CHAPTER 14 OVERVIEW OF BIOSTATISTICS
Dental health professionals have a variety of uses for data: for designing a health care program or facility, for evaluating the effectiveness of an oral hygiene education program, for determining the treatment needs of a specific population, and for proper interpretation of the scientific literature, to name just a few. In these instances, data are helpful only to the extent that these sets of data may be summarized and interpreted. Thus evidence-based decisions can be made about the results of research, program evaluation, or needs assessment. These tasks that we ask of data illustrate the two major divisions of statistics: descriptive statistics and inferential statistics. Descriptive statistical techniques enable researchers to numerically describe and summarize a set of data; inferential statistical techniques provide a basis for testing hypotheses and applying statistical results to the group of individuals or objects that form the population of interest.
DESCRIPTIVE STATISTICS
Random Samples
A similar procedure may be applied for selecting a random sample by using a table of random numbers, which can be found in most statistics textbooks. For this example, it would be necessary to use four columns of digits in the tables so that each student, 1 through 5000, would have an equal probability of being selected. Selection would begin by blindly identifying a number on the table that corresponds to a member of the total population (1 through 5000). The selection process continues by taking numbers horizontally or vertically until the desired sample size is reached. Repeated numbers are omitted when encountered during sample selection in both procedures.
Scales of Measurement
In general, data are any information that can be collected. Name, address, job title, social security number, age, gender, income, height, and weight are examples of data. Though not all data are represented by numbers, this discussion is limited to numerical variables. Before one can determine the appropriate methods for summarizing and displaying data, it is necessary to understand the nature of the variable of interest, that is, its scale of measurement. The type of data also plays an important role in deciding which statistical procedures to apply in a test of a hypothesis. The two major scales of measurement are the following classifications: categorical (enumeration) data and continuous data (measurements).
DISPLAYING DATA
Frequency Distribution Tables
The following example illustrates this descriptive display of data. A group of 33 dental students has taken Part I of the National Boards examinations. Their examination scores have been recorded. The dean of the dental school wishes to summarize these scores at the next school faculty meeting. Here are a few of the ways that the information could be presented.
First, an ungrouped frequency distribution table of the National Board scores is presented in Table 14-1. The variable of interest is the examination score, which is shown in the first column of the table. The examination scores for the group are listed in descending order. The next column of the table contains the frequency with which each score occurs in the data set. Next, the frequency of occurrence is expressed as a relative frequency, that is, as a percent of the total number of scores represented in the table. For example, three students scored 77 on the examination. This represents 9.1% of the group of 33 students.
Second, the data can be displayed as a cumulative frequency distribution. Table 14-1 shows the cumulative frequency and cumulative percent for the National Board scores. These descriptive measures express the frequency of occurrence of scores up to and including any given value in the data set. For example, 25 students (75.8% of the group) scored 80 or below on this examination. Also, the score that defines the 97th percentile is 88.
A grouped frequency distribution for the National Board scores is illustrated in Table 14-2. Note that although the data are condensed in a useful fashion, some information is lost. The frequency of occurrence of an individual data point cannot be obtained from a grouped frequency distribution. For example, seven students scored between 74 and 77, but the number of students who scored 75 is not shown here.
Table 14-2 Grouped Frequency Distribution of National Board Scores
Scores | Number of Students | % |
---|---|---|
56-61 | 2 | 6 |
62-65 | 3 | 9 |
66-69 | 5 | 15 |
70-73 | 4 | 12 |
74-77 | 7 | 21 |
78-81 | 7 | 21 |
82-85 | 3 | 9 |
86-89 | 2 | 6 |
Graphs
A bar graph is a two-dimensional pictorial display of data that is measured on a categorical scale, either nominal or ordinal. Each category is represented by a separate bar, and the height of the bar reflects the number or percent of observations belonging to that category. In a bar chart, the bars do not touch each other, and the order of the bars (categories) should be determined by what makes the most sense for the variable that is pictured in the chart. Figure 14-1 is an example of a graph of the distribution of a categorical variable measured on the nominal scale. Each bar displays the percent of the study’s subjects who belong to each category of marital status. Because the scale is nominal, the bars can be scrambled with no loss of meaning or understanding.
A histogram is also a graphic representation formed directly from a frequency distribution table, but a histogram is used to display a continuous measurement variable. A histogram is a display in which the horizontal (abscissa) axis is a continuous number line that represents the measurement scale of the variable of interest. These values on the x axis are grouped into equal intervals, and the number of observations in each interval are counted and displayed on the vertical (ordinate) axis. Graphically, a histogram is similar to a bar graph because the frequency is also represented by the height of a bar over the interval in question. However, the bars in a histogram must touch one another because of the continuous nature of the scale of measurement. Figure 14-2 shows the histogram for the continuous variable age (in years).
Tables
In addition to graphs, data are often summarized in tables. When material is presented in tabular form, the table should be able to stand alone; that is, correctly presented material in tabular form should be understandable even if the written discussion of the data is not read. A major concern in the presentation of both figures and tables is readability (Box 14-1). Tables and figures must be clearly understood and clearly labeled so that the reader is aided by the information rather than confused. The student is directed to standard biostatistics texts for a formal discussion on summarizing data in graphic and tabular form. Also, scientific writing style manuals generally contain discussions on the formal display of tables and graphs. It is also helpful to scan the existing literature for good examples of both graphs and tables.
BOX 14-1 Suggestions for the Display of Data in Graphic or Tabular Form
NUMERICAL SUMMARY OF DATA
Measures of Central Tendency
The mode of a data set is that value that occurs with the greatest frequency. When two or more values have equally large frequencies, it is possible for a distribution to have more than one mode. For example, the distribution of scores in Table 14-1 has two modes, 77 and 81. Both occur with the equally high frequency of three. The primary value of the mode lies in its ease of computation and in its convenience as a quick indicator of the central value in a distribution. Beyond this, its statistical uses are extremely limited.
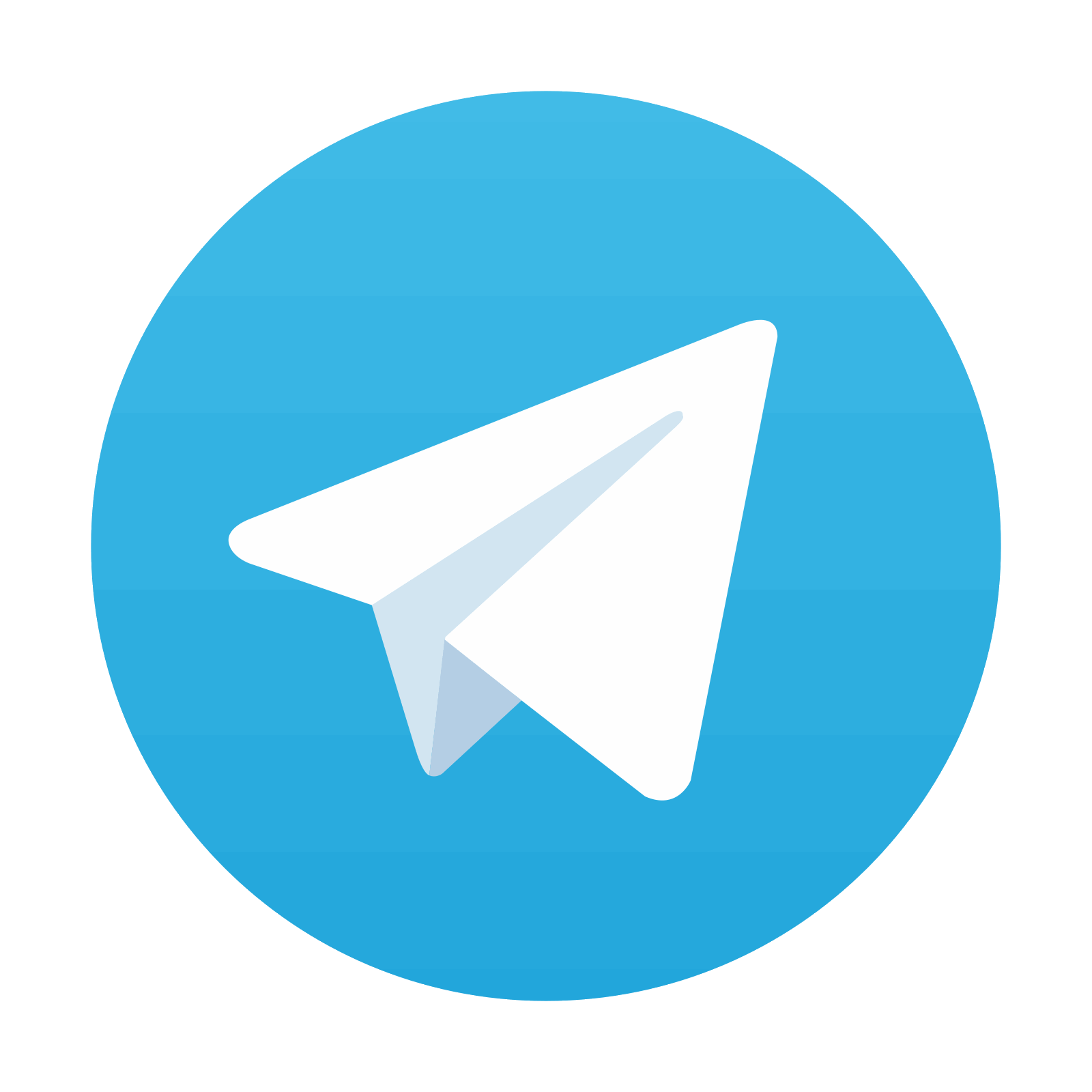
Stay updated, free dental videos. Join our Telegram channel

VIDEdental - Online dental courses
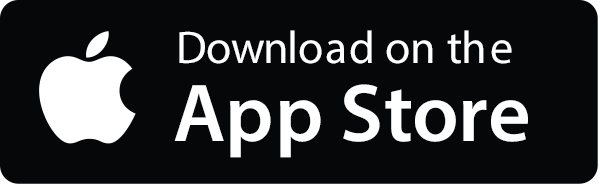
