It has been reported that periodontitis increases the risk of cardiometabolic diseases. It also has been proven that smoking or obesity increases periodontitis and that they independently contribute to cardiometabolic diseases. Thus, smoking or obesity becomes a confounding factor for periodontitis. Therefore, if we wish to establish the unbiased relationship between periodontitis and cardiometabolic diseases, the effects of smoking or obesity that coincides with periodontitis must be controlled
In a complex biological system such as human physiology, the permutations of confounding factors can determine health versus disease state and usually are enumerated with large individual variations. Therefore, while difficult to achieve absoluteness, there are means to reduce or eliminate some of their impacts as illustrated in Fig. 8.1 as well as use of cross-correlation approaches to optimize the final results.
Although we previously assumed that innate immune system is activated by invading pathogens only, as our knowledge expands, we now know that obesity and diabetes endogenously activate innate immunity and generate low-grade inflammation [61, 62]. Pischon et al. reported that periodontal treatment resulted in decreased e-selectin levels. Unfortunately, this study did not provide pretreatment characteristics of the cohort. We have no way of knowing whether metabolic inflammation could have biased the results. Although it was a “self as control” study design, metabolic inflammation would have altered the serum inflammatory markers. Thus, it is important to adjust some measure of metabolic inflammation [63].
In recent years, the gut microbiome was publicized as “a new organ” causing obesity [53]. Diet will provide substrate for gut microbiome and will alter the intestinal microbial composition. Indeed, African children who eat a high fiber diet showed a significant enrichment in Bacteroidetes and depletion in Firmicutes (P < 0.001), with an abundance of bacteria from the genus Prevotella and Xylanibacter. These bacteria are known to have genes that hydrolyze cellulose and xylan. Meanwhile, these findings were not observed in European children [64].
It appears two opposing theories are conflated suggesting a third factor may be involved in “diet drives microbiome change” or “microbiome alters dietary absorption” leading to obesity. A recent study explained that “microbes are highly varied between individuals and fluctuate within an individual.” [65] Furthermore, another study reported “no simple taxonomic signature of obesity in the microbiota of the human gut” [66]. In a meta-analysis, Sze and Schloss concluded that most of these sequencing studies are underpowered and used inappropriate statistical methods, and more importantly, they may show associations but not causality [67].
Often many dental researchers who are not knowledgeable in the concept of confounding combine the groups together like those who have diabetes and periodontitis or those who smoke and have periodontitis and claim that periodontal treatment improved CVD or glycemic control. In these cases, the confounding by diabetes or smoking must be controlled meticulously, or the results will be biased.
Another point that should be considered in data management is when smoking is dichotomized; it should never be smoking = “yes/no.” Even though CVD risk declines with increasing time from smoking cessation, past smokers are at an increased risk of having CVD, and this dichotomy wrongly places them in the “no” category. If one must dichotomize smoking, it should be “ever smoke = yes/no.” In this scenario, the past smokers and current smokers are grouped together which is more appropriate. Alternatively, a continuous measure of smoking exposure, such as pack-years, can be employed to distinguish those with little smoking exposure to those with heavy smoking exposure.
A recent classic example of a hidden confounder that has misled the biomedical research and clinical trials is the fact that at high-dose regimens of bisphosphonates (BISPs) for cancer patients and repeat doses over 3–5 years, the cumulative dose on the bone reaches to high enough level that it impacts bone osteocytes and bone lining cells. This approach in preclinical and clinical trials led the investigators evaluating the cancer bone metastasis and cancer bone burden to observe ~20–30% reduction. This was interpreted in terms of BISPs having direct anticancer effect. Remarkably, the impact of BISPs on the bone which reflects degeneration of the local bone cells and bone vitality becomes a confounding factor since the dead bone cannot support cancer colonization, and hence reduction in cancer bone burden was not directly attributable to the effect on cancer cells.
8.5 Minimizing Biases
Recently elaborate 16r RNA sequencing was done in the subgingival crevicular fluid of patients who have systemic lupus erythematosus (SLE) with and without periodontitis. They observed dysbiosis in the group with periodontitis [68]. The question still remains: “Is dysbiosis due to periodontitis?” or “Are both dysbiosis and periodontitis the phenotypes clustering immune-suppressive treatments of SLE?” In translational research or in any research activities, the ultimate goal is reducing diseases. To achieve this goal, we must decrease the exposure to causal risk factors. Therefore, it is of utmost importance to find causal factors if we wish to lower human diseases occurrence.
8.5.1 Simpson’s Paradox
Simpson’s paradox is defined as “the results indicate the reverse of the true relationship because a confounding factor is not considered.” A source of bias in some translational research originates from the lack of epidemiologic understanding among bench scientists. Some researchers reported, “Obesity alters gut microbial ecology” [51]. The same group also reported “gut microbiome contributes to energy harvest from the diet and energy storage in the host (i.e., caused obesity)” [69]. These two theorems have opposing cause-effect directions. Backhed et al. also reported that “introduction of a gut microbiota into adult germ-free mice caused a 57% increase in body fat” [69].
Alternatively, many researchers reported that diet-induced obesity alters gut microbiome [26, 57, 70–73] and this process involves toll-like receptor activation followed by cytokine production that is manifested in metabolic inflammation [74–77]. Utilizing antibiotics and changing the gut microbiome in leptin-deficient ob−/ob− mice suppressed metabolic endotoxemia, inflammation, and associated disorders [54]. The absence of CD14 in the same mouse group brought similar effects to antibiotics suggesting that innate immune sensing is involved in obesity and that CD14 acts as a co-receptor (along with the Toll-like receptor TLR 4 and MD-2) for the detection of bacterial lipopolysaccharide (LPS). We must remember that the innate immune system can be activated by both microbial and metabolic stimuli [61, 78–80]. Indeed, Fleissner et al. reported that absence of intestinal microbiota does not protect mice from diet-induced obesity [81], and another study refuted the highly cited claim by Turnbaugh et al. [53] that energy harvest from short-chain fatty acids by microbiota in the gut caused obesity [82]. Murphy and colleagues observed a progressive increase in Firmicutes which was confirmed in both HF-fed and ob/ob mice (we interpret this as the diet and obesity altered gut microbiome). But the changes in the microbiota were not associated with the marker for energy harvest [82]. At this time, it is not clear whether an obesogenic diet causes gut microbial changes or gut microbiome alteration caused obesity.
A potential Simpson’s paradox is possible in the case of childhood infections or antibiotic use causing preadolescent obesity [83, 84]. All these studies ignored the fact that infectious inflammation can be confounded by metabolic inflammation [61, 85]. In a recent longitudinal study, infection and antibiotic use in infancy were reported to be causally associated with obesity in the adolescent [86]. However, this study ignored the main culprits of obesity, namely, obesogenic diet, insufficient physical activities, and the resultant energy imbalance [26, 61].
The mainstream thesis on the cause for obesity is still the excess energy due to high caloric intake and lack of physical activity [26]. One study compared high and low Toll-like receptor 5 (TLR5) gene expression and obesity. The group with high TLR5 gene expression was obese because of the flagellin-producing microbiota detected by TLR5 [87]. However, a Simpson’s paradox may have occurred because those who have high expression of TLR5 gene were fatter at baseline (BMI = 30.6 vs 20.7, p = 0.04), much more insulin and leptin resistant. This finding is directly opposite to that of a highly touted animal study in molecular science [88]. In the latter study, Vijay-Kumar et al. reported that TLR knockout mice developed spontaneous obesity and metabolic syndrome [88] and the transfer of the fecal microbiome from TLR5-deficient mice to wild-type germ-free mice caused obesity and metabolic syndrome. We are not certain whether mice and humans will have the same innate immune response triggered by TLR5 activation or if there are some built-in biases in these studies. Clearly, more incisive review of the studies using TLRs in animals and humans are needed. Our opinion is that TLR activation is the result of obesity which also caused metabolic inflammation as we and others have reported [61, 89]. Numerous studies support this thesis that TLR activation is the result of obesity: TLRs were activated in nonalcoholic fatty liver disease [90] and other obesity-related cardiometabolic diseases [91, 92].
In another study, the third trimester (T3) stool of pregnant women showed the strongest signs of inflammation and energy loss. When their fecal microbiotas were transferred to germ-free mice, T3 microbiota induced greater adiposity and insulin insensitivity compared to that of first trimester [93]. This study ignored the fact that pregnancy is an immune-tolerant state, and as the fetus grows, inflammation increases due to more relaxed immunity not to reject semi-allogeneic fetus. Thus, it is plausible that T3 stool would display more prominent metabolic dysfunction and inflammation.
8.5.2 Conundrum in Microbiome Research
Lately, popular topics in research include gut microbiome and gene sequencing. These clinical and translational research studies hold significant potential impact for leading to improve understanding and ultimate application to dental disease and cardiovascular disease. But since this is a new area of research, it is fraught with many deficiencies arising from inappropriate methodology, misconceived study rationale, and misinterpretation of results. Consequently there are many conflicting reports. Beyond potential reverse causation due to using feces to estimate gut microbiome function, additional reasons for this disparity exist: the high functional redundancy in host-microbiome interactions, normal individual variation in microbiome composition, differences in study design, diet composition, the host system between studies, and inherent limitations to the resolution of rRNA-based microbial profiling [94].
Initial evidence for obesity-altered microflora came from an animal study that leptin-deficient ob/ob mice displayed a decrease in Bacteroidetes and a proportional increase in Firmicutes compared with lean siblings (ob/+) given the same diet [51]. Confounders in the relationship of gut microbiome and obesity are diet and the genetic lineage of the animals [71].
Some microbiome studies used germ-free mice to prove gut microbiota cause obesity. We question the validity of using germ-free mice and extrapolating the results to humans. Although infection can change metabolism, the germ-free state in humans is unnatural, and its clinical interpretability is limited. At birth, the gut of a human newborns is sterile but by passing through the birth canal, subsequent breast feeding, and introduction of solid foods, the infant’s gut is colonized with a microbial community [95]. This colonization has multiple benefits because microbiome educates the developing immune system [96] and trains it how to distinguish harmful pathogens from harmless commensals, or part of self, and to react accordingly [97, 98]. If this introduction of microbiota is disturbed, some autoimmune disease, such as Type 1 diabetes, may occur [99]. Another benefit of having well-colonized microbiome is breaking down indigestible food components, degrading potentially toxic food compounds like oxalate, and synthesizing certain vitamins and amino acids [100]. Additionally, a more powerful driver of obesity and metabolic syndrome is diet and physical activity balance [26]. Diet changes gut microbiome and intestinal permeability which allows some microbiota to translocate into the blood stream [57].
Additionally, the gut microbiome is usually assessed in the feces and is likely to be the results of obesity rather than the cause. For this reason, the fecal transplant from lean persons improved insulin sensitivity, and it is plausible because lean donors have the normal microbial community, which is not affected by obesity [101]. However, it is not clear whether these changes are permanent or if insulin resistance will return as soon as the recipients resume their obesogenic diets. Moreover, microbiome change is transient [102], and perhaps, it is prudent to make dietary change and increase energy expenditure by exercising more. As we have stated in the previous section, that fecal microbiome assessment is not appropriate for establishing the causal role of microbiota in the gut immunity. In addition, modulating gut microbiome by antibiotics appeared to improve insulin signaling and glucose tolerance by reducing circulating LPS levels and inflammatory signaling in mice [103]. However, this phenomenon was not duplicated in humans in a recent randomized trial [104]. Moreover, using antibiotic treatment to mimic germ-free state in an attempt to prevent obesity via changing gut microbiome in humans has some obvious problems such as the development of resistance to antibiotics. Additional problems using antibiotics are gut microbiome is necessary to protect the host from invading pathogens, energy extraction, and developing immune system [100]. Moreover, reduced exposure to important gut bacteria may result in higher incidence of human allergies and autoimmune diseases [105].
Current knowledge of the mucosa-associated bacterial communities in the intestine and colon is limited because the knowledge was largely based on fecal microbiome analyses. It was reported that the lumenal and fecal bacterial communities were significantly different [106, 107]. It was demonstrated that the cecum contained 100 times more bacteria than the terminal ileum [108]. Admittedly, collecting colonic samples is difficult because of their viscosity and the difficulties in ensuring adequate anaerobic conditions. This proves potential sources of discrepancy in aerobic fecal and anaerobic intestinal microbiome. Only intubation and pyxigraphy can be performed in healthy subjects, and both should be repeated to study the stability of the flora or the influence of various parameters on its composition [106]. Some microorganisms, such as the methanogens, represent <0.003–0.03% in the right colon, and the same bacteria are present at 5–12% or more of all bacteria in the feces. Strict anaerobes analyzed using probes specific for the Bacteroides (Bacteroides, Porphyromonas, and Prevotella spp.) and Clostridium groups (Clostridium, Eubacterium, and Ruminococcus spp.) revealed that these bacteria represented 44% of fecal bacterial rRNA and only 13% of cecal bacterial rRNA. These differences suggest that studying the right-sided colonic flora would be more appropriate than studying feces for the diseases involving the right part of the colon, such as ileocecal Crohn’s disease [106].
Many studies report the dietary intake shaping gut microbiome [109] as well as causing obesity [54, 110, 111]. However, equally numerous studies report gut microbiome causing obesity independent of dietary effects. Some studies, once scrutinized carefully, erroneously reported gut microbiome causes obesity although dietary factors precede alteration in gut microbiome [53, 94, 112]. Clearly, the lack of understanding of the causality principle, namely, the temporal relationship, made them to refer an imprecise association as causal relationship [53, 112]. Furthermore, the gut microbiome consisting of approximate 1000 species and their composition can change due to antibiotics, illness, stress, aging, bad dietary habits, and other lifestyle factors [113]. Gut microbiome evolves with the human development from germ-free state of newborn infants to approximating adults’ microbiome by the age of 1–3 years [114]. Incidental environmental exposures play a major role in determining the distinctive characteristics of the microbial community [114].
A recent randomized trial utilizing Lactobacillus rhamnosus GG has been shown to decrease neuropsychiatric disorders later in the childhood by stabilizing gut permeability and restoring epithelial barrier function by tight junction control, mucin production, and antigen-specific immunoglobulin A production [115]. The underlying pathology in many autoimmune or allergic disorders is the increased intestinal permeability that brings dysregulation of immune responses as well as dysbiosis in response to ubiquitous environmental antigens. It should be noted that obesity causes increased intestinal permeability [116]. Thus, finding the very first initial trigger may prove to be a causal factor.
8.5.3 Toll-Like Receptors in Infection and Metabolism
Germ-free mice [55] were protected from developing obesity even with a high-fat diet while Toll-like receptor 5 (TLR5) knockout mice became obese and hyperphagic [88]. These results prompted the conjecture that infection or the gut microbiotas may be at the root of obesity [88]. However, in an in vitro study, subcutaneous adipocytes cultured and exposed to saturated fatty acids expressed increased TLR4 and MyD88 and upregulated NF-kB activity with significantly increased secretion of IL-6 and TNF-α [117]. This suggests fatty acids caused TLR4 expression, and not TLR4 caused fat-related inflammation observed in obesity. This further proves that TLR4 can be activated by metabolic factors [61].
As we wrote in the previous section, a high-fat diet (HFD)-fed mice expressed increased LPS in the serum (metabolic endotoxemia) [57] and activated TLR4. TLR4, in turn, induced enteric neuronal apoptosis in a p-JNK1 dependent pathway [118]. The authors also observed that the HFD-fed mice had a statistically significant reduction in Bacteroidetes (P < 0.001) and a significant increase in Firmicutes, Bifidobacteria, and E. coli (P < 0.001) relative to mice fed a regular diet. When they supplemented the mice’s diet with oligofructose (prebiotic), the level of endotoxemia decreased in HFD-fed mice. The researchers interpreted prebiotics restored dysbiosis, but we consider that prebiotics prevented high-fat diet induced intercellular permeability which resulted in a lesser degree of dysbiosis. Again, it was proven that the high-fat diets initiated increased intercellular permeability, metabolic endotoxemia, and gut dysbiosis and also activated TLR4, which in turn induced intestinal neuronal apoptosis resulting in gut motility reduction [118]. It is important to recognize which factor initiated the sequence of the events and that factor should be considered as the cause.
A recent human trial largely refuted all the animal studies reporting that gut microbial colonization may cause obesity. Reijnders and colleagues manipulated gut microbiota by antibiotics (7-day administration of amoxicillin, vancomycin, or placebo) and observed host metabolism in 57 obese, prediabetic men. Vancomycin, but not amoxicillin, decreased bacterial diversity but did not affect tissue-specific insulin sensitivity, energy/substrate metabolism, postprandial hormones and metabolites, systemic inflammation, gut permeability, and adipocyte size. More importantly, energy harvest, adipocyte size, and whole-body insulin sensitivity were not altered at 8 weeks of follow-up, despite considerable alteration in microbial composition [104]. We interpret this as antibiotics, or the lack of innate immune sensor such as TLR5 may alter the gut microbiome but may not affect metabolism or obesity. This was also the view of an expert who first reported diet-induced endotoxemia, increased serum levels of LPS due to increased intestinal permeability [119].
Germ-free mice colonized with Bacteroides thetaiotaomicron had improved host nutrient absorption and thus potentially increases the possibility of developing obesity [120]. However, the multicomponent ileal/cecal flora produced no significant change in levels of either mRNA compared with germ-free controls [120]. We interpret these results as “germ-free mice being colonized with one or two microbes may introduce bias because it is acting as an infection while multi-microbial inoculation may have balancing effects among the microbes” and produced less detrimental impacts.
8.6 Reducing Measurement Errors
Traditionally, translational studies tend to have a lesser degree of measurement error than large epidemiologic studies. However, it is still possible that measurement errors may lead to paradoxical results. For example, the gut microbiome includes over 1000 microbial species [121], and identifying a few microbes that are causally associated with the disease of interest is truly a daunting task. This also applies to all the large-scale proteomics studies by mass spectrometry and/or protein arrays where definition of a biomarker for causal or diagnosis is evaluated.
Traditional culture-dependent methods have numerous drawbacks such as the time and money required, difficulties in identifying the different colonies grown in agar, the lack of sensitivity, predilection for the most common culture conditions favoring fast-growing and easy-growing species, and ignoring those in low concentration or requiring unusual culture conditions, such as anaerobic conditions. Conversely, the cutting-edge analytical method of 16S rRNA also has several limitations: Firstly, the accuracy of identification is directly dependent on the completeness of the reference database. Secondly, the identification power is lower at the species than higher taxonomic levels. Thirdly, many studies use a fragment of the gene, which restricts its discriminatory power even more. Fourthly, many bacterial species have more than one copy of 16S rRNA, and inter-copy sequence variations may be present [121]. In addition, the presence of microbe in the diseased tissue does not prove that microbe caused the disease. Some microbes have the unusual capability of slipping through intercellular spaces and are ubiquitously present in many diseased and non-diseased tissues. Some examples are Fusobacterium nucleatum (F. nucleatum) and Porphyromonas gingivalis (P. gingivalis). Whether they are innocent bystanders or truly causal microbiotas has yet to be proven. The reason for that is the majority of the studies have some methodological flaws. For example, oral gavage with P. gingivalis resulted in intestinal dysbiosis in mice [122]. This study provided a novel concept that orally ingested microbial species can cause gut dysbiosis linking the oral cavity to the gut microbiome. However, to prove that P. gingivalis is unique in causing gut dysbiosis, the control group should have been other microbiotas, such as Salmonella, Escherichia coli, or Staphylococcus. Using saline as control, they had proved that ingestion of “bacteria,” not specifically P. gingivalis, caused dysbiosis which is not unlike food poisoning. Additionally, ingesting P. gingivalis is not the same as P. gingivalis present in human periodontitis. To be a cause for an infection, microbiota must overcome several obstacles [123]: First, they must outcompete the huge number of commensal bacteria [124]; second, they must disrupt epithelial barrier function [125]. In the manipulation of epithelial barrier function, several mechanisms have been recognized. One is via over expression of IL-6 [126] or manipulation of the actin cytoskeleton [127]. Here we need to be reminded that obesity and metabolic inflammation overexpress IL-6 [128, 129] and also increase intercellular permeability.
Another example of the use of inappropriate control group is highly touted “Justification for the Use of Statins in Primary Prevention: An Intervention Trial Evaluating Rosuvastatin (JUPITER)” trial where in a cohort who were overweight, many of them smoked, and were hypertensive but had not yet developed heart disease, these subjects were given rosuvastatin and the results were compared to the control group who took placebo. Certainly this cohort needed to lower their body weight and decrease smoking and hypertension by lifestyle changes. Thus the appropriate comparison group should have been lifestyle changes comparable to pharmaceutical intervention. Moreover, the outcome of cardiac events included “hospitalization for unstable angina” in the arithmetic sum of all cardiac events. This means “hospitalization for unstable angina” had equal weight as did myocardial infarctions or cardiac deaths. This is clinically inappropriate. When we look at the major cardiac events only, the cardiac event rate in the rosuvastatin group was 83/8901 and numerically 0.009 which means less than 1% had a cardiac event. The placebo group event rate was 157/8901 which can be translated as 0.018 which is less than 2%. And yet, the relative risk decreased about 50% (0.009 vs 0.018) with rosuvastatin administration. Despite the low actual number of events involved in this cohort, now statin treatment for the asymptomatic population is accepted as a standard of care. Some minute improvement in outcome can be manipulated to be highly significant by increasing the sample size because the power of a study (i.e., the probability of obtaining a statistically significant result) is dependent on the sample size. P-value is calculated by a Z-score which indicates how many standard deviations the observed value is away from the mean.

X: sample mean; μ: population mean or true mean; σ: standard deviation (SD); n: sample size.
As we know X, sample mean, and σ, standard deviation, come from the experiment results which should not be changed. However, the sample size can be manipulated by recruiting a large number of participants. If the sample size (n) increases, the denominator becomes smaller because denominator is SD divided by the square root of the sample size. If the denominator is small, even minute changes in the enumerator (the outcome) can generate a large Z-score, and the P-value becomes significant. Thus, it is important to realize that although the P-value is significant, sometimes the results may not be clinically meaningful. Inevitably, with a large sample size, often the measurement will be done by using questionnaires or proxies that are less precise. Consequently, their results are often imprecise but highly significant. Nevertheless, journals and funding agencies tend to believe results from studies with large sample sizes. Hence, it is always important to ask whether statistical significance is actually clinically relevant. An extension of the above concept can be also found in the misleading conclusions of the experimental data being different by only 10–20% between the comparators with P-value <0.05 and hence touted as “statistically significant”. In most cases a difference of 10–20% between the compared groups, although it may be statistically significant, such data or changes are frequently not biologically significant or relevant.
8.7 Utilizing the Appropriate Statistical Methods
Translational research often involves small sample size because the elaborate laboratory methodology requires time and money to conduct. Also, the results are affected by the techniques used (mass spectrometry or polymerase chain reaction, etc.), researchers’ skills to perform the experiment, and the animal models or species used. In some research, using the appropriate animal model is important. For example, in short-chain fatty acids assessment, murine models may be of limited value, while pigs or dogs are much better to estimate the human relationship with short-chain fatty acids and gut microbiotas.
The pervasive problems in translational studies are sample sizes are too small and using inappropriate statistical methods. When the sample size is five or six in each group, we cannot expect that these data will have a normal distribution. However, many researchers use the t-test which assumes that the underlying data has a normal distribution. Also, multigroup comparisons often use ANOVA, but the ANOVA requires that each compared group must have a normal distribution and each group must have the same sample size. Even a total sample size of 32 (each group consists of N = 8 in four groups), the each group (n = 8) must be normally distributed. In addition, for ANOVA , homogeneity of variance assumption is crucial to obtaining valid statistical results. Particularly in laboratory studies involving count data, variance may increase exponentially with group means, which can be problematic. Especially, in some 16s rRNA sequencing, the usual sample size is less than ten due to the constraints of cost, time, and computing ability. So, the sample size issue has been raised with regard to the gut microbiota research in a recent meta-analysis, and the median classification accuracy for predicting obesity by the gut microbiome composition was very modest, being between 33.01% and 64.77% [67].
One of our own students conducted a four-group comparison where how various reagents affect microbial growth. The total sample size was over 200, but the underlying assumption of homogeneity of variance was violated, and several different experimental variations were involved such as the timing of adding reagents, different numbers of microbes added at the beginning of the experiment, and differing composition of microbes. Due to these limitations in study design and data distribution, we could not use parametric regression methods. Thus, we created each subgroup reflecting on the variation in the methodology and compared to appropriate reference via nonparametric methods. In addition to concerns about sample size and variance, problems arise when the underlying distributions under comparison are highly skewed. In most biological data where the groups being compared are highly skewed, it is generally more appropriate to utilize nonparametric testing.
Some randomized trials select egregiously poor reference groups to amplify the efficacy of their interventions. For example, if the intervention is giving milk to school children and assessing obesity outcome, the correct reference group should be drinking water [130] or diet soda [131]. If the chosen reference group is sugared soda which has been established as obesogenic [132], the results may not substantiate much health benefit from drinking milk.
Another example of using an inappropriate reference group can be found in a heart failure medicine trial. In a pharmaceutical company sponsored PARADIGM-HF trial [133], a new added ingredient LCZ696 to a previously marketed angiotensin receptor blocker valsartan was tested. Since the new product has a new added ingredient to valsartan, the appropriate reference group should be valsartan without LCZ696 and substantiate that added ingredient is safe and equally efficacious or better. However, they compared its efficacy to enalapril, an early angiotensin-converting enzyme inhibitor with well-known side effects of cough in many patients. To our opinion, since this drug will be given to advanced heart disease patients, the safety issue should be tested carefully. We do not comprehend why this point is not recognized by the leaders of the American Cardiologists group.
In conclusion, even in translational research, all of the epidemiologic principles such as developing scientifically sound rationale, establishing causal relationship, controlling for confounding, minimizing biases and measurement errors, and using appropriate statistical methods are of paramount importance.

VIDEdental - Online dental courses
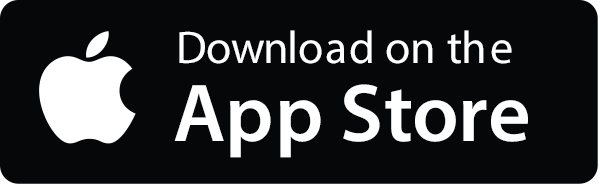
