Abstract
Since the introduction of three-dimensional (3D) orthognathic planning software, studies have reported on their predictive ability. The aim of this study was to highlight the limitations of the current methods of analysis. The predicted 3D soft tissue image was compared to the postoperative soft tissue. For the full face, the maximum and 95th and 90th percentiles, the percentage of 3D mesh points ≤2 mm, and the root mean square (RMS) error, were calculated. For specific anatomical regions, the percentage of 3D mesh points ≤2 mm and the distance between the two meshes at 10 landmarks were determined. For the 95th and 90th percentiles, the maximum difference ranged from 7.7 mm to 2.2 mm and from 3.7 mm to 1.5 mm, respectively. The absolute mean distance ranged from 0.98 mm to 0.56 mm and from 0.91 mm to 0.50 mm, respectively. The percentage of mesh with ≤2 mm for the full face was 94.4–85.2% and 100–31.3% for anatomical regions. The RMS error ranged from 2.49 mm to 0.94 mm. The majority of mean linear distances between the surfaces were ≤0.8 mm, but increased for the mean absolute distance. At present the use of specific anatomical regions is more clinically meaningful than the full face. It is crucial to understand these and adopt a protocol for conducting such studies.
Since the introduction of three-dimensional (3D) imaging in orthognathic planning, there have been numerous studies reporting on the accuracy of facial soft tissue prediction of the various software systems. Determining the soft tissue accuracy of any 3D planning system is important, as it forms the basis of the surgical plan and is the only visual aid available to show the patient their final predicted facial soft tissue appearance in 3D.
Assessing the accuracy of two-dimensional (2D) profile prediction programmes is relatively simple and involves importing a template, constructed by superimposing the pre- and postoperative lateral cephalograms on the anterior cranial base, into the planning software. The preoperative hard tissue can then be moved to the postoperative position, based on the template, and a predicted soft tissue profile generated. The difference in specific anatomical landmark position, in the vertical and horizontal directions, between the prediction and the postoperative soft tissue, is a measure of the accuracy.
For analysis of 3D images, volumetric data – computed tomography (CT) or cone beam CT (CBCT) – are generally converted into surface data prior to analysis; this is unnecessary for laser and stereophotogrammetry, as these techniques directly capture the air/soft tissue surface. These surface data can simply be thought of as hundreds if not thousands of 3D landmarks or points in space, joined together to form a ‘polygonal surface mesh’ or ‘triangular surface mesh’. Assessing the accuracy of 3D surgical predictions compared to the actual postoperative result relies on similar techniques of superimposition to 2D, but the method of measurement is potentially more complex. To date, several methods of analysis have been reported including: (1) differences in distance of specific landmarks, (2) differences between all the 3D points of the two entire facial surface meshes, and (3) differences between all the 3D points of the two facial surface meshes following division into predefined anatomical regions. Quantitative analysis of each technique involves measuring the linear distances between specific landmarks or between all of the 3D points of the two 3D surface meshes. This can be performed taking into account the direction using the average distance difference, i.e. the signed difference, or irrespective of direction using the absolute Euclidean difference, and finally the root mean square (RMS) difference. The signed differences will cancel out positive and negative values, under-estimating the error; the absolute Euclidean difference will ignore the direction and only report the magnitude; and the RMS error will give disproportionate weight to very large differences. To reduce the effect of outlying points, the absolute distances between the numerous 3D points of the two surface meshes can be ordered in decreasing magnitude and the data within the 90th lower percentile can be averaged to produce the mean for the 90th percentile. The mean for the 90th percentile can also be calculated using the same method. Other studies have reported the percentage of 3D points where the distance is 2 mm or less between the prediction and actual soft tissue surface meshes.
The aim of this study was to use the methods of analysis described previously to measure the accuracy of 3D surgical predictions, using 10 patients as worked examples, to highlight the limitations of each analysis. It is important as clinicians to have a basic level of understanding in order to assess the accuracy of future 3D orthognathic planning programmes.
Materials and methods
Patient selection
The data for this study were collected, anonymized, and released with the approval of the necessary authorities, and local ethics approval was obtained. The data for the worked examples is based on 10 patients who received only a Le Fort I osteotomy with minimal vertical movements and no associated mandibular surgery. As part of the normal orthognathic surgical treatment protocol, all patients had presurgical CBCT scans taken immediately prior to surgery (T 1 ) and a post-surgical CBCT taken at least 6 months after surgery (T 2 ).
Image preparation
The CBCT scans (DICOM) at T 1 and T 2 were imported into 3dMDvultus 2.2.0 (3dMD, Atlanta, GA, USA) and converted into surface images. Using the automated threshold values, the soft tissue and hard tissue were individually segmented and saved in STL (Standard Tessellation Language) format.
Using VRMesh CAD/CAM mesh editing software (VirtualGrid, Seattle City, WA, USA) installed on a personal computer (Dell XPS Intel quad-core processor), the pre- and postoperative hard tissue STL files for each patient were imported and aligned on the base of the skull. The preoperative image remained static whilst the postoperative image was moved to the aligned position. These two images were merged and exported as a single STL file. This provided a template that could be imported into 3dMDvultus to guide the actual hard tissue movements needed to generate the soft tissue prediction.
Virtual planning
The template (as above) was imported into 3dMDvultus and the preoperative CBCT image loaded. Since both the preoperative CBCT and STL files were the same, both images automatically aligned on one another. Virtual osteotomy cuts were made on the preoperative maxillary and mandibular model and each moved to their respective postoperative positions, guided by the template. The soft tissue prediction and underlying hard tissue were saved as STL files for each patient.
Analysis
The postoperative hard tissue and soft tissue STL files were imported into VRMesh and then ‘grouped’ together to maintain their relationship relative to one another; the same was carried out for the prediction. The two hard tissue images were then aligned on the base of the skull; since both hard and soft tissues were grouped they moved together. If the prediction was perfect, when the hard tissues were aligned, the soft tissues would also be perfectly aligned. Each set of complete images was trimmed to a standardised shape removing any hair, ears, and neck; the soft tissue images were separately exported as VRML (Virtual Reality Modeling Language) files for analysis. Each soft tissue image was then further divided into anatomical regions as shown in Table 1 ; each region was saved as a VRML file.
Anatomical region | Definition of region | Description of soft tissue area |
---|---|---|
Nose | Bridge of nose | Nasion–maxillofrontale (R)–subnasale–nostril base point (R)–nostril base point (L)–maxillofrontale (L) |
Right nare | Right nostril | Nostril base point (R)–alar curvature point (R) |
Left nare | Left nostril | Nostril base point (L)–alar curvature point (L) |
Upper lip | Upper lip | Subnasale–cheilion (R)–cheilion (L)–stomion |
Lower lip | Lower lip | Cheilion (R)–cheilion (L)–stomion–sublabiale |
Chin | Chin | Sublabiale–cheilion (R) perpendicular at level of pogonion–cheilion (L) perpendicular at level of pogonion–gnathion |
Right paranasal | Right cheek | Endocanthion (R)–cheilion (R)–gonion (R) |
Left paranasal | Left cheek | Endocanthion (L)–cheilion (L)–gonion (L) |
Surface mesh analysis
The full face VRML postoperative and prediction images for each patient were imported into an in-house developed software package. The maximum and the mean 95th and 90th percentiles were measured as discussed previously. The percentage of 3D points that were 2 mm or less between the postoperative and prediction facial surface meshes were also calculated, together with the RMS error.
For each predefined anatomical region, the percentage of 3D mesh points 2 mm or below between the postoperative and prediction facial surface meshes were measured.
Landmark analysis
For each patient, the postoperative and prediction facial surface meshes were imported into VRMesh and 10 landmarks were chosen ( Table 2 ). As each landmark was placed, the software automatically indicated the value of the distance between the two surface meshes at that point.
Landmark | Definition |
---|---|
Alar a | The most lateral point on each alar curvature |
Subnasale | The midpoint of the angle at the columella base where the lower border of the nasal septum and surface of the upper lip meet |
Cheilion a | The point located at each labial commissure |
Philtrum | Midline of upper lip at cupid’s bow |
Stomion | The lowest midline point on the vermillion of the upper lip |
Soft tissue B point | The deepest midline point on the labiomental fold, which determines the lower border of the lower lip or the upper border of the chin |
Labrale inferius | The midpoint of the mucocutaneous border of the lower lip |
Pogonion | The most anterior midpoint of the chin, located on the skin surface in front of the identical bony landmark of the mandible |
Results
Table 3 shows the maximum distance, the distances between the predicted and actual complete facial surface meshes for the 95th and 90th percentiles, and standard deviation for each of the 10 patients. Also shown is the percentage of 3D points where the distance is less than 2 mm, as well as the RMS error. Using the 95th and 90th percentiles, the maximum difference ranged from 7.7 mm (patient 7) to 2.2 mm (patients 2 and 6) and from 3.7 mm (patient 7) to 1.5 mm (patient 6), respectively. The mean difference of the absolute distance ranged from 0.98 mm (patient 1) to 0.56 mm (patient 6) and from 0.91 mm (patient 1) to 0.50 mm (patient 6) for the 95th and 90th percentiles, respectively. When considering the percentage of points with less than 2 mm error, this ranged from 94.4% (patient 6) to 85.2% (patient 7). The RMS error ranged from 2.49 mm (patient 7) to 0.94 mm (patient 3).
Patient No. | 90th percentile | 95th percentile | 100% mesh points | |||||
---|---|---|---|---|---|---|---|---|
Max (mm) | Mean (mm) | SD (mm) | Max (mm) | Mean (mm) | SD (mm) | % mesh below 2 mm | RMS error (mm) | |
1 | 2.1 | 0.91 | 0.55 | 2.8 | 0.98 | 0.63 | 89.0 | 1.66 |
2 | 1.7 | 0.53 | 0.53 | 2.2 | 0.59 | 0.62 | 93.8 | 0.95 |
3 | 1.9 | 0.56 | 0.46 | 2.5 | 0.63 | 0.55 | 90.3 | 0.94 |
4 | 1.7 | 0.52 | 0.41 | 2.4 | 0.59 | 0.52 | 93.0 | 1.11 |
5 | 2.2 | 0.63 | 0.54 | 2.9 | 0.70 | 0.65 | 88.8 | 1.50 |
6 | 1.5 | 0.50 | 0.43 | 2.2 | 0.56 | 0.52 | 94.4 | 1.15 |
7 | 3.7 | 0.59 | 0.66 | 7.7 | 0.87 | 1.33 | 85.2 | 2.49 |
8 | 2.4 | 0.69 | 0.68 | 3.6 | 0.82 | 0.86 | 87.9 | 2.41 |
9 | 2.2 | 0.71 | 0.54 | 3.0 | 0.79 | 0.66 | 88.1 | 1.40 |
10 | 2.3 | 0.61 | 0.55 | 3.4 | 0.72 | 0.71 | 87.7 | 1.46 |
The percentage of 3D points with less than 2 mm error when the face is divided into anatomical regions is shown in Table 4 . For the chin, 100% to 90% of the points were less than 2 mm, for the left nare 97.9% to 55.5%, left paranasal 99.9% to 70.4%, lower lip 100% to 31.3%, nose 98.1% to 78.6%, right nare 99.4% to 67.3%, right paranasal 98.2% to 55.8%, and upper lip 99.4% to 47.3%.
Patient No. | Chin | Left nare | Left paranasal | Lower lip | Nose | Right nare | Right paranasal | Upper lip |
---|---|---|---|---|---|---|---|---|
1 | 90 | 90.8 | 72.5 | 50.0 | 86.3 | 83.5 | 75.6 | 47.3 |
2 | 100 | 93.3 | 96.9 | 74.3 | 78.6 | 92.8 | 93.4 | 99.4 |
3 | 100 | 79.7 | 86.8 | 100 | 95.8 | 76.4 | 55.8 | 99.1 |
4 | 100 | 95.8 | 99.9 | 100 | 93.3 | 87.2 | 87.1 | 89.2 |
5 | 100 | 92.4 | 88.1 | 99.8 | 81.1 | 84.7 | 85.5 | 87.0 |
6 | 99.8 | 97.9 | 97.8 | 76.1 | 98.1 | 99.4 | 98.3 | 87.9 |
7 | 98.8 | 89.4 | 85.9 | 99.8 | 90.6 | 88.6 | 92.5 | 79.6 |
8 | 94.5 | 55.0 | 96.9 | 31.3 | 85.5 | 97.6 | 94.4 | 84.3 |
9 | 100 | 95.3 | 70.4 | 68.1 | 85.9 | 90.3 | 79.7 | 79.8 |
10 | 99.9 | 97.5 | 87.3 | 79.7 | 78.7 | 67.3 | 92.9 | 73.6 |
Mean | 98.3 | 88.7 | 88.3 | 77.9 | 87.4 | 86.8 | 85.6 | 82.7 |
SD | 3.4 | 13.0 | 10.3 | 23.6 | 6.9 | 9.6 | 12.6 | 14.9 |
95% CI | ||||||||
Lower | 95.6 | 79.4 | 80.9 | 61.0 | 82.5 | 79.9 | 76.5 | 72.1 |
Upper | 100.0 | 98.0 | 95.6 | 94.8 | 92.3 | 93.7 | 94.5 | 93.4 |
Table 5 shows the mean differences between the prediction and actual soft tissue change following superimposition of the two images and measuring the difference at 10 specific landmarks for each patient. All of the measurements except right alar and subnasale were 0.8 mm or below. Right alar and subnasale were 1.1 ± 1.0 mm and −1.7 ± 1.5 mm, respectively. The negative sign indicates that the predicted image lies behind the actual soft tissue image. The root mean square difference for all the measurements was 1.3 mm.
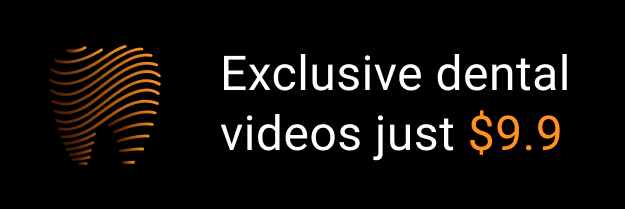