 ± 0.6 | 0.8 | 2.4 | <0.01 † |
Average maximum part analysis (mm) | 3.6 ± 0.6 | 2.5 | 4.3 | NS ∗ | 3.3 ± 0.5 | 2.5 | 3.9 | NS ∗ |
Interexaminer reliability of Segura segmentation was excellent. The ICC values between the 3 examiners using Segura were 0.98 (95% CI, 0.95-0.99) for volume and 0.97 (95% CI, 0.85-0.99) for surface area. Using the superior cutting plane largely reduced the differences in surface area and slightly reduced volume and part analysis. The average differences in volume and surface area were 5.5% ± 3.2% and 2.7% ± 1.1%, respectively, and greater differences were noted in subjects 8, 9, and 10 ( Table II ; Fig 3 ). The average difference in median part analysis was 0.4 ± 0.1 mm. Greater part analysis values ranged between 3.5 and 5.8 mm ( Table II ) and were localized ( Fig 4 ). In terms of segmentation time, examiner 2 (M.O.L.) spent the least time (32.3 ± 11.9 minutes) followed by examiner 1 (N.A.A.) (46.8 ± 6.7 minutes) and examiner 3 (M.A.Q.A.) (53.5 ± 8.3 minutes). The differences in volume, surface area, and minimum part analysis were statistically significant between the second examiner and the remaining 2 examiners.
