Modeling missing participant outcome data (MOD) in a meta-analysis has been advocated as conceptually and statistically advantageous to methods that manipulate the data before analysis by imputing or excluding MOD. In this column, we introduce the pattern-mixture model for an aggregate binary outcome. We also provide recommendations to handle MOD in meta-analysis properly.
The pattern-mixture model
The pattern-mixture model is applied in each arm of every trial. The ultimate goal is to adjust the probability of an event in the randomized sample for MOD. The pattern-mixture model discerns the randomized participants into those completing the trial arm (called completers) and those leaving prematurely, as well as those experiencing or not the event in each subgroup. We have information on the number of completers and MOD and the outcome of the completers. We do not have information on the outcome of the missing participants. For the latter, we can assume a distribution for the probability of an event. Alternatively, we may prefer a distribution for the odds ratio (OR) of an event between the missing participants and completers. , The assumed distribution comprises the plausible range of values and their likelihood in the probability or OR scale. It reflects our prior belief about the reason(s) for MOD and the uncertainty around this belief. The OR has been preferred for describing the mechanism of MOD better in each trial arm and is known as the informative missingness odds ratio (IMOR).
The informative missingness odds ratio
The IMOR quantifies the departures from the missing at random (MAR) assumption. Being an OR, it takes values from 0 to infinity. An IMOR >1 suggests that missing participants are more likely to experience the event than completers. Conversely, an IMOR <1 implies that completers are more likely to experience the event than missing participants. IMOR equal to 1 corresponds to the MAR assumption, which would imply here that missing participants and completers are equally likely to experience the event.
IMOR is applied in the logarithmic scale and is commonly assumed to follow a normal distribution. , The mean reflects our assumption on average about the MOD mechanism, and the variance implies our uncertainty about this assumption. The MAR assumption refers to a 0 mean because we are on a logarithmic scale. Considering a variance equal to 1 corresponds to a 95% confidence interval for log IMOR that ranges from −1.96 (the odds of an event is 7 times more likely in completers) to 1.96 (the odds of an event is 7 times more likely in missing participants).
Fixing log IMOR to its assumed mean value corresponds to the imputed case analysis (ICA approach). As with the ICA approach, the randomized sample is preserved, but the uncertainty about the MOD mechanism is not accounted for, leading to spuriously precise meta-analysis results. The Table presents the analogy of IMOR to the ICA approach when log IMOR is fixed to its mean value (fixed IMOR).
ICA | Description | IMOR E | IMOR C |
---|---|---|---|
ICA-0 | All missing outcome data are nonevents | 0 | 0 |
ICA-1 | All missing outcome data are events | ∞ | ∞ |
ICA-b | Best-case scenario for the experimental intervention | 0 (or ∞) | ∞ (or 0) |
ICA-w | Worst-case scenario for the experimental intervention | ∞ (or 0) | 0 (or ∞) |
ICA-p C | Same risk as in the control intervention | pC(1−pE)pE(1−pC) p C ( 1 − p E ) p E ( 1 − p C ) |
1 |
ICA-p E | Same risk as in the experimental intervention | 1 | pE(1−pC)pC(1−pE) p E ( 1 − p C ) p C ( 1 − p E ) |
ICA-p | Intervention-specific risk | 1 | 1 |
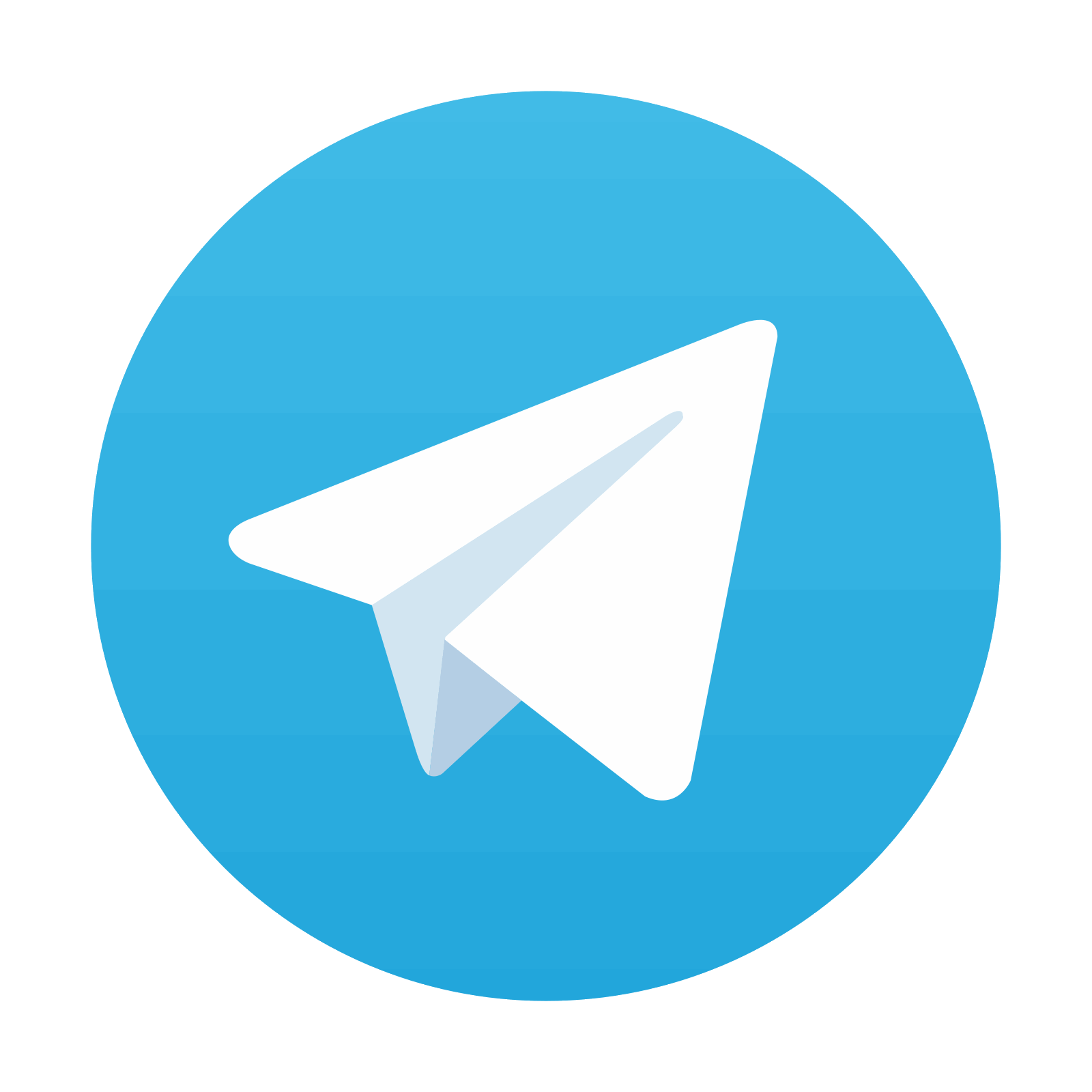
Stay updated, free dental videos. Join our Telegram channel

VIDEdental - Online dental courses
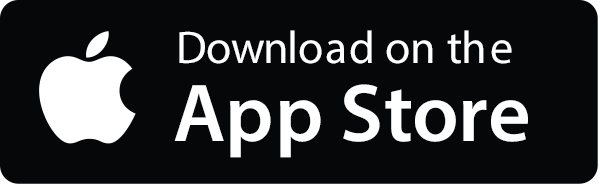

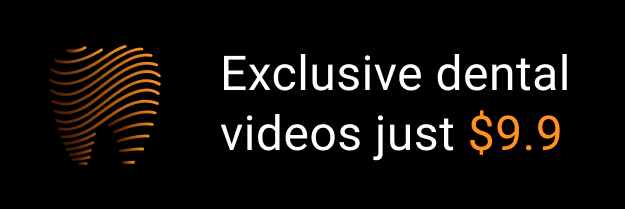