Abstract
Objectives
To review the history, theory and current applications of Weibull analyses sufficient to make informed decisions regarding practical use of the analysis in dental material strength testing.
Data
References are made to examples in the engineering and dental literature, but this paper also includes illustrative analyses of Weibull plots, fractographic interpretations, and Weibull distribution parameters obtained for a dense alumina, two feldspathic porcelains, and a zirconia.
Sources
Informational sources include Weibull’s original articles, later articles specific to applications and theoretical foundations of Weibull analysis, texts on statistics and fracture mechanics and the international standards literature.
Study selection
The chosen Weibull analyses are used to illustrate technique, the importance of flaw size distributions, physical meaning of Weibull parameters and concepts of “equivalent volumes” to compare measured strengths obtained from different test configurations.
Conclusions
Weibull analysis has a strong theoretical basis and can be of particular value in dental applications, primarily because of test specimen size limitations and the use of different test configurations. Also endemic to dental materials, however, is increased difficulty in satisfying application requirements, such as confirming fracture origin type and diligence in obtaining quality strength data.
1
Introduction
This paper reviews Weibull statistics in order to facilitate informed decisions regarding practical use of the analysis as it applies to dental material strength testing. Weibull statistics are commonly used in the engineering community, but to a somewhat lesser extent in the dental field where applicability has been questioned . A possible confusing factor is that Waloddi Weibull originally presented his analyses partially on empirical grounds; published theoretical confirmation was not available until many years later. Even as a theoretical basis was being constructed, Weibull, as an engineer, seemed more concerned with what worked . In order to assess applicability to the specific field of dental material strength testing, it is helpful to start with a brief history and overview of basic concepts of extreme value theory, fracture mechanics, and flaw populations as they pertain to the theoretical foundation of Weibull analysis.
1.1
Background: brittle failure prediction, fracture mechanics and flaws
It is often noted that dental restorative ceramics and composites, while popular in terms of esthetics and biocompatibility, are susceptible to brittle fracture. This type of failure is particularly difficult to predict. Imminent brittle fracture is seldom preceded by warning, such as visible deformation, nor do seemingly identical brittle components appear to break at the same applied stress. Ductile materials deform to evenly distribute stresses throughout a region, but in stiff, brittle materials, stress concentrations at specimen geometry changes, cracks, surface irregularities, pores and other intrinsic flaws are not relieved. Hence the design methodologies and test methods for ductile materials are unsuitable for brittle materials and different test methods and design approaches are needed.
A series of catastrophic events, often epitomized by the Liberty ship and Comet airplane disasters, spectacularly demonstrated the inapplicability of ductile strength testing analyses to brittle failure prediction. Such disasters spurred the development of the science of fracture mechanics in order to understand the conditions of failure through crack growth rather than by ductile mechanisms .
In the early 1920s, Griffith postulated that crack extension in brittle materials occurs when there is sufficient elastic strain energy in the vicinity of a growing crack to form two new surfaces . In the 1950s Irwin built on Griffith’s work to associate crack extension with an “energy release rate” . This led to a new parameter, K Ic —fracture toughness, or resistance to crack growth. Irwin’s approach enabled strength predictions based on fracture toughness calculations that relate crack extension to the sizes of preexisting cracks or “flaws” within a material. For the most common case of a small flaw in a far field tensile stress field, modern fracture mechanics relates the applied fracture stress at the fracture origin, σ f , to a flaw size, c :
σ f = K I c Y √ c
where Y is a dimensionless, material-independent constant, related to the flaw shape, location and stress configuration and is called the stress intensity shape factor. “Flaws” are not necessarily inadvertent defects or blemishes in a material. No material is perfectly homogenous, and all contain some sort of discontinuities on some scale. These discontinuities might be pores, inclusions, distributed microcracks associated with grain boundaries or phase changes during processing, regions of dislocations or slight variations of chemistry, or many other possible variants and combinations. They could also be surface distributed flaws such as grinding cracks. Flaws in this sense are intrinsic to the material or the way it was shaped or processed, and are distributed in some way throughout the material surface or volume. It can be seen from the equation that the smallest strengths are associated with the largest flaw sizes.
The idea of failure being associated with a largest flaw, or “weakest link theory”, is not recent. Leonardo DaVinci is reputed to have conducted tests circa 1500 involving baskets suspended by different lengths of wire of nominally identical diameter . DaVinci gradually filled the baskets with sand and noted the baskets suspended by shorter wires could hold more sand, an outcome that is expected if it is assumed that there is a lower probability of encountering a large flaw in a shorter wire. DaVinci did not know exactly where a particular wire would break, but he recommended that multiple tests be done for each wire length, suggesting that there was a statistical variation in the strengths and failure locations of wires of a the same length. Since DaVinci’s time, much progress has been made both in our ability to identify critical flaw properties and in refining predictions based on weakest link theory and statistical failure probabilities.
1.2
Extreme value distributions of “largest flaws”
Suppose a material has a flaw size distribution such as the normal, or Gaussian, distribution illustrated in Fig. 1 . Although the flaws have different sizes, suppose they are all of the same general type that initiate failure. Finally, suppose that small material test specimens are withdrawn from this parent population. Each test specimen will contain a discrete flaw population based on the parent distribution, and the largest flaw within the highly stressed regions of each test specimen will precipitate failure if uniform tension is applied.

If one is very unlucky, a randomly withdrawn material test specimen will contain an unusually large flaw, at the very end of the tail at the right of the parent distribution in Fig. 1 . The test specimen will be very weak. If one is very fortunate, however, the largest flaw in a random material test specimen will not be far into the tail. This specimen will be relatively strong. If many random test specimens are withdrawn, most of them will contain a largest flaw that is somewhere within the parent population tail of large flaws. The “largest flaw” distribution might look like the shaded portion of Fig. 1 .
The distribution of “largest flaws” of many withdrawn test specimens from a parent population is an example of an extreme value distribution. The “largest flaw” population is not expected to be a symmetric distribution, whether or not the parent population is Gaussian as in Fig. 1 example.
Suppose now that larger-sized test specimens of the same material are tested. Each of these physically larger test specimens contains more flaws than each of the smaller test specimens in the previous thought experiment. Since a larger test specimen contains more flaws, it is more likely to contain a very large flaw corresponding to the far right portion of the tail in Fig. 1 parent population. If many larger-sized test specimens are withdrawn, their “largest flaw” distribution will be weighted further to the right within the parent population tail than the previous “largest flaw” distribution of smaller test specimens. The “largest flaw” distributions “march to the right” as the physical sizes of the test specimen increase.
One result of “largest flaw” distributions that depend on the physical size of test specimens is that strength distributions, which are based on flaw sizes, will similarly depend on test specimen size. Strength distributions will inversely “march” left or right in accordance with “largest flaw” distributions in a mathematically predictable fashion. Also, strength distributions would not be expected to be symmetric if the “largest flaw” distributions are not symmetric.
Currently, many data in the dental literature are simply reported in terms of mean values with standard deviations calculated by assuming symmetric, normal strength distributions about an average. This assumption can still yield insight into material strengths and strength ranges. In fact, the assumed normal strength distribution may not greatly differ from an extreme value distribution in providing strength estimates of similarly sized and stressed test pieces. Unfortunately, such predictions only hold for the specific test specimen size and shape in the particular test configuration under laboratory conditions. As will be shown, besides more accurately characterizing material strength, extreme value statistics are a powerful tool that yield parameters that can be used to relate test strength data to expected strengths for different stress configurations, different test specimen sizes, and different testing conditions.
1.3
The Weibull distribution
Returning to Fig. 1 , it can be seen that the parent distribution tail at the right is much more important than the rest of the parent distribution containing smaller flaws. Since small flaws in the test specimens do not precipitate failure, it does not matter how small these flaws are or how their sizes are distributed. The left side of the total flaw distribution in Fig. 1 has very little bearing on the “largest flaw” distribution.
The original total flaw population does not have to be normal or symmetric to result in an extreme value distribution shaped similar to the shaded portion in the figure. The right side of the parent flaw distribution, the tail of large flaws, dominates the shape of the “largest flaw” distribution. This is because only one very large flaw in a test specimen is necessary to be counted as “the largest flaw”, but if the largest flaw is relatively small, all the other flaws in the test specimen have to be smaller. The distribution is thus skewed to the right as in Fig. 1 , following the parent distribution tail. Extreme value distributions in general “follow the tail” . Focusing only on the tails allows simple, generalized parametric functions to be used in extreme value statistical models. Such models have been defined to accommodate underlying distribution tails that deviate considerably from the Gaussian shape in Fig. 1 example.
There are three commonly recognized families of extreme value distributions where G ( x ) is the probability distributions function for an outcome being less than x for a sample set of n independent measurements:
Type I . Gumbel G ( x ) = exp ( − ex p − ( x − μ ) / σ ) for all x
Type II . Fr é chet : G ( x ) = exp ( − ( ( x − μ ) / σ ) − ξ ) for x ≥ μ = 0 otherwise
Type III . Weibull : G ( x ) = exp ( − ( ( μ − x ) / σ ) ξ ) for x ≤ μ = 1 otherwise
where μ , σ (>0), and ξ (>0) are the location, scale and shape parameters, respectively.
Fisher and Tippet are credited with defining the extreme value distributions in 1928, when they showed there could only be the three types. Some graphical examples of the probability density functions of the three types of extreme value distributions are shown in Fig. 2 .

All three extreme value distributions have a theoretical basis for characterizing phenomena founded on weakest link theory. For strength dependency on an underlying material flaw distribution, the goodness-of-fit of any of the extreme value distributions depends on the shape of the flaw distribution tail. In this regard, Type III, or the Weibull distribution, is usually considered the best choice because it is bounded (the lowest possible fracture strength is zero), the parameters allow comparatively greater shape flexibility, it can provide reasonably accurate failure forecasts with small numbers of test specimens and it provides a simple and useful graphical plot . In what has been hailed as his “hallmark paper” in 1951 , Weibull based the wide applicability of the distribution on functional simplicity, satisfaction of necessary boundary conditions and, mostly, good empirical fit. The parameter symbols and form of the extreme value functions are usually written differently for reliability analyses. In the specific case of Weibull fracture strength analysis, the cumulative probability function is written such that the probability of failure, P f , increases with the fracture stress variable, σ :
P f = 1 − exp − σ − σ u σ m
This is known as the Weibull three parameter strength distribution. The threshold stress parameter, σ u , represents a minimum stress below which a test specimen will not break. The scale parameter or characteristic strength, σ , is dependent on the stress configuration and test specimen size. The distribution shape parameter, m , is the Weibull modulus. This is the equation form that Weibull presented in his original publications, directly derived from weakest link theory . He was conservative and disclaimed any theoretical basis, not because of misgivings concerning extreme value or weakest link theory, which was well established by then, but because he perceived it was “hopeless to expect a theoretical basis for distribution functions of random variables such as strength properties”. Since Weibull’s initial publications in 1939 and 1951, however, the science of fracture mechanics has enabled determinations of quantitative functional relationships between strength and flaws in brittle materials, as exemplified in Eq. (1) .
By 1977, Jayatilaka and Trustrum used fracture mechanics to develop a general expression for the failure probability using several general flaw size distributions suggested by experimental work. They coupled these flaw size distributions with the fracture mechanics criterion, Eq. (1) , and integrated the risk of breakage over component volumes and then derived a number of different strength distributions . Their derivations are too lengthy to repeat here and the reader is encouraged to review their exposition in the original references. They showed that the right side tail of many parent flaw size distributions such as shown in Fig. 1 often can be modeled by a simple power law function: f ( c ) = constant c − n where c is the flaw size. In such cases, the resulting strength distribution is the Weibull distribution with m = 2 n − 2. Danzer et al. have done similar derivations with more generalized flaw size distributions and have reached the same conclusions. Subsequent work, including painstaking measurement of flaw sizes and constructing distributions, has confirmed the power law function for the distribution of large crack sizes and hence the theoretical basis for the Weibull approach . In other words, a reasonable power law distribution for large flaw sizes, classical fracture mechanics analysis, and weakest link theory lead directly to the Weibull strength distribution.
Today’s engineers routinely utilize Weibull statistics for characterizing failure of brittle materials. Numerous and diverse studies in the engineering literature report data in terms of Weibull parameters where the strengths are related to fractographically determined flaw types and sizes . Such studies substantiate the existence of flaw distributions that lead to strength distributions that can be modeled by Weibull statistics for a wide range of materials. As noted earlier, fractographic examinations are becoming more common in relating flaw types and sizes to strengths of dental restoration materials . While this shows that characterizing flaw populations in dental materials is possible, this does not prove it is easy or possible in every case. Many dental materials have rough microstructures, where it can be particularly difficult to identify fractographic features . Also, it is not unusual to have multiple flaw types present which complicate the Weibull analysis.
Major standards organizations throughout the world have published specific guidelines for reporting ceramic strength in terms of Weibull parameters, including ASTM (C1239) , the Japanese Industrial Standards Organization (JIS R1625) , the European Committee for Standardization (CEN ENV 843) , and the International Organization for Standards (ISO 20501) . These standards are very similar and use the identical Maximum Likelihood Estimation analysis to calculate the Weibull parameters. A newly revised (2008) Ceramic Material for Dentistry standard (ISO 6872) is the sole exception and uses a simpler linear regression calculation in an informative annex.
Since many brittle materials used in structural engineering are also used in restorative dentistry, are Weibull analyses appropriate for ceramic dental materials? To answer this, the specific underlying assumptions and conditions inherent in applying Weibull statistics must be examined.
2
Considerations for using Weibull statistics for dental materials
A good data set is required for any credible property determination, and it can be deduced from the previous paragraphs that diligence in test specimen preparation and testing procedures is particularly important when using Weibull statistics. Test specimens breaking from inconsistent machining or handling, or haphazard alignment, are not representative of a specific flaw population, and do not contribute to a valid data set for Weibull analysis. A materials advisory board committee in 1980 concluded that: “Ceramic strength data must meet stringent quality demands if they are to be used to determine the failure probability of a stressed component. Statistical fracture theory is based on the premise that specimen-to-specimen variability of strength is an intrinsic property of the ceramic, reflecting its flaw population and not unassignable measurement errors. Ceramic strength data must be essentially free of experimental error.” Even with meticulous test implementation, however, it is the stipulation of a single flaw population that seems to cause the most difficulty in using Weibull statistics in dental material strength testing.
In Eq. (1) , the parameter Y distinguishes different types of flaws as well as different test specimen test configurations. A blunt flaw, such as a pore, is more benign than a sharp flaw, such as a microcrack. A material under load may break from a sharp flaw but not break from a blunt flaw of a similar size. Suppose a material contains the two flaw types, small microcracks and large pores. Each flaw type has its own distribution. If all the test specimens break from microcracks, or all the test specimens break from pores, then either the microcrack size distribution or the pore size distribution will govern the strength distribution. If, however, some test specimens break from microcracks, and other test specimens break from pores, the strength distributions resulting from the different flaw populations overlap and an associated extreme value distribution cannot be modeled by one single flaw size distribution tail. In this case, the Weibull strength distribution would not be expected to appear smoothly continuous. If many test specimens are tested and enough of them break from either flaw population, parts of two distinct Weibull distributions may be discernable. Censored statistical analyses must be used in such cases . Bends or kinks in a Weibull distribution function are often indicative of fracture resulting from multiple flaw types.
Thus, lack of a good Weibull fit is suggestive of an inconsistent underlying flaw population, assuming the material was tested properly and failed in a brittle manner. Conversely, a good Weibull fit is sometimes taken as indicative of a single, dominant flaw type and confirmation of adequate care in testing procedures. Unless material familiarity and previous testing dictates otherwise, it is prudent to verify the cause of fracture initiation. This is often done fractographically and is encouraged, and in cases required, by the standards for Weibull analyses . There even are guidelines and formal standards for fractographic analysis that have been prepared with Weibull analysis in mind .
Another consideration in using Weibull statistics is the increased number of test specimens that might be needed to characterize an entire strength distribution rather than simply estimate a mean strength value. The optimal number of test specimens depends on many variables, including material and testing costs, the values of the distribution parameters and the desired precision for an intended application. Helpful calculations and tables to make such decisions can be found in the previously cited standards. In the absence of specific requirements, a general rule-of-thumb is that approximately 30 test specimens provide adequate Weibull strength distribution parameters, with more test specimens contributing little towards better uncertainty estimates . More information regarding optimal test specimen numbers, as well as reasons why Weibull distributions are so often observed in material testing practice are discussed by Danzer et al. . They also detail conditions necessary to obtain a Weibull distribution and suggest alternative statistical approaches for analyzing strength data for materials that do not satisfy these conditions, such as materials with unusual or highly mixed flaw distributions. In this sense, Weibull analysis can be regarded as a special, simple case of a broader statistical approach for analyzing strength data . Indeed, Danzer now uses the expression “Weibull material” as one with a single flaw type whose size distribution fits a power law function on the right side tail.
The previous paragraphs highlight some of the assumptions and difficulties in utilizing Weibull statistics in characterizing strength measurements of dental materials. What are the benefits?
It was initially noted that extreme value statistics can be used to predict changes in distributions according to the physical size of the individual test specimens. This is one of the strongest virtues of the Weibull model and what distinguishes it from other distributions. In practical terms, this means that strength values for one test specimen size may be “scaled” to expected strength values for different sized test specimens. This strength scaling permits comparison of strengths of structures with stress gradients such as bend bars or flexed disks. Examples of strength scaling are shown later in this paper. So far we have assumed that all flaws in a body are exposed to the same tensile stresses, but in bodies with stress gradients, some large flaws may be in a low tensile stress or compression region and will not cause fracture. Fracture occurs where there is a critically loaded flaw that has a size, c , and shape factor Y , and a local stress, σ , combination such that the critical fracture toughness, K Ic , is reached in accordance with eq. (1) . 1
1 For simplicity, we ignore rising R -curve behavior whereby fracture resistance changes with crack size and we also ignore environmentally assisted slow crack growth.
Fig. 3 by Johnson and Tucker illustrates how fracture origin sites may be scattered in a 3-point bend bar. For materials with low Weibull moduli (i.e., the flaw sizes are quite variable) fracture can occur from a large portion of the test specimen. On the other hand, the origin sites are concentrated to only the highest stressed regions if the Weibull modulus is large, since the flaw sizes are all similar.
The validity of the Weibull approach can be tested by its ability to scale strengths for a particular specimen size to another size or testing configuration. A review of ceramic flexural strength data tabulated a number of studies wherein ceramic strength scaling by Weibull analysis was successful over a size range as much as four orders of magnitude in volume or area! Strength scaling is done by the concept of an “equivalent” volume or area under stress, discussed in a following section. Whether equivalent volumes or equivalent areas are used depends on whether fracture initiates from volume flaws or surface flaws within the stressed region. 2
2 In rare instances, strength is controlled by edge origin sites, and strength scales with the effective edge lengths.
Using Weibull statistics to calculate corresponding strengths for different test specimen sizes, test specimen shapes and stress configurations is particularly appealing for use in the dental field where sample sizes and testing configurations are frequently different.
Identification and quantization of different flaw populations can explain strength differences and ultimately lead to improved materials. Such approaches can, for example, isolate the effects of grinding media and surface treatments, or determine whether strength might be improved by reducing porosity. Most important of all, determination of the dominant flaw populations, the effects of stress configurations and physical size are all necessary for correlations to clinical components.
2
Considerations for using Weibull statistics for dental materials
A good data set is required for any credible property determination, and it can be deduced from the previous paragraphs that diligence in test specimen preparation and testing procedures is particularly important when using Weibull statistics. Test specimens breaking from inconsistent machining or handling, or haphazard alignment, are not representative of a specific flaw population, and do not contribute to a valid data set for Weibull analysis. A materials advisory board committee in 1980 concluded that: “Ceramic strength data must meet stringent quality demands if they are to be used to determine the failure probability of a stressed component. Statistical fracture theory is based on the premise that specimen-to-specimen variability of strength is an intrinsic property of the ceramic, reflecting its flaw population and not unassignable measurement errors. Ceramic strength data must be essentially free of experimental error.” Even with meticulous test implementation, however, it is the stipulation of a single flaw population that seems to cause the most difficulty in using Weibull statistics in dental material strength testing.
In Eq. (1) , the parameter Y distinguishes different types of flaws as well as different test specimen test configurations. A blunt flaw, such as a pore, is more benign than a sharp flaw, such as a microcrack. A material under load may break from a sharp flaw but not break from a blunt flaw of a similar size. Suppose a material contains the two flaw types, small microcracks and large pores. Each flaw type has its own distribution. If all the test specimens break from microcracks, or all the test specimens break from pores, then either the microcrack size distribution or the pore size distribution will govern the strength distribution. If, however, some test specimens break from microcracks, and other test specimens break from pores, the strength distributions resulting from the different flaw populations overlap and an associated extreme value distribution cannot be modeled by one single flaw size distribution tail. In this case, the Weibull strength distribution would not be expected to appear smoothly continuous. If many test specimens are tested and enough of them break from either flaw population, parts of two distinct Weibull distributions may be discernable. Censored statistical analyses must be used in such cases . Bends or kinks in a Weibull distribution function are often indicative of fracture resulting from multiple flaw types.
Thus, lack of a good Weibull fit is suggestive of an inconsistent underlying flaw population, assuming the material was tested properly and failed in a brittle manner. Conversely, a good Weibull fit is sometimes taken as indicative of a single, dominant flaw type and confirmation of adequate care in testing procedures. Unless material familiarity and previous testing dictates otherwise, it is prudent to verify the cause of fracture initiation. This is often done fractographically and is encouraged, and in cases required, by the standards for Weibull analyses . There even are guidelines and formal standards for fractographic analysis that have been prepared with Weibull analysis in mind .
Another consideration in using Weibull statistics is the increased number of test specimens that might be needed to characterize an entire strength distribution rather than simply estimate a mean strength value. The optimal number of test specimens depends on many variables, including material and testing costs, the values of the distribution parameters and the desired precision for an intended application. Helpful calculations and tables to make such decisions can be found in the previously cited standards. In the absence of specific requirements, a general rule-of-thumb is that approximately 30 test specimens provide adequate Weibull strength distribution parameters, with more test specimens contributing little towards better uncertainty estimates . More information regarding optimal test specimen numbers, as well as reasons why Weibull distributions are so often observed in material testing practice are discussed by Danzer et al. . They also detail conditions necessary to obtain a Weibull distribution and suggest alternative statistical approaches for analyzing strength data for materials that do not satisfy these conditions, such as materials with unusual or highly mixed flaw distributions. In this sense, Weibull analysis can be regarded as a special, simple case of a broader statistical approach for analyzing strength data . Indeed, Danzer now uses the expression “Weibull material” as one with a single flaw type whose size distribution fits a power law function on the right side tail.
The previous paragraphs highlight some of the assumptions and difficulties in utilizing Weibull statistics in characterizing strength measurements of dental materials. What are the benefits?
It was initially noted that extreme value statistics can be used to predict changes in distributions according to the physical size of the individual test specimens. This is one of the strongest virtues of the Weibull model and what distinguishes it from other distributions. In practical terms, this means that strength values for one test specimen size may be “scaled” to expected strength values for different sized test specimens. This strength scaling permits comparison of strengths of structures with stress gradients such as bend bars or flexed disks. Examples of strength scaling are shown later in this paper. So far we have assumed that all flaws in a body are exposed to the same tensile stresses, but in bodies with stress gradients, some large flaws may be in a low tensile stress or compression region and will not cause fracture. Fracture occurs where there is a critically loaded flaw that has a size, c , and shape factor Y , and a local stress, σ , combination such that the critical fracture toughness, K Ic , is reached in accordance with eq. (1) . 1
1 For simplicity, we ignore rising R -curve behavior whereby fracture resistance changes with crack size and we also ignore environmentally assisted slow crack growth.
Fig. 3 by Johnson and Tucker illustrates how fracture origin sites may be scattered in a 3-point bend bar. For materials with low Weibull moduli (i.e., the flaw sizes are quite variable) fracture can occur from a large portion of the test specimen. On the other hand, the origin sites are concentrated to only the highest stressed regions if the Weibull modulus is large, since the flaw sizes are all similar.
The validity of the Weibull approach can be tested by its ability to scale strengths for a particular specimen size to another size or testing configuration. A review of ceramic flexural strength data tabulated a number of studies wherein ceramic strength scaling by Weibull analysis was successful over a size range as much as four orders of magnitude in volume or area! Strength scaling is done by the concept of an “equivalent” volume or area under stress, discussed in a following section. Whether equivalent volumes or equivalent areas are used depends on whether fracture initiates from volume flaws or surface flaws within the stressed region. 2
2 In rare instances, strength is controlled by edge origin sites, and strength scales with the effective edge lengths.
Using Weibull statistics to calculate corresponding strengths for different test specimen sizes, test specimen shapes and stress configurations is particularly appealing for use in the dental field where sample sizes and testing configurations are frequently different.
Identification and quantization of different flaw populations can explain strength differences and ultimately lead to improved materials. Such approaches can, for example, isolate the effects of grinding media and surface treatments, or determine whether strength might be improved by reducing porosity. Most important of all, determination of the dominant flaw populations, the effects of stress configurations and physical size are all necessary for correlations to clinical components.
3
Experimental examples and discussion
As noted above in Eq. (2) , three parameters were used to define the previously introduced extreme value functions, but only two Weibull parameters are generally reported for strengths. The two-parameter Weibull distribution is obtained by setting σ u = 0, although the three parameter form is not uncommon. When the three parameter Weibull distribution is used, the lower strength bound, σ u , might represent a lower strength limit for a data set. This is analogous to a data set that may have been previously proof-tested or inspected to eliminate flaws over a certain size. The lower bound strength could also correspond to a physical limit to a crack size. It is very risky to assume a finite threshold strength exists without careful screening or nondestructive evaluation. Hence, the two-parameter form is most commonly used for simplicity.
Setting σ u to zero in Eq. (2) and taking the double logarithm of the resulting two-parameter Weibull distribution yields:
P f = 1 − exp − σ σ m
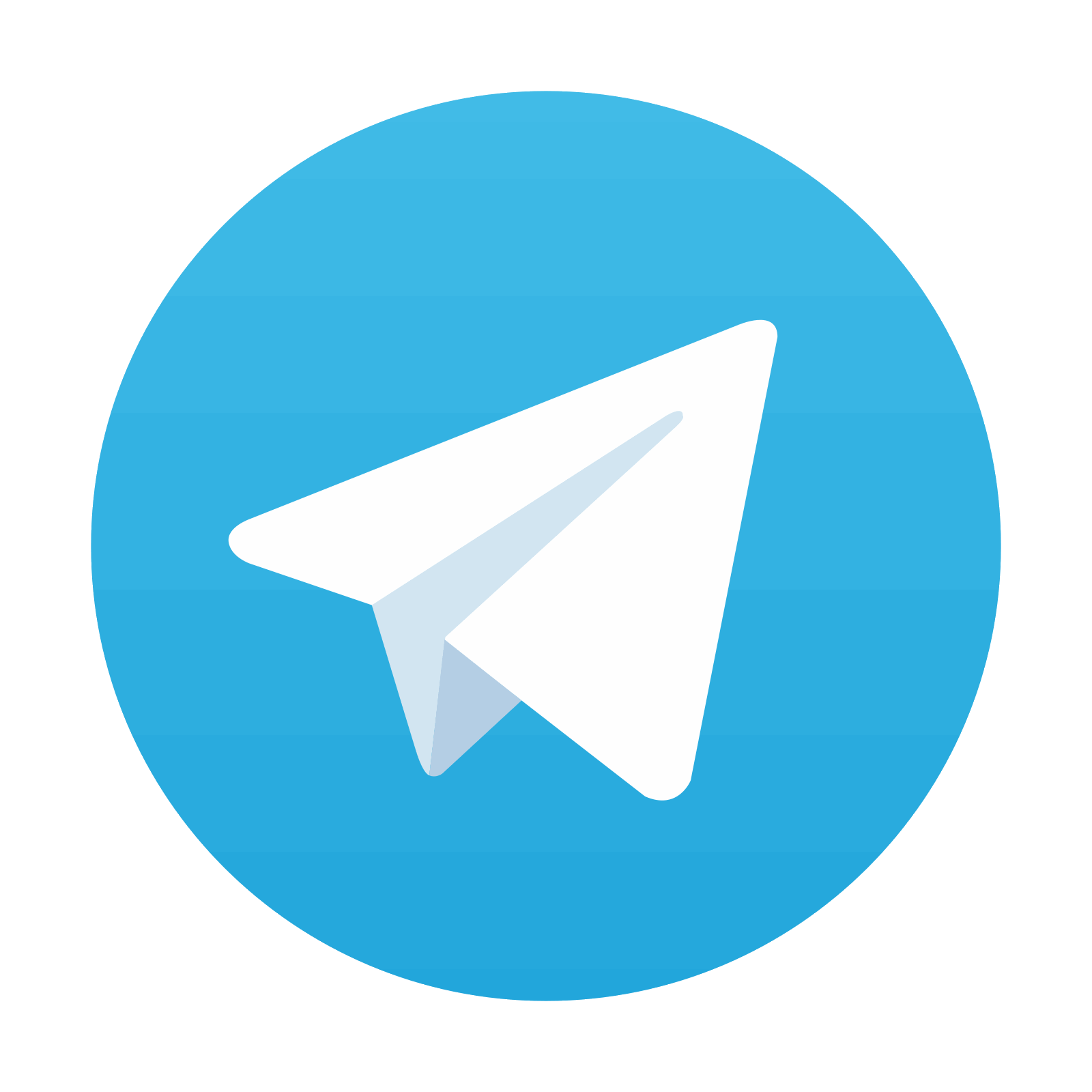
Stay updated, free dental videos. Join our Telegram channel

VIDEdental - Online dental courses
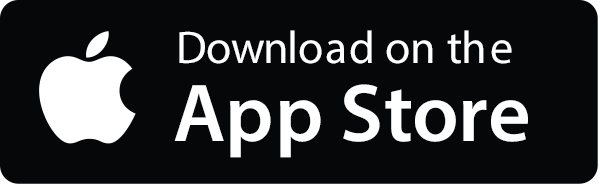