Parameter measured | Before superior cutting plane | After superior cutting plane | ||||||
---|---|---|---|---|---|---|---|---|
Mean ± SD | Minimum | Maximum | P value ∗ | Mean ± SD | Minimum | Maximum | P value ∗ | |
Average volume difference | ||||||||
(cm 3 ) | 1.8 ± 0.6 | 1 | 3 | <0.05 | 1.4 ± 0.7 | 0.3 | 2.7 | <0.05 |
(%) | 7.4 ± 2.7 | 3.8 | 12.5 | 5.5 ± 3.2 | 1.2 | 11.5 | ||
Average surface area difference | ||||||||
(cm 2 ) | 14.7 ± 4.5 | 7.7 | 20.9 | ≤0.01 | 4.4 ± 1.9 | 1.1 | 8.2 | ≤0.01 † |
(%) | 9.6 ± 2.9 | 4.9 | 12.9 | 2.7 ± 1.1 | 0.7 | 4.6 | ||
Average nedian part analysis (mm) | 0.4 ± 0.1 | 0.0 | 0.6 | NS | 0.4 ± 0.1 | 0.0 | 0.5 | NS |
Average minimum part analysis (mm) | 2.4 ± 0.7 | 1.1 | 3.5 | <0.01 † | 2.2 ± 0.7 | 1.1 | 3.5 | <0.01 † |
Average maximum part analysis (mm) | 4.6 ± 2.6 | 0.6 | 9.8 | NS | 3.5 ± 2.3 | 0.6 | 5.8 | NS |
Time of segmentation process (min) | ||||||||
Examiner 1 | 46.8 ± 6.7 | 40 | 60 | <0.01 | ||||
Examiner 2 | 32.3 ± 11.9 | 18 | 50 | |||||
Examiner 3 | 53.5 ± 8.3 | 38 | 65 |
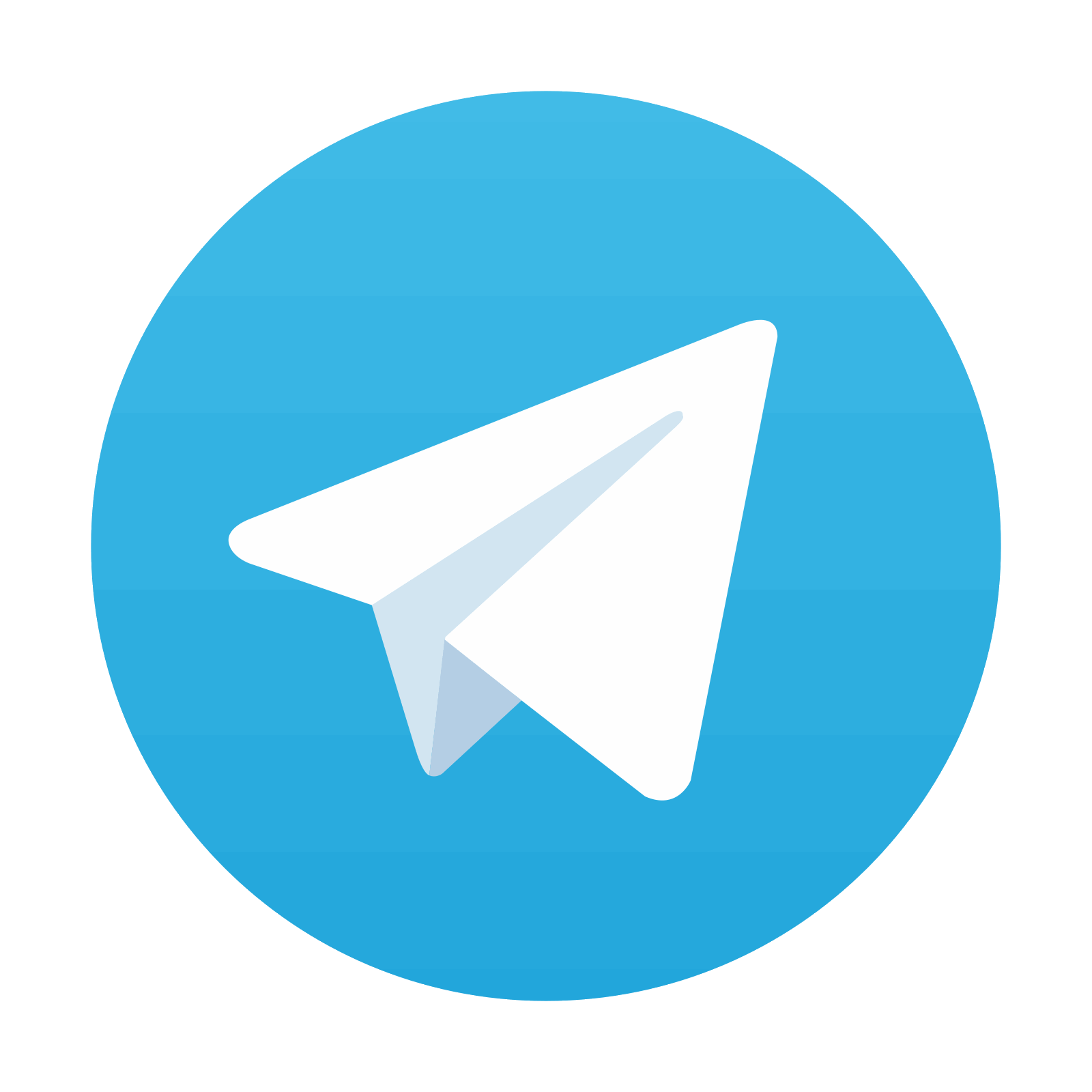
Stay updated, free dental videos. Join our Telegram channel

VIDEdental - Online dental courses
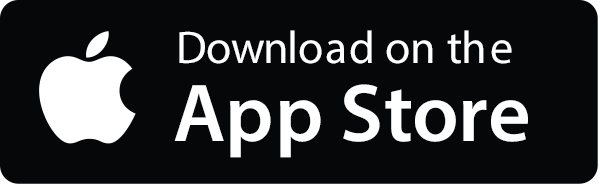

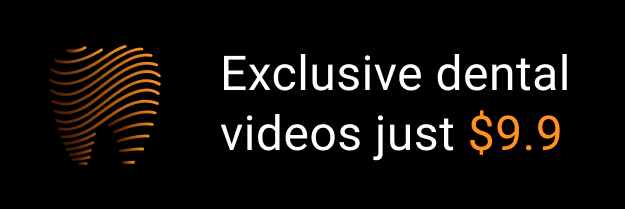