The primary interest of a systematic review is to estimate the summary effect size from a sample of studies and to infer the impact of the compared interventions on a specific health condition. In a previous article, we discussed the statistical heterogeneity between the included studies in the meta-analysis. Investigating heterogeneity is an important step in providing a more accurate interpretation of the results. The observed between-study heterogeneity in a meta-analysis can be the result of differences in the characteristics of the included studies, such as year of publication, dose, duration of applied intervention, or timing of outcome data collection. Common methods to explore heterogeneity on the basis of important study characteristics include subgroup analysis and meta-regression. In this article, we introduce the subgroup analysis, and we also present an index that quantifies the proportion of heterogeneity explained by the covariate of interest.
Subgroup analysis: definition, aims, and application
Is the intervention more effective if given for longer periods of time? Are high-risk populations less likely to benefit from the intervention? Do studies with a small sample size tend to provide larger effect sizes? Is a larger effect size associated with inadequate randomization? These questions emphasize the importance of examining whether the effect size varies according to specific study characteristics to possibly explain the heterogeneity of the results across the included studies. This variation is known as effect modification or interaction. The study characteristics are known as covariates, and they can refer to the design and conduct of the studies (eg, duration of follow-up and blinding status) or to various clinical characteristics (eg, sex and average participant age). The choice of these characteristics should be specified a priori in the protocol of a review to prevent data-driven analyses and possibly selective reporting of only interesting results such as those that are statistically significant. Subgroup analysis and meta-regression are the tools to explore possible effect modifications.
The general definition of subgroup analysis is the split of the data into subgroups based on the a-priori determined covariate of interest (ie, sex) and comparisons made between those subgroups. In terms of meta-analysis, subgroup analysis is the split of the included studies into subgroups defined by a categorical covariate (eg, blinding status and geographic setting) and the comparison of the study results between these subgroups of studies. For instance, some studies in a meta-analysis were conducted in the United States and others in Europe. Therefore, we could split the studies into 2 subgroups according to their geographic settings, apply a meta-analysis model separately in each subgroup (ie, we estimate the summary effect size and its variance in each subgroup), and then compare these 2 summary effect sizes using an appropriate test that would show if there is a difference in the estimate between studies conducted in the United States and Europe.
In previous articles, we introduced 2 meta-analysis models—the fixed-effect and random-effects models , —and we discussed the differences in their assumptions and applications. These models apply also to subgroup analysis and meta-regression. Consider a meta-analysis of 10 studies that compares 2 treatments. In 6 studies, the follow-up duration was 7 months (subgroup A), and in 4 studies, it was 9 months (subgroup B). Under the fixed-effect model, we assume that all studies in subgroup A share a common effect size, and all studies in subgroup B share another common effect size. Therefore, under this model, the studies are assumed to be identical in all respects (eg, all studies were performed by the same researchers using the same methods). However, these conditions are rarely met in systematic reviews; hence, the fixed-effect model is less likely to hold in practice.
By contrast, under the random-effects model, the true effect size is assumed to vary across the studies in subgroup A and subgroup B. In this model, we allow some variation of the true effects across the studies of both subgroups. Within either subgroup, it is possible to observe differences from study to study in various study characteristics, and these differences might have an impact on the effect size. Therefore, the random-effects model is more plausible in practice than the fixed-effect model. In this article, we focus on the subgroup analysis using the random-effects model.
Comparing the subgroups
There are 3 computational methods for comparing the summary effect sizes of the subgroups : the Z-test, the Q-test for heterogeneity, and the Q-test based on 1-way analysis of variance (ANOVA). These approaches are mathematically equivalent, and they provide the same P value if, and only if, we compare the summary effect sizes of only 2 subgroups. In this article, we will discuss the latter, which is the simplest approach to apply, and it is also implemented from RevMan software (version 5.3; The Cochrane Collaboration, London, UK). Description of the other 2 methods can be found in the book by Borenstein et al.
Q-test based on 1-way ANOVA: comparing more than 2 subgroups
When we compare more than 2 subgroups, we use 1-way ANOVA. In the simplest case of 1 study, the idea of the ANOVA lies in the partition of the total variance (of all subjects about the grand mean) into the within-group variance (of the subjects about the mean of their group) and the between-group variance (of the group means about the grand mean). Then, we use these variance components to test the null hypothesis that the effect sizes are the same in all groups against the alternative hypothesis that at least 1 group has a different effect size. The same idea holds in a meta-analysis, only that the means are based on studies rather than subjects. The detailed steps to perform this approach are provided in Supplementary Appendix 1 .
Estimating heterogeneity τ 2 in subgroup analysis: to pool or not to pool?
In the random-effects meta-analysis, τ 2 reflects the variation of the true effects across all studies. In the subgroup analysis, we split the studies into subgroups defined by a covariate. In this case, we are interested in learning the dispersion of the true effect sizes within each subgroup. Therefore, we estimate the heterogeneity within each subgroup. There are 2 possible scenarios; these (subgroup-specific) heterogeneities can be either similar or different. Similar heterogeneities imply that the effect sizes have the same dispersion in both groups, whereas different heterogeneities imply that the effect sizes are distributed in a wider or narrower range from 1 subgroup to another. If the heterogeneities are similar, we can pool these values to yield a common estimate for the heterogeneity and then apply this estimate to all subgroups. By contrast, if these heterogeneities are substantially different, then we should not pool these values. However, if most of the subgroups have only a few studies (for instance, 5 or fewer studies), then it is advised to pool these heterogeneities to obtain an accurate estimate. Figure 1 addresses the dilemma to pool or not to pool the heterogeneities across the subgroups. The formula to pool subgroup-specific heterogeneities can be found in Supplementary Appendix 1 .

The R 2 index: an intuitive measure of explained heterogeneity
The comparison of the subgroups does not inform about the amount of heterogeneity explained by the covariate of interest. To achieve this, we use an index that quantifies the proportion of explained heterogeneity by that covariate. This index is known as the R 2 index, and it is defined as a ratio of the explained heterogeneity to the total heterogeneity :
R2=Texplained2Ttotal2
or equivalently
R2=1−Tunexplained2Ttotal2
where <SPAN role=presentation tabIndex=0 id=MathJax-Element-3-Frame class=MathJax style="POSITION: relative" data-mathml='Tunexplained2′>?2???????????Tunexplained2
T u n e x p l a i n e d 2
includes the pooled heterogeneities of the subgroups and <SPAN role=presentation tabIndex=0 id=MathJax-Element-4-Frame class=MathJax style="POSITION: relative" data-mathml='Ttotal2′>?2?????Ttotal2
T t o t a l 2
is the heterogeneity that we obtain from a conventional random-effects meta-analysis model without splitting the included studies into subgroups. This index takes values in the range of 0-1 (or in the range of 0%-100%, expressed as percentage). However, it will never be equal to 1 (or 100%) because the variance within each subgroup is inherently nonzero; hence, some unexplained heterogeneity will always be present. Please note that although this index ranges from 0 to 1 in the population, it may give results outside this range because of sampling error. In this case, we set the value to 0 (0%) or 1 (100%).
In the next article of this series, we use a real example to illustrate the Q-test based on 1-way ANOVA and the calculation of the R 2 index.
Supplementary Appendix 1
Performing Q-test based on 1-way ANOVA
In this approach, we first compute the variance in each subgroup. For the subgroup j , the formula is given by
Qj∗=∑i=1kjwij(Yij−Mj)2=∑i=1kj(Yij−Mjvij)2
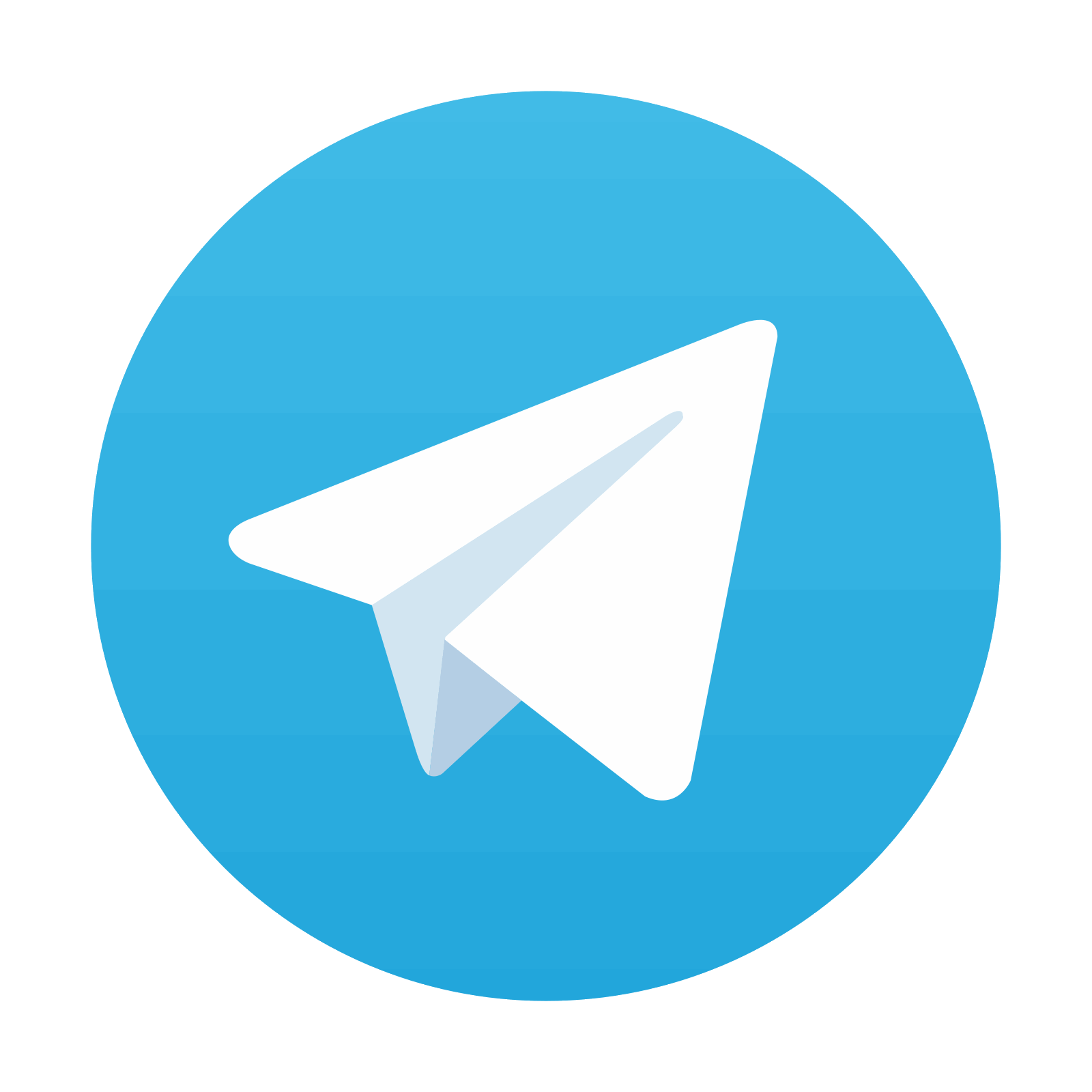
Stay updated, free dental videos. Join our Telegram channel

VIDEdental - Online dental courses
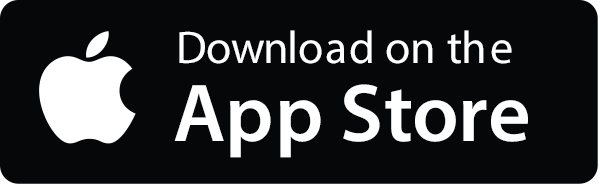

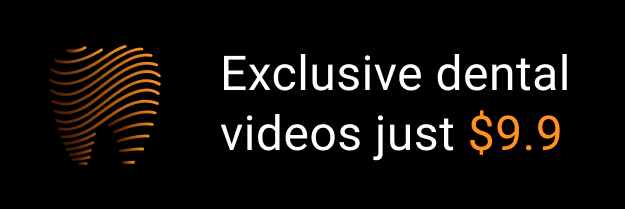