Introduction
In recent years, artificial intelligence (AI) has been applied in various ways in medicine and dentistry. Advancements in AI technology show promising results in the practice of orthodontics. This scoping review aimed to investigate the effectiveness of AI-based models employed in orthodontic landmark detection, diagnosis, and treatment planning.
Methods
A precise search of electronic databases was conducted, including PubMed, Google Scholar, Scopus, and Embase (English publications from January 2010 to July 2020). Quality Assessment and Diagnostic Accuracy Tool 2 (QUADAS-2) was used to assess the quality of the articles included in this review.
Results
After applying inclusion and exclusion criteria, 49 articles were included in the final review. AI technology has achieved state-of-the-art results in various orthodontic applications, including automated landmark detection on lateral cephalograms and photography images, cervical vertebra maturation degree determination, skeletal classification, orthodontic tooth extraction decisions, predicting the need for orthodontic treatment or orthognathic surgery, and facial attractiveness. Most of the AI models used in these applications are based on artificial neural networks.
Conclusions
AI can help orthodontists save time and provide accuracy comparable to the trained dentists in diagnostic assessments and prognostic predictions. These systems aim to boost performance and enhance the quality of care in orthodontics. However, based on current studies, the most promising application was cephalometry landmark detection, skeletal classification, and decision making on tooth extractions.
Highlights
- •
Artificial intelligence (AI) tools can contribute to accurate orthodontics diagnosis.
- •
AI tools can save time.
- •
AI can automatically detect landmarks on the lateral cephalogram.
- •
AI can do skeletal classification and decision making on tooth extraction.
- •
By using AI and precision medicine, orthodontics may evolve this field in the future.
Artificial intelligence (AI) refers to a system’s ability to mimic human-like intelligence or be defined as making effective and right decisions according to a gold standard. The increasing availability of data, computing power, and improvements in analytics methods allow AI to be integrated with many aspects of modern society. Its ubiquitous impacts are already visible in our daily lives, from web searches to content filtering on social media and consumer products like smartphones, cameras, and cars.
One of the main subcategories of AI is machine learning. Machine learning, which needs training data, is a technique to provide predictions of new data and conditions on the basis of the previously learned data’s statistical pattern. This technique allows the computer model to improve over time through experience without classic explicit programming. Deep learning is a subset of machine learning, which requires a model to be fed a large amount of data to learn features about the data with abstractions from multiple processing layers, with the privilege that does not require much engineering efforts to preprocess the data. Deep learning methods have been notably used in visual object recognition and object detection. Being in the age of big data, world governments and companies employed AI and its subfields as one of the leading strategies to deal with complexity in real-world decision making on the basis of large amounts of data.
Medicine is one of the fields that has been enhanced by AI. Specifically, AI’s ability to process a large amount of data reduced the likelihood of neglecting valuable information. It is a potent and reliable tool to help physicians by reducing diagnosis time. , Promising applications of AI diagnostic models have been reported in radiology, dermatology, and oncology studies. These examples include automatic detection of pulmonary nodules, prostate cancer, coronary artery calcification, differentiating skin lesions, lung nodules into benign or malignant, and assessing bone age. ,
AI also has applications in the field of dentistry. Studies suggest that AI can become a powerful decision-making tool within dentistry to promote clinical care. Diagnostic imaging is the most notable use case for the use of AI in dentistry. Currently, applications and research in AI dental radiology focus on the diagnosis of osteoporosis, classification/segmentation of maxillofacial cysts and tumors, , description of periapical disease, cephalometric landmarks detection, etc.
AI has shown to be an effective solution for the diagnosis and evaluation of orthodontic problems. Orthodontic treatments for malocclusion can be categorized as either extraction or nonextraction treatments. This decision is traditionally made on the basis of clinical experience gained over time; therefore, it is hard for new practitioners to make these decisions. Investigations suggest that deep learning methods could help to resolve this problem. Deciding if a patient requires orthognathic surgery can also be challenging for practitioners. AI has shown potential in this field to help clinicians determine whether surgical intervention is necessary. , Cephalometric analysis is extensively used in orthodontics to diagnose facial growth anomalies. The manual localization of cephalometric landmarks on x-ray images is a time-consuming approach with a high error rate. Recent studies demonstrate outstanding achievements in landmark detection by AI methods, especially using deep learning models. , Furthermore, researchers can predict esthetics following orthognathic surgery using AI, which can be a good criterion for operating ( Fig 1 ). Because orthodontics may be unfamiliar with the terms used in the present study, the authors provide a table with terms and definitions ( Supplementary Table I ).

The studies mentioned above have shown promising results regarding the effectiveness of AI to automate decision making in orthodontics. Further investigations must review these methods and evaluate the effectiveness in achieving the mentioned goals in real-world orthodontic scenarios. This study aims to review how different AI models perform in various orthodontic applications: orthodontic diagnosis, treatment planning, and prognosis.
Material and methods
Protocol
The present systematic review follows Preferred Reporting Items for Systematic Reviews and Meta-Analyses (PRISMA) extension for scoping reviews. A filled version of the PRISMA form is provided in Supplementary Table II . This study’s research question was, What are the applications of machine learning techniques and their performances in the field of orthodontics ? The study looked for publications that evaluated the performance of any machine learning or deep learning approaches in the following domains: (1) analysis of orthodontics data, (2) prediction of outcomes in the orthodontics treatments, (3) orthodontic diagnosis, and (4) orthodontics treatment planning.
Eligibility criteria
The following inclusion criteria were used in the selection of the articles:
- 1.
Studies that used machine learning or deep learning algorithms, by definition, a set of algorithms that automatically detect patterns in data improved through experience.
- 2.
Studies that compared the measurement of model outcomes with ground truth or gold standards.
Exclusion criteria were as follows:
- 1.
Studies that used any machine learning or deep learning approaches for problems not directly related to orthodontics (eg, sleep apnea).
- 2.
Studies that did not provide a clear explanation of the machine learning or deep learning model that was used to solve their problem.
- 3.
Review studies were excluded.
Information sources and search
An electronic search was conducted on PubMed, Google Scholar, Scopus, and Embase to find the relevant literature. The search was limited to English publications from January 2010 to June 2020. Various combinations of the following keywords were used in the search procedure: machine learning , deep learning , neural network , artificial intelligence , cephalo∗ , orthodon∗ . The search results are presented in Table I . Endnote X9 (Clarivate, Philadelphia, Pa) was used as a reference manager to manage the search results. Using this tool, duplicate studies were removed. Furthermore, references that were cited within the retrieved papers were reviewed for finding any missing studies. Books, book sections, generics, and thesis were first excluded.
Database | Keywords | No. results |
---|---|---|
Pubmed | ((“machine learning” OR “deep learning” OR “neural network” OR “artificial intelligence”) AND (“cephalo∗” OR “orthodon∗”)) AND ((“2010/01/01”[Date – Publication] : “2020/06/30”[Date – Publication])) | 102 |
Google Scholar | ((“machine learning” OR “deep learning” OR “neural network” OR “artificial intelligence”) AND (“cephalo∗” OR “orthodon∗”)) | 315 |
Scopus | (‘machine learning’:ti,ab,kw OR ‘deep learning’:ti,ab,kw OR ‘neural network’:ti,ab,kw OR ‘artificial intelligence’:ti,ab,kw) AND (‘cephalo∗’:ti,ab,kw OR ‘orthodon∗’:ti,ab,kw) AND [2010-2020]/py | 210 |
Embase | ((TITLE-ABS-KEY (“machine learning”) OR TITLE-ABS-KEY (“deep learning”) OR TITLE-ABS-KEY (“neural network”) OR TITLE-ABS-KEY (“artificial intelligence”)) AND (TITLE-ABS-KEY (“cephalo∗”) OR TITLE-ABS-KEY (“orthodon∗”))) AND PUBYEAR > 2009 | 62 |
Selection of sources of evidence
To identify eligible journal papers and conference proceedings, 2 investigators (H.M.-R. and M.N.) screened the title and abstracts on the basis of inclusion and exclusion criteria independently. Then, the full texts of potentially eligible publications were retrieved for further assessments. Considering the inclusion and exclusion criteria, 2 investigators identified the eligible publications in this stage independently. Any disagreements were resolved through consensus.
Data charting process
The data charting process was conducted by 2 investigators independently. Following the completion of the charting process, any disagreements were discussed and resolved through consensus.
Data items
The following data were extracted for the corresponding groups of studies: the studies’ objective, dataset specifications, data preprocessing procedure, the best-applied machine learning or deep learning model architecture, model measurements, and model performance (on the basis of the best model).
Critical appraisal of individual sources of evidence
For assessing the quality and risk-of-bias (RoB) of the included studies, the QUADAS-2 was used. Using this tool, RoBs were evaluated in 4 domains: patient selection, index tests, reference standard, and flow and timing. Using QUADAS-2, the authors rated concerns regarding the included studies’ applicability in 3 different domains: patient selection, index test, reference standard. There were 3 options in each domain: high , low , and unclear RoB. Because reference standard was the most influencing factor in the relevant studies, the authors considered the reference standard as the primary domain. If it was high or unclear , the RoB of the whole study was set as high or unclear , respectively. If it was low , the RoB of the study was determined on the basis of other domains. If a study had at least 2 high domains or 1 high and 1 unclear , the RoB of the whole study was set as high RoB. Otherwise, if a study had at least 2 unclear domains, the RoB of the entire study was set as unclear . Two investigators completed the evaluation independently. Any disagreements were resolved through consensus.
Synthesis of results
The included studies were divided into 4 categories on the basis of their objective and the application of machine learning: (1) landmark detection in the lateral cephalometry, (2) diagnosis and problem analysis in orthodontics, (3) treatment planning and prognosis, and (4) other studies.
Because there were numerous landmark detection publications in lateral cephalometry, it was considered a separate category. The primary outcomes in all publications were measurable or had predictive outcomes for evaluating the machine learning model. Using a wide range of specific diagnostic tools in each publication, conducting meta-analysis was impossible.
Results
Selection of sources of evidence
A total of 689 studies were retrieved in our initial search in the following databases: PubMed (n = 102), Google Scholar (n = 315), Scopus (n = 210), and Embase (n = 62). After removing the duplicates and title/abstract screening, 61 studies were selected for full-text eligibility assessments. Finally, considering the inclusion and exclusion criteria, 49 studies remained. Moreover, on the basis of the included studies’ references, 3 new publications were added to the selected studies. The PRISMA flow diagram is presented in Figure 2 .

Characteristics of sources of evidence
The publication year of various types of machine learning studies is presented in Figure 3 . As it can be seen, considering that we included studies before July 2020, there is notable growth in the publication of this study from the year 2019.

Critical appraisal within sources of evidence
Results of the RoB assessment of included studies are presented in Supplementary Table III . A total of 77.55% of studies (38/49) were identified as low RoB studies, whereas 14.29% and 8.16% of included studies were identified as studies with high and unclear RoB, respectively.
Results of individual sources of evidence and synthesis of results
Landmark detection in lateral cephalometry
Twenty-one publications were included regarding using machine learning algorithms to detect orthodontic landmarks ( Table II ). Except for Kunz et al, the primary measurable outcomes for evaluating the included studies were at least 1 of the following: successful detection rate in the range of 2-mm (2-mm SDR), mean radial error , and classification accuracy (on the basis of classification accuracy classified per clinical parameter). These measurements are defined as follows :
2−mmSDR=#{j:∥Ld(j)−Lr(j)∥<2}#Ω×100%
where L d and L r are the location of the predicted and referenced landmark, respectively. #Ω is the number of detections made and j ∈ Ω . Furthermore, we defined these as follows:
MRE=∑i=1NRiN
where the radial error ( R ) is <SPAN role=presentation tabIndex=0 id=MathJax-Element-3-Frame class=MathJax style="POSITION: relative" data-mathml='Δx2+Δy2′>Δ?2+Δ?2‾‾‾‾‾‾‾‾‾‾‾√Δx2+Δy2
Δ x 2 + Δ y 2
, and Δx and Δy are the absolute distances in the x-direction and y-direction between the predicted and referenced landmarks, respectively. N is the number of landmarks. Finally, we defined these as follows:
ClassificationAccuracy=TP+TN#AllSamples
where TP and TN are the number of true positive and true negative samples, respectively.
Performance measurements | ||||||||
---|---|---|---|---|---|---|---|---|
Author (y) | Dataset source | Dataset size | No. landmarks | Data preprocessing | Best model architecture | 2-mm SDR | MRE | Classification accuracy |
Oh et al (2020) | ISBI 2015 grand challenge in dental x-ray image | 400 cephalograms | 19 | Data augmentation (consists of geometry and intensity transforms) | A deep anatomic context feature learning framework that enforces the CNN | 86.20% (test 1) 75.89% (test 2) |
11.77 ± 10.13 pixels (test 1) 14.55 ± 8.22 pixels (test 2) |
– |
Song et al (2020) | (A) ISBI 2015 grand challenge in dental x-ray image (B) A dataset provided by Shandong University |
(A) 400 cephalograms (B) 100 images (only for test) |
19 | Extracted patches for each landmark (400 patches for each landmark on each image, totally 60,000) | Transfer learning using pretrained ResNet-50 | 86.4% (dataset A, test 1) 74.0% (dataset A, test 2) 62.0% (dataset B) |
1.077 mm (dataset A, test 1) 1.542 mm (dataset A, test 2) 2.1 mm (dataset B) |
– |
Kunz et al (2020) | A private orthodontic dental practice | 1792 cephalograms (postaugmentation) | 18 | Data augmentation (rotation, tilting, parallel shifting, mirroring, noise adding as well as changes in brightness and contrast) | Customized CNN | Pearson product–moment correlation coefficients: r > 0.864 with P < 0.001. Absolute mean differences: >0.37° for angular parameters, >0.20 mm for metric parameters and >0.25% for the proportional parameter. There were no significant differences between the gold standard and the predictions. (except for SN-MeGo) |
||
Kim et al (2020) | (A) Obtained from 2 medical institutions, (B) ISBI 2015 grand challenge in dental x-ray image |
(A) 2075 cephalograms (B) 400 cephalograms |
23 | Cropped to a width-height ratio of 1.0; region of interest images of each landmark with the original resolution were extracted | 2-stage DNN using a stacked hourglass network | 82.92% (dataset A) 84.53% (dataset B) |
1.37 mm (dataset A) 1.16 mm (dataset B) |
91.17% (dataset A) 83.13% (dataset B) |
Hwang et al (2020) | Not mentioned | 1311 cephalograms | 80 | – | YOLOv3 algorithm with custom modifications | – | 1.46 ± 2.97 mm (compared with human 1.50 ± 1.48 mm) | – |
Gilmour and Ray (2020) | ISBI 2015 grand challenge in dental x-ray image | 400 cephalograms | 19 | Extracted patches for each landmark | Transfer learning using pretrained ResNet-50 with foveated pyramid attention algorithm | 88.32% (test 1) 77.05% (test 2) |
1.01 ± 0.85 mm (test 1), 1.33 ± 0.74 mm (test 2) |
– |
Zhong et al (2019) | ISBI 2015 grand challenge in dental x-ray image | 300 cephalograms (Test 2 was excluded) | 19 | Cropped to 1935 × 1935 pixels. Scaled image by 0.15 times | An attention-guided deep regression model through 2 stage U-net (using the expansive exploration) | 86.74% | 1.14 ± 1.03 mm | – |
Song et al (2019) | ISBI 2015 grand challenge in dental x-ray image | 400 cephalograms | 19 | Extracted patches for each landmark (200 patches for each landmark) | Transfer learning using pretrained ResNet-50 | 85.0% (test 1), 81.8% (test 2) |
1.147 mm (test 1), 1.223 mm (test 2) |
– |
Qian et al (2019) | ISBI 2015 grand challenge in dental x-ray image | 400 cephalograms | 19 | Data augmentation using multiscaling strategy (narrow image size from 0.3 to 0.9), produced more than 1000 cephalograms | An improved faster R-CNN (using multitask loss) | 82.5% (test 1), 72.4% (test 2) |
– | – |
Park et al (2019) | Obtained from Seoul National University Dental Hospital | 1311 cephalograms | 80 | – | YOLOv3 and single shot multibox detector algorithm with custom modifications | 80.4% | – | – |
Nishimoto et al (2019) | Scraping cephalograms on the internet | 219 cephalograms | 10 | Data augmentation by rotating, deviating, and changing contrast produced more than 7803 cephalograms | Customized CNN | – | 17.02 ± 11.13 pixels | – |
Goutham et al (2019) | ISBI 2015 grand challenge in dental x-ray image | 400 cephalograms | 7 | A total of 6750 segmentation map for each landmark point, adaptive histogram equalization | Modified u-net | 65.13% | – | – |
Dai et al (2019) | ISBI 2014 grand challenge in dental x-ray image | 300 cephalograms | 19 | Cropping with template matching | Adversarial encoder-decoder networks | Between 35% and 40% for each landmark | 2.5-7.5 mm for each landmark | – |
Chen et al (2019) | (A) ISBI 2015 grand challenge in dental x-ray image (B) 5 new datasets collected (A-E) for the purpose of the study |
(A) 400 cephalograms, (B) 1857 cephalograms |
19 | – | End-to-end deep learning with novel attentive feature pyramid usion module using Inception | 86.21% (test 1) and 73.89% (test 2), Best result on other data sets: 94.73% |
1.25 mm (test 1) and 1.47 mm (test 2).Best result on other datasets: 0.88 mm | |
Wang et al (2018) | (A) ISBI 2015 grand challenge in dental x-ray image (B) Dataset provided by Peking University School and Hospital of Stomatology (private) |
(A) 300 cephalograms (Test 2 was excluded), (B) 165 cephalograms |
(A) 19 (B) 45 |
SIFT-based patch feature extraction | Multiresolution decision tree regression voting | (A) 73.37% (B) 72.08% |
(A) 1.69 ± 1.43 mm (B) 1.71 ± 1.39 mm |
|
Arık, S., et al. (2017) | ISBI 2014, 2015 grand challenge in dental x-ray image | 400 cephalograms | 19 | Down sampled by 3 (by taking the average of each 3 × 3 patch) for dimensionality reduction | Customized CNN | 75.58% (2014), 75.37% (2015-test 1), and 67.68% (2015-test 2) | – | 75.92% (2015-test 1) and 76.75% (2015-test 2) |
Lindner, C. et al (2016) | ISBI 2015 grand challenge in dental x-ray image | 400 cephalograms | 19 | – | RF regression-voting using constrained local model framework | 84.7% | 1.2 mm | 78.4% (over all classes), 83.4% (over all subjects) |
Lindner and Cootes (2014) | ISBI 2015 grand challenge in dental x-ray image | 400 cephalograms | 19 | – | RF regression-voting using constrained local model framework | 74.95% | 1.67 ± 1.65 mm | 77% |
Chu et al (2014) | ISBI 2014 grand challenge in dental x-ray image | 200 cephalograms | 19 | – | Combination of RF regression-based landmark detection with sparse shape composition model based landmark correction | 77.79% (4mm-SDR) | – | – |
Vandaele et al (2015) | ISBI 2014 grand challenge in dental x-ray image | 200 cephalograms | 19 | Downsizing into 6 different resolutions | Ensembles of extremely randomized trees combined with simple pixel-based multiresolution features | 77.58% | 1.83 ± 1.81 mm | – |
Mirzaalian, H., et al (2014) | ISBI 2014 Grand Challenge grand challenge in dental x-ray image | 200 cephalograms | 19 | – | Random decision forest-based likelihoods | 65.26 ± 18.26 % | About 2 mm | – |
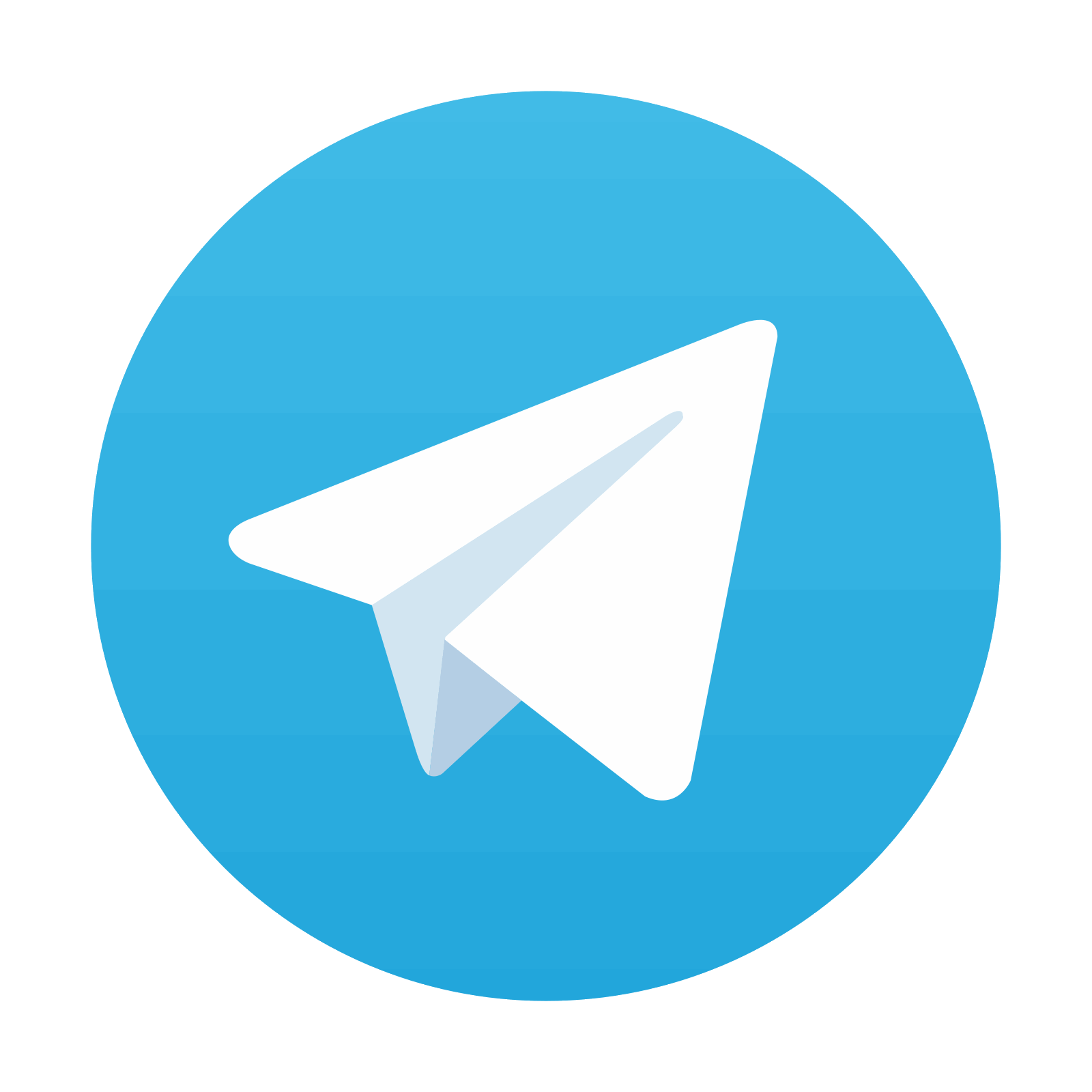
Stay updated, free dental videos. Join our Telegram channel

VIDEdental - Online dental courses
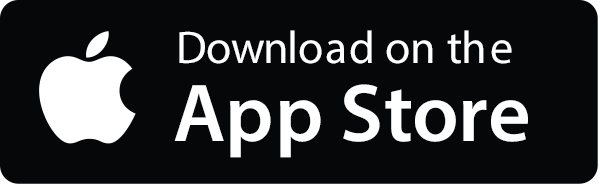

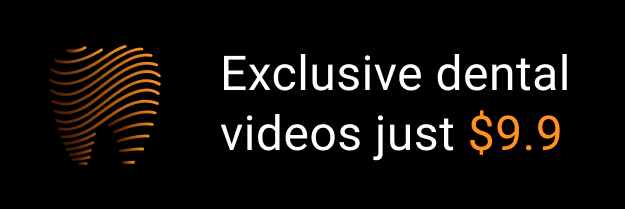